Machine Learning for House Price Prediction in London
My goal with my dissertation was to use it as a way to expand my knowledge horizons, by combining learning a new skill [machine learning] with a real-world topics that sparks my interest [real estate].
This project allowed me to dive into the booming topic of artificial intelligence, an area I was completely unfamiliar with. In addition, it provided me with the opportunity to use the knowledge I acquired from my degree in the built environment to critically analyse the problem from a different perspective than most people with backgrounds in economics or computing. Throughout the research process, I developed an understanding of London real estate to a deeper extent, beyond just the buildings as individual physical assets themselves, but how it behaves holistically as a market.
FULL PROJECT AVAILABLE BELOW
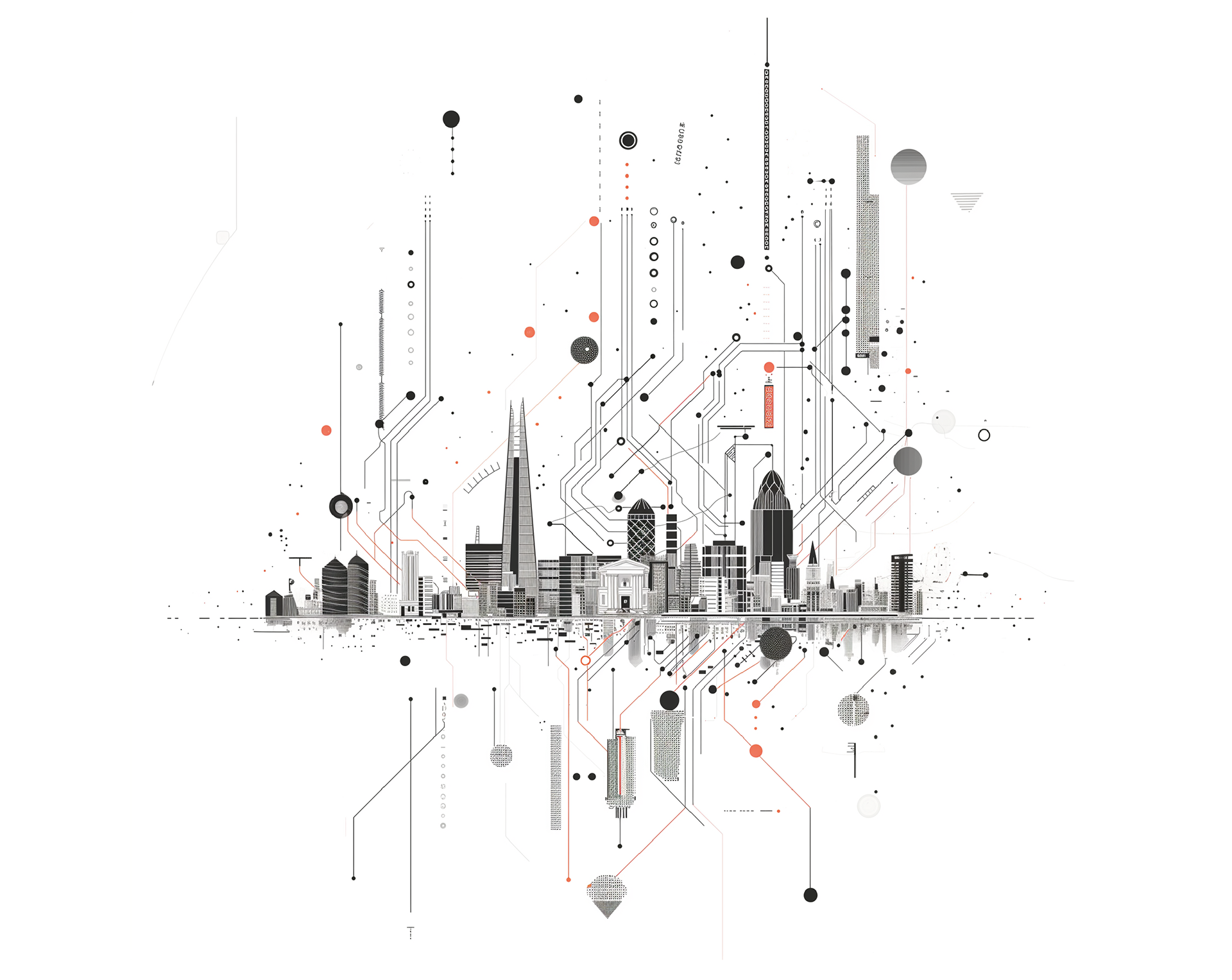
ABSTRACT
Predicting real estate prices in dynamic urban environments like London poses a significant challenge. A multitude of factors influence property value, including human, temporal, spatial and economic dynamics. The present study contributes to advancing the art and science of real estate forecasting, using a results-based, multi-phased approach. By analysing current practice and industry initiatives, the study seeks to develop a transparent framework which can be built upon using only publicly available data sources and tools. To model property location, an innovative approach of distance feature engineering is proposed. The process of model testing and iteration reveals key predictors affecting property prices, as well as challenges regarding data quality and model robustness. Findings reveal the significance of size and location-related features, as well as the limitations inherently present in predicting real estate prices, highlighting the complex relationship of known and unknown variables. While machine learning can provide valuable insights to stakeholders, discrepancies between predictions and real-life outcomes require cautious interpretation of the results, in order to mitigate against risk and avoid adverse implications on involved parties.
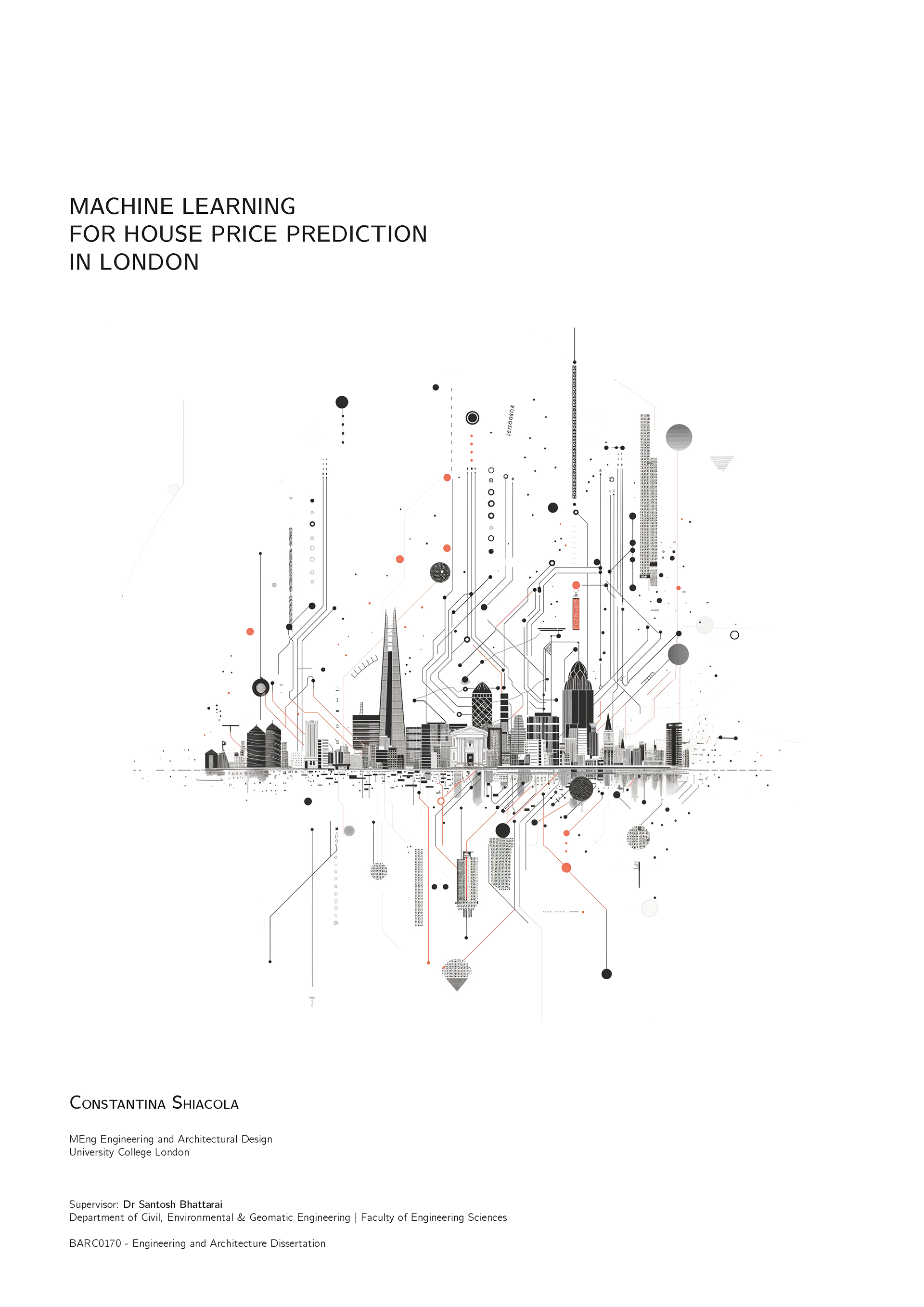
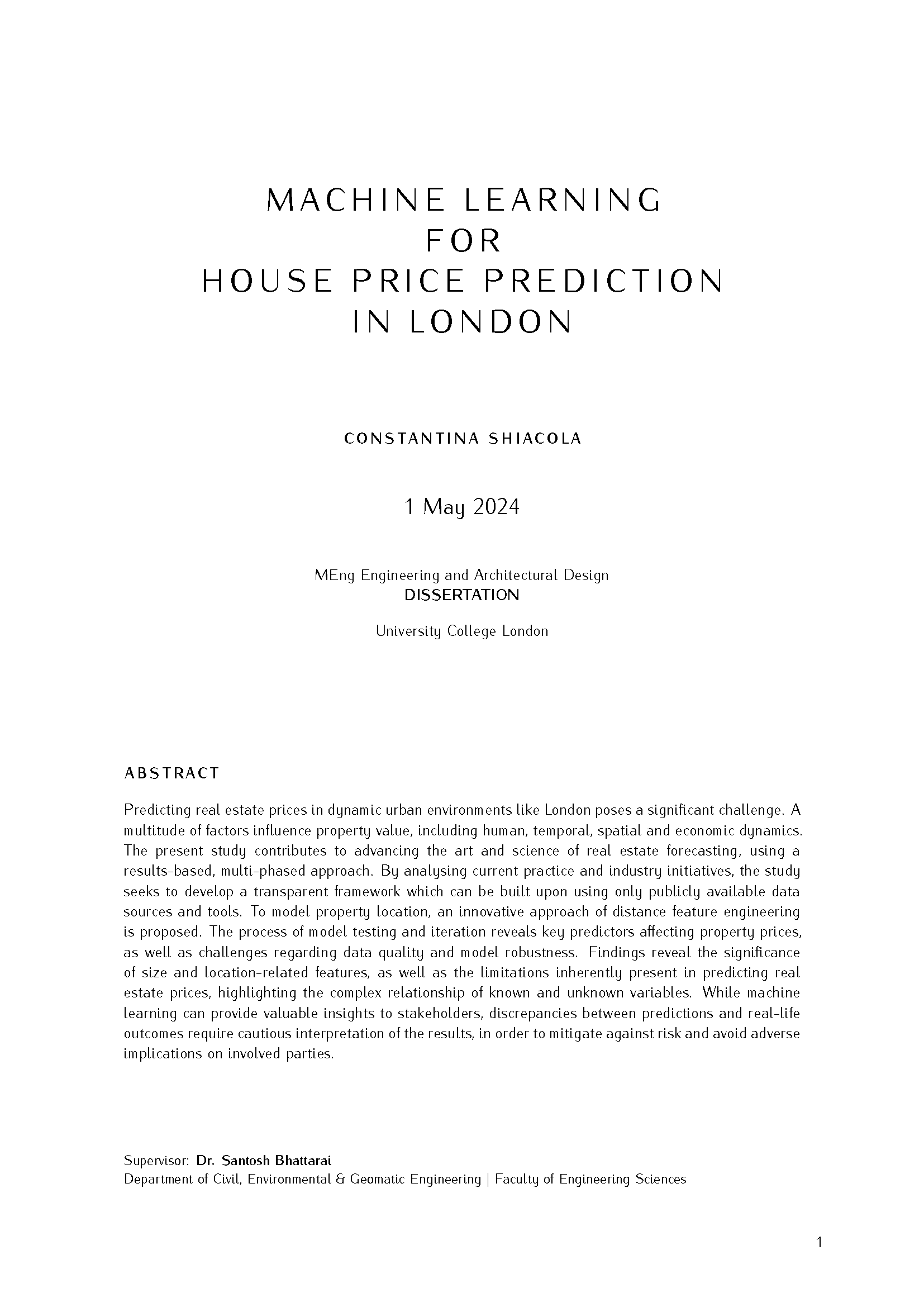
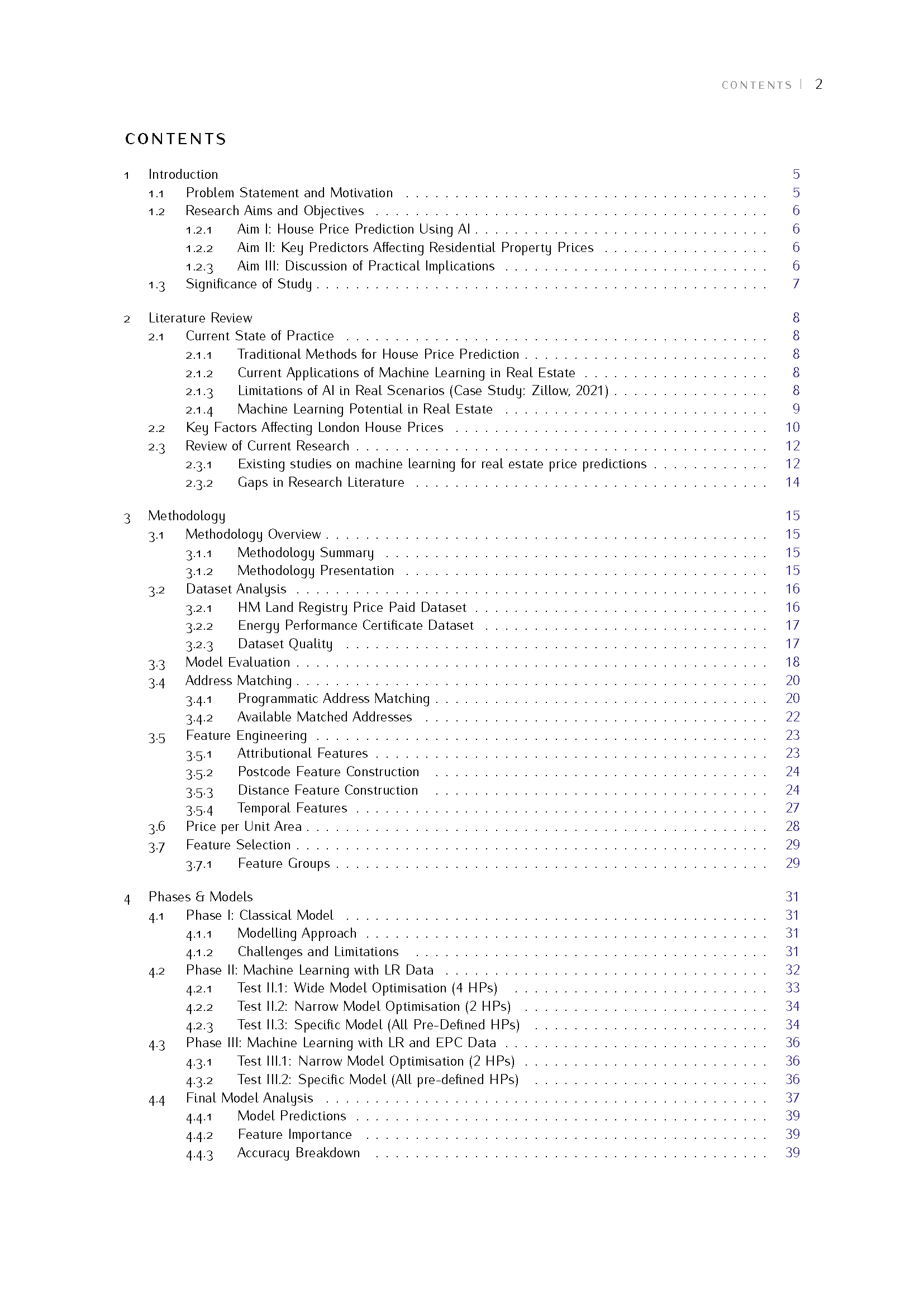
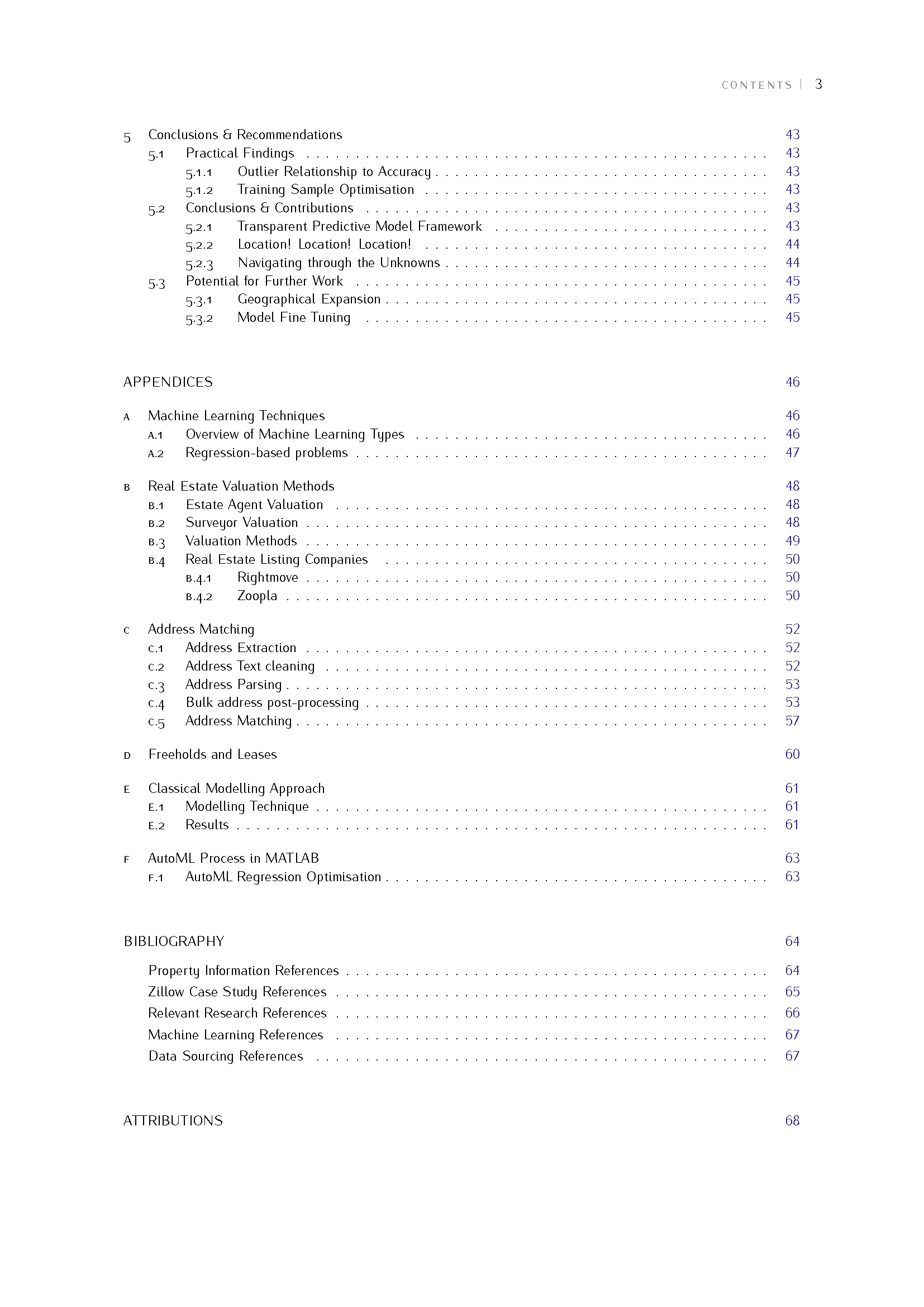
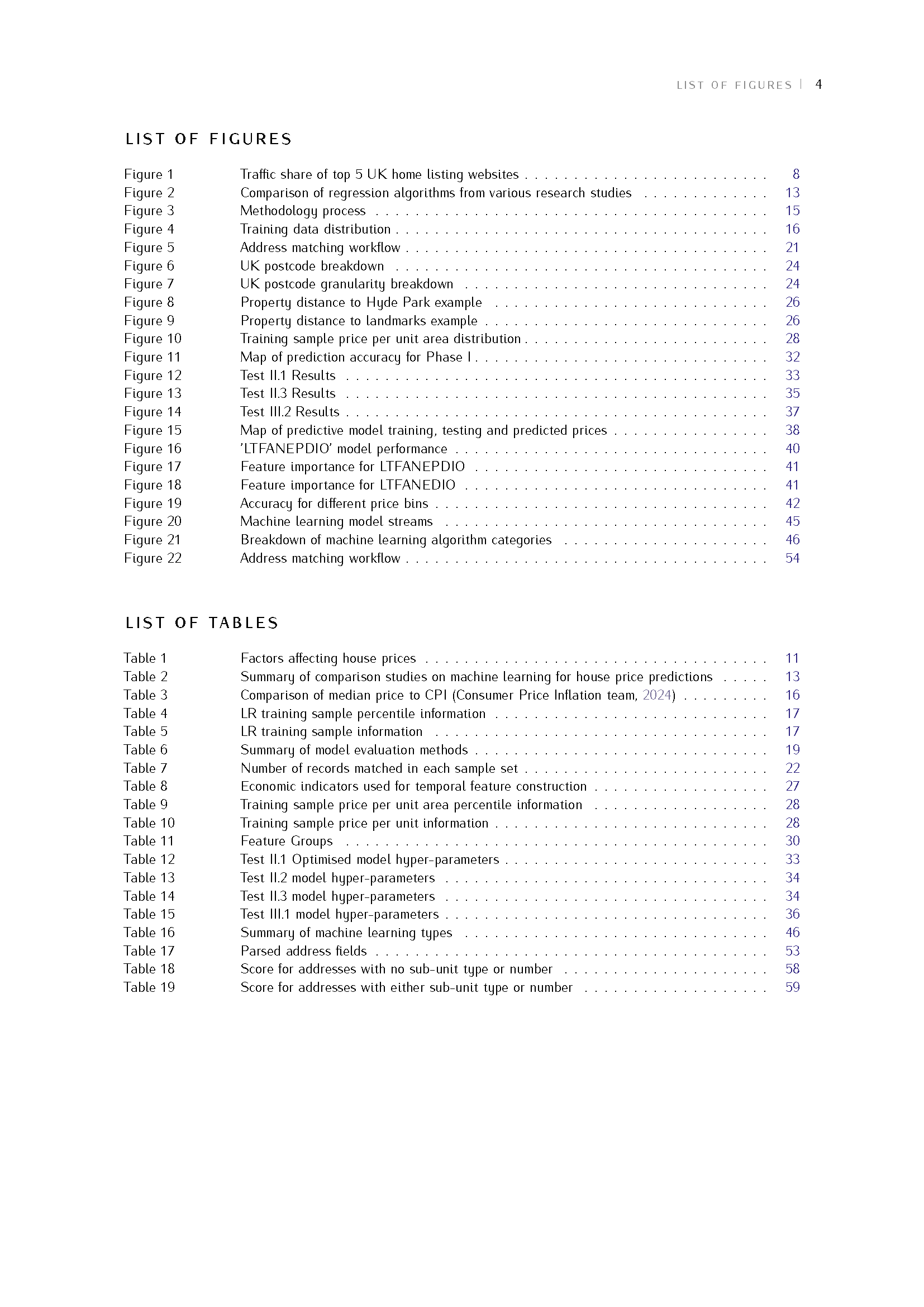
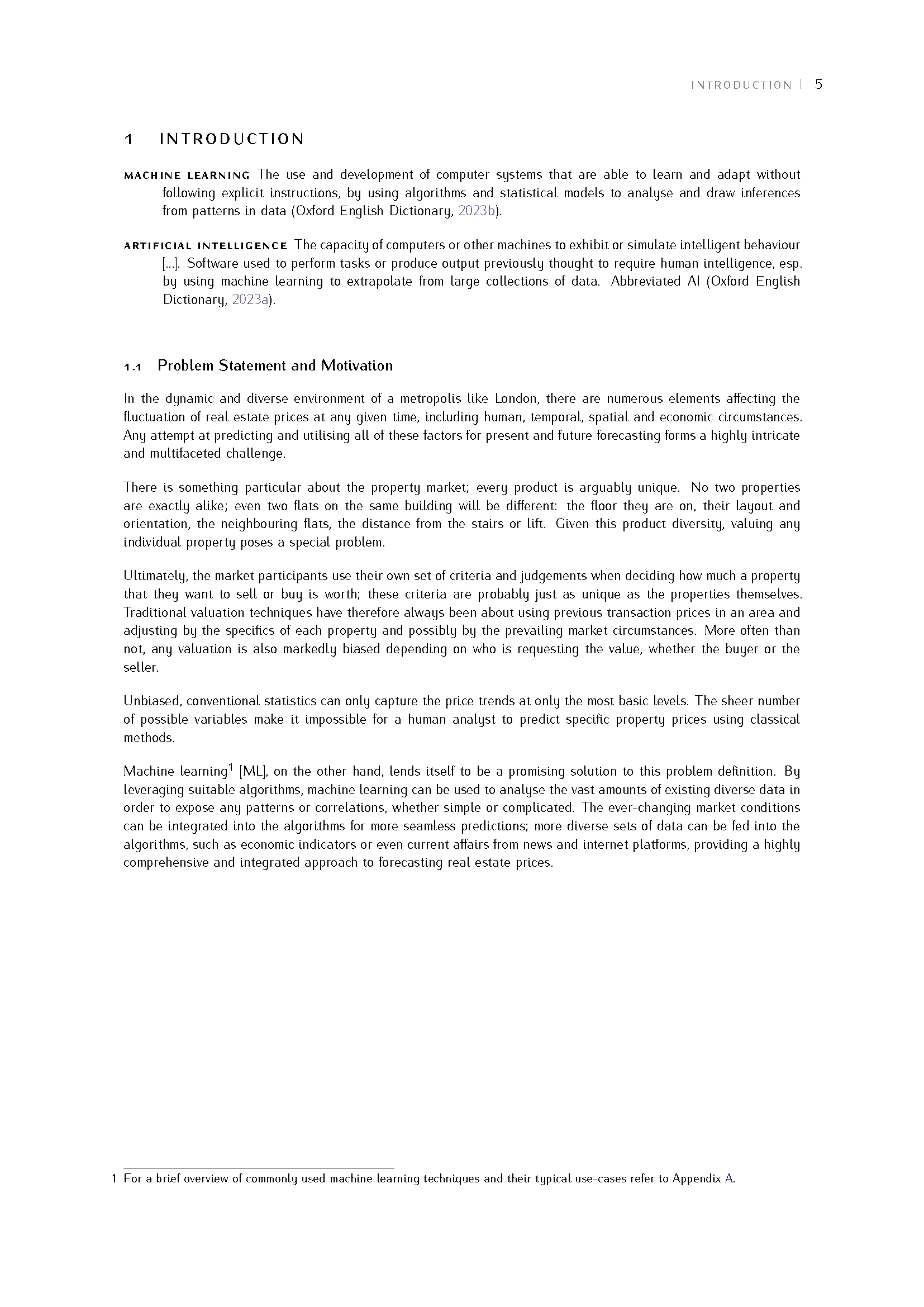
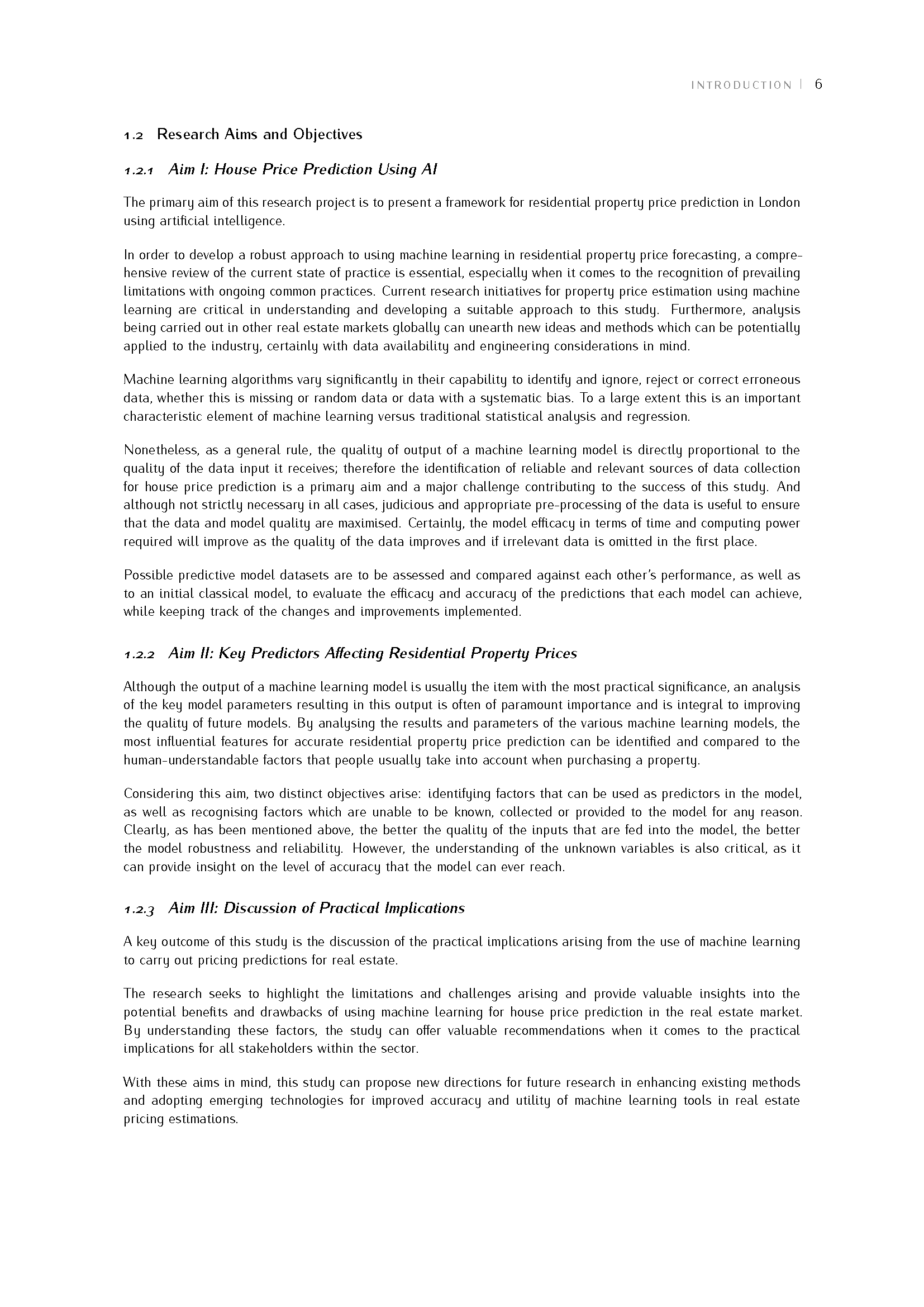
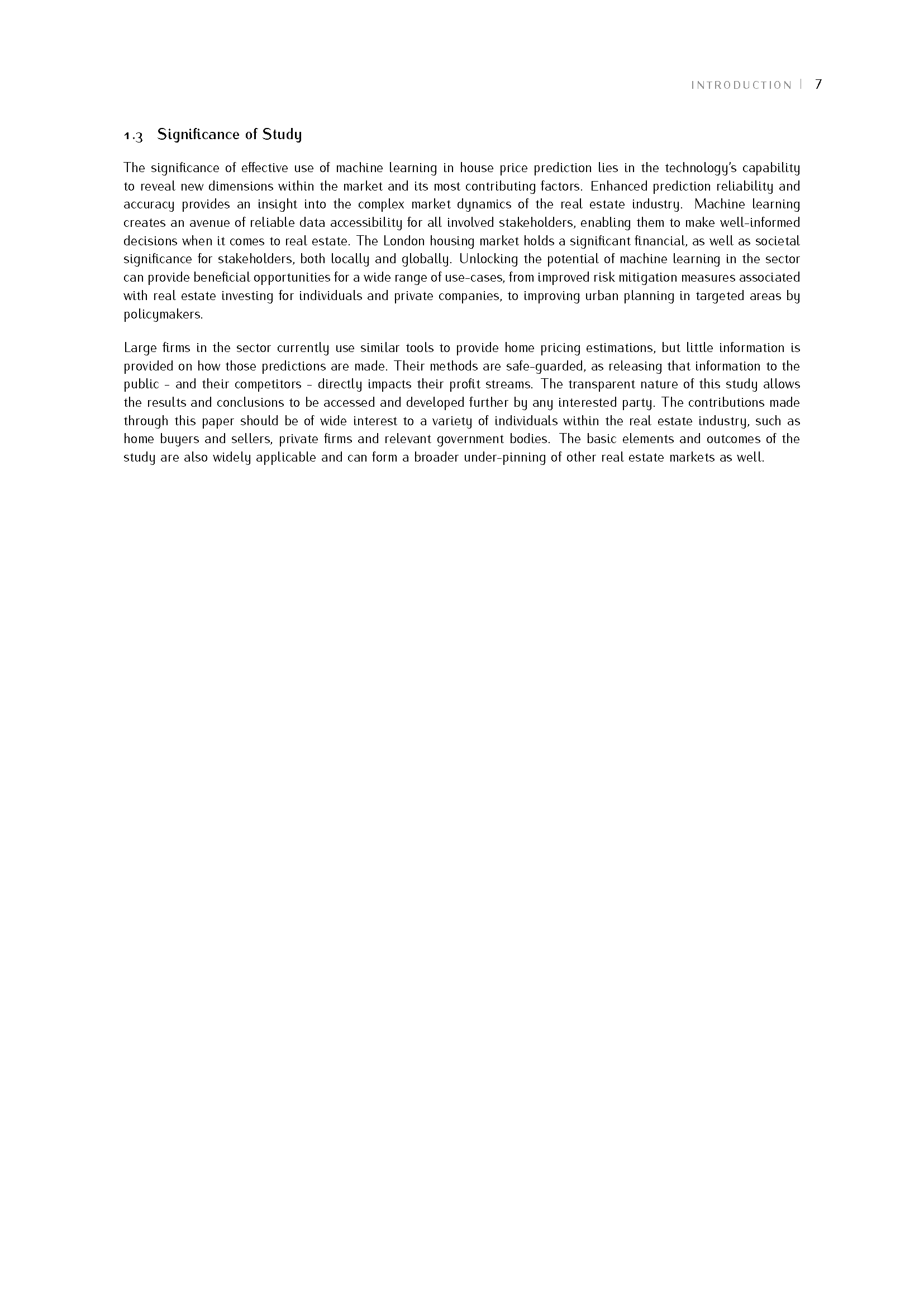
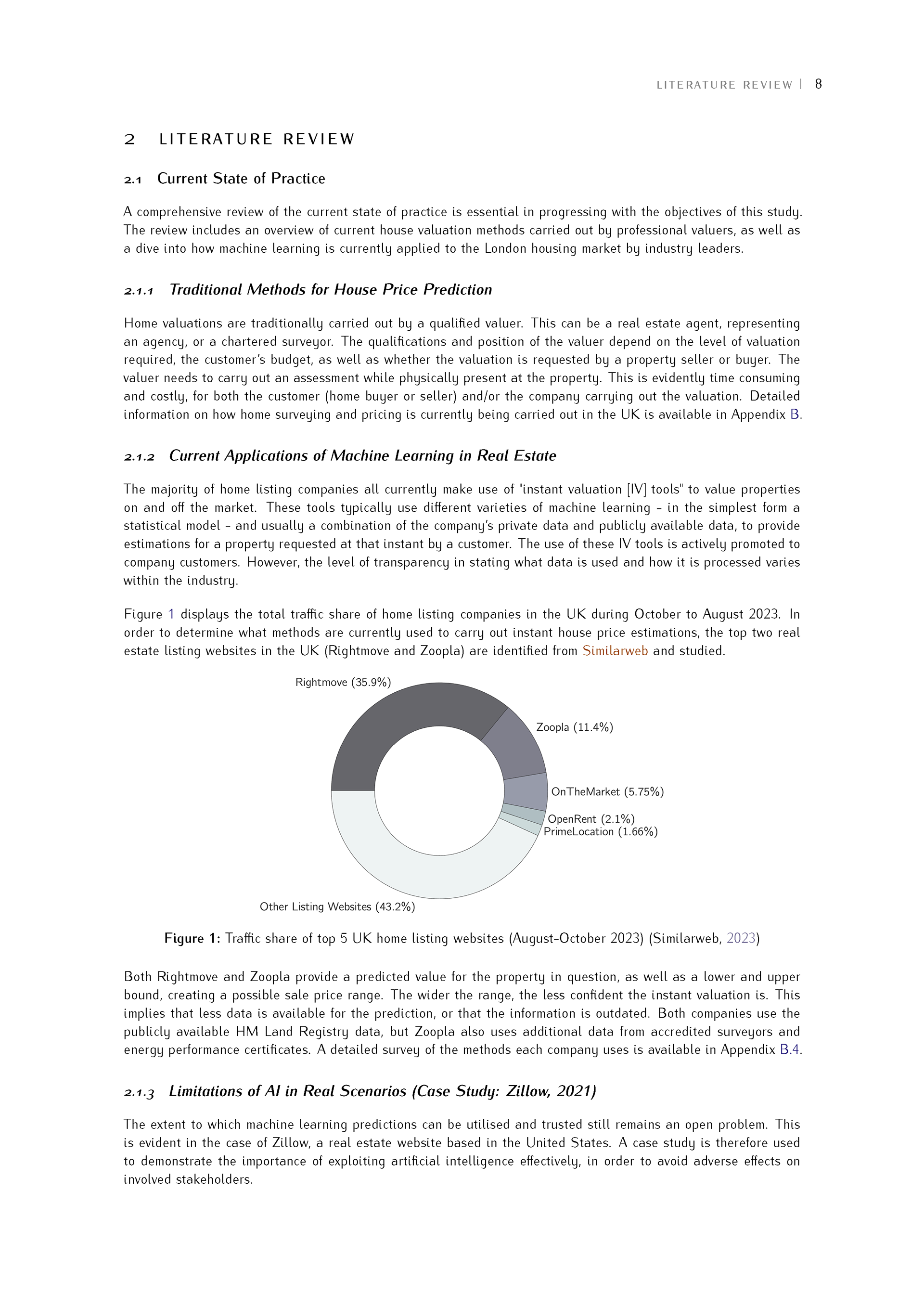
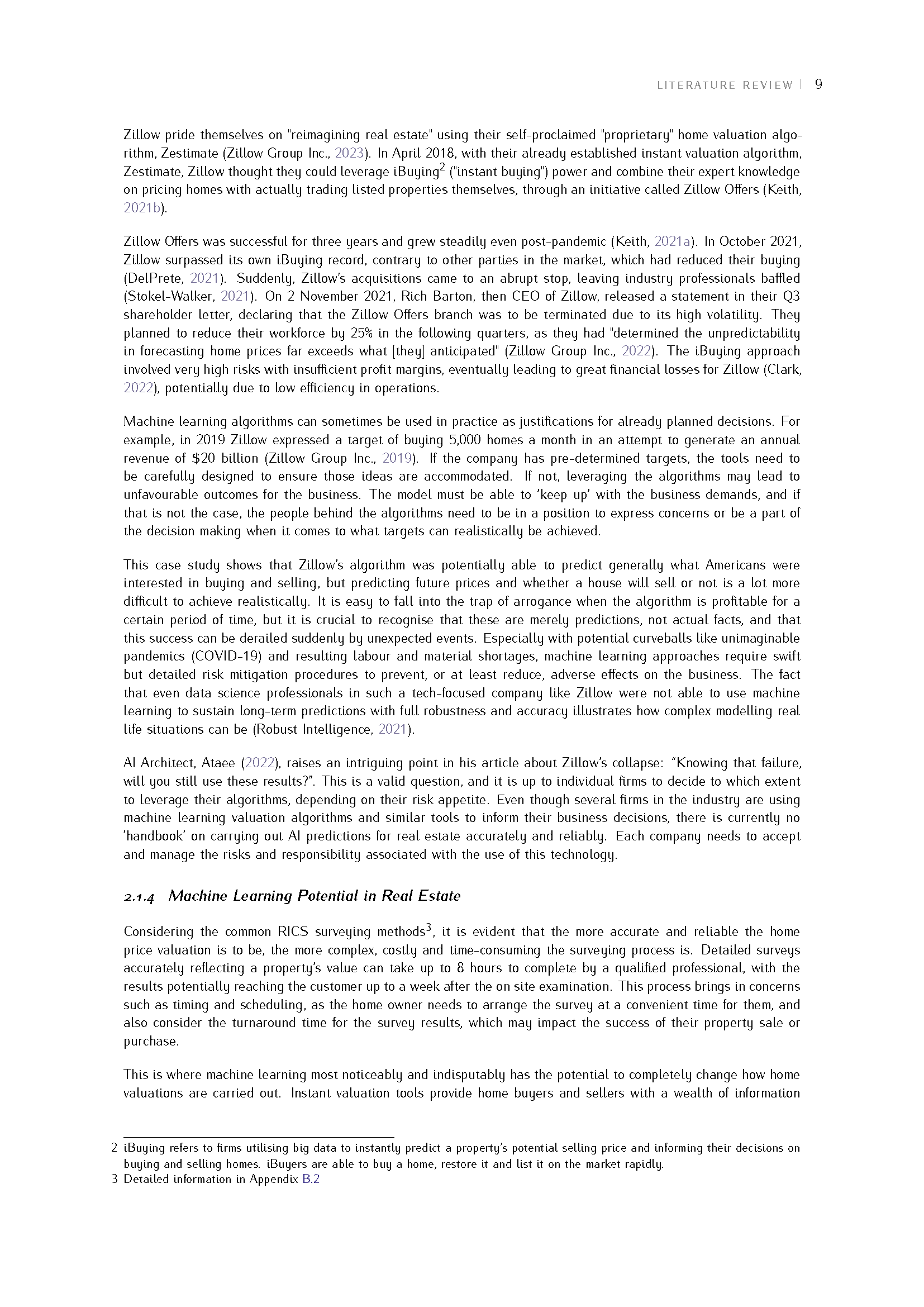
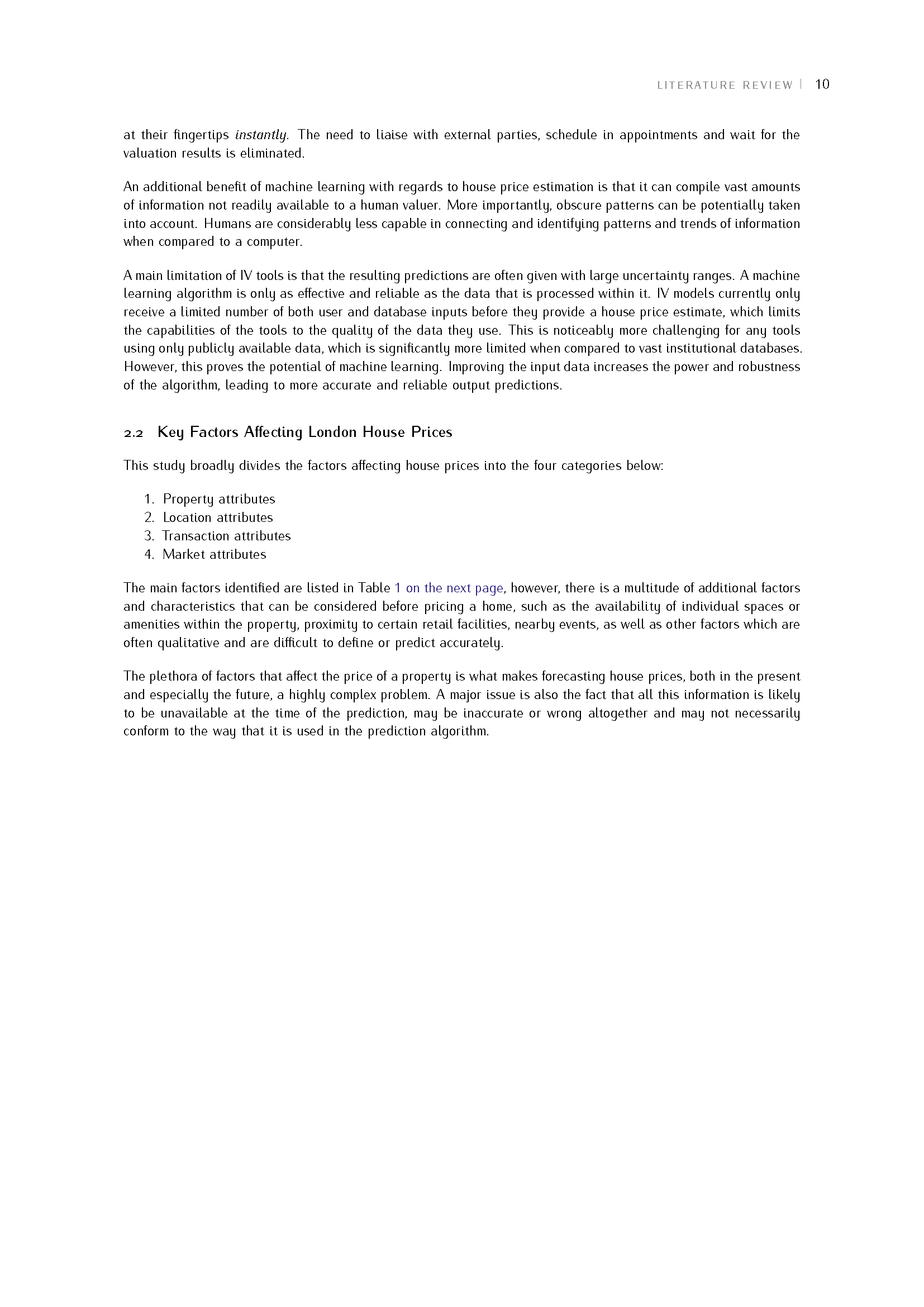
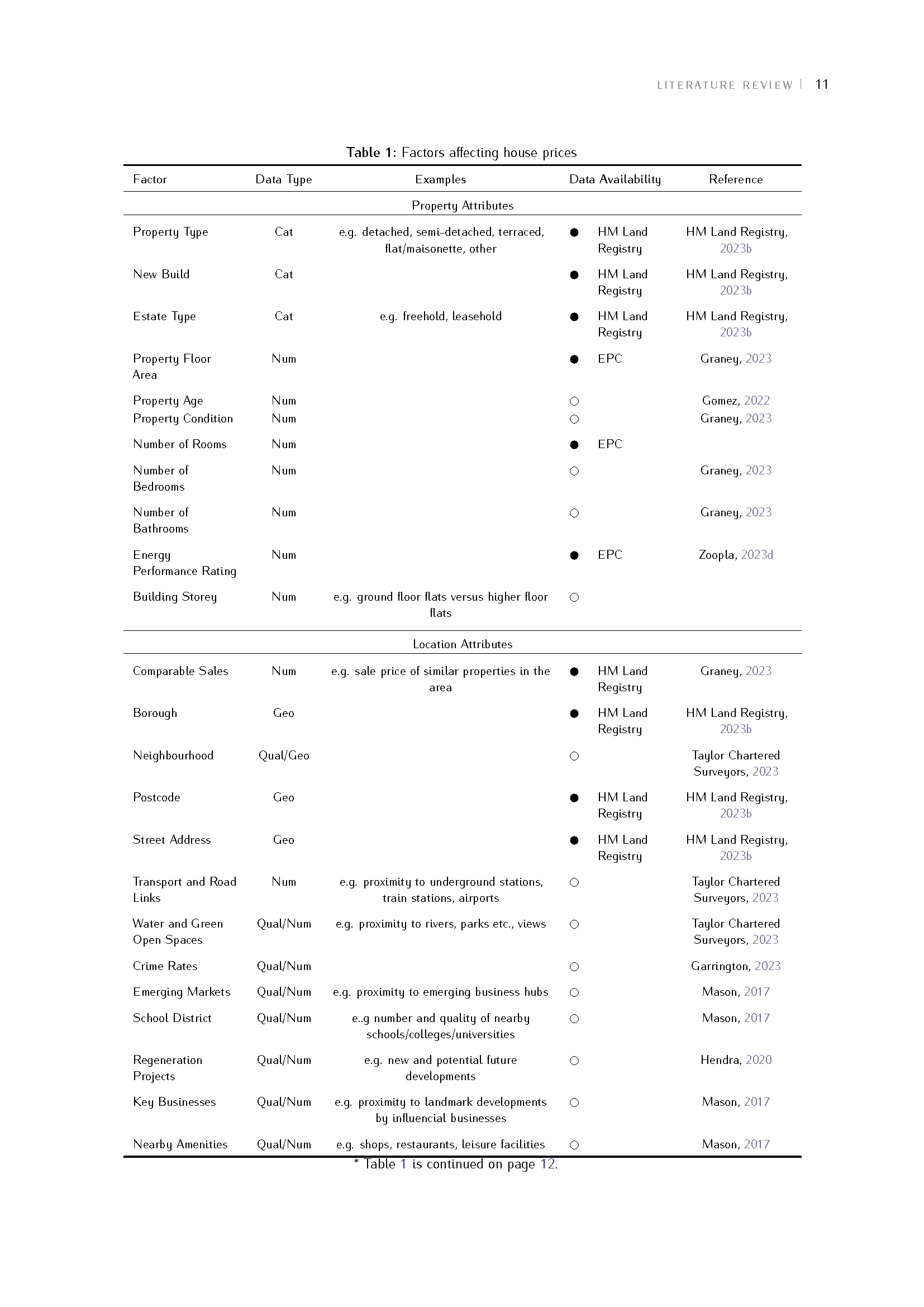
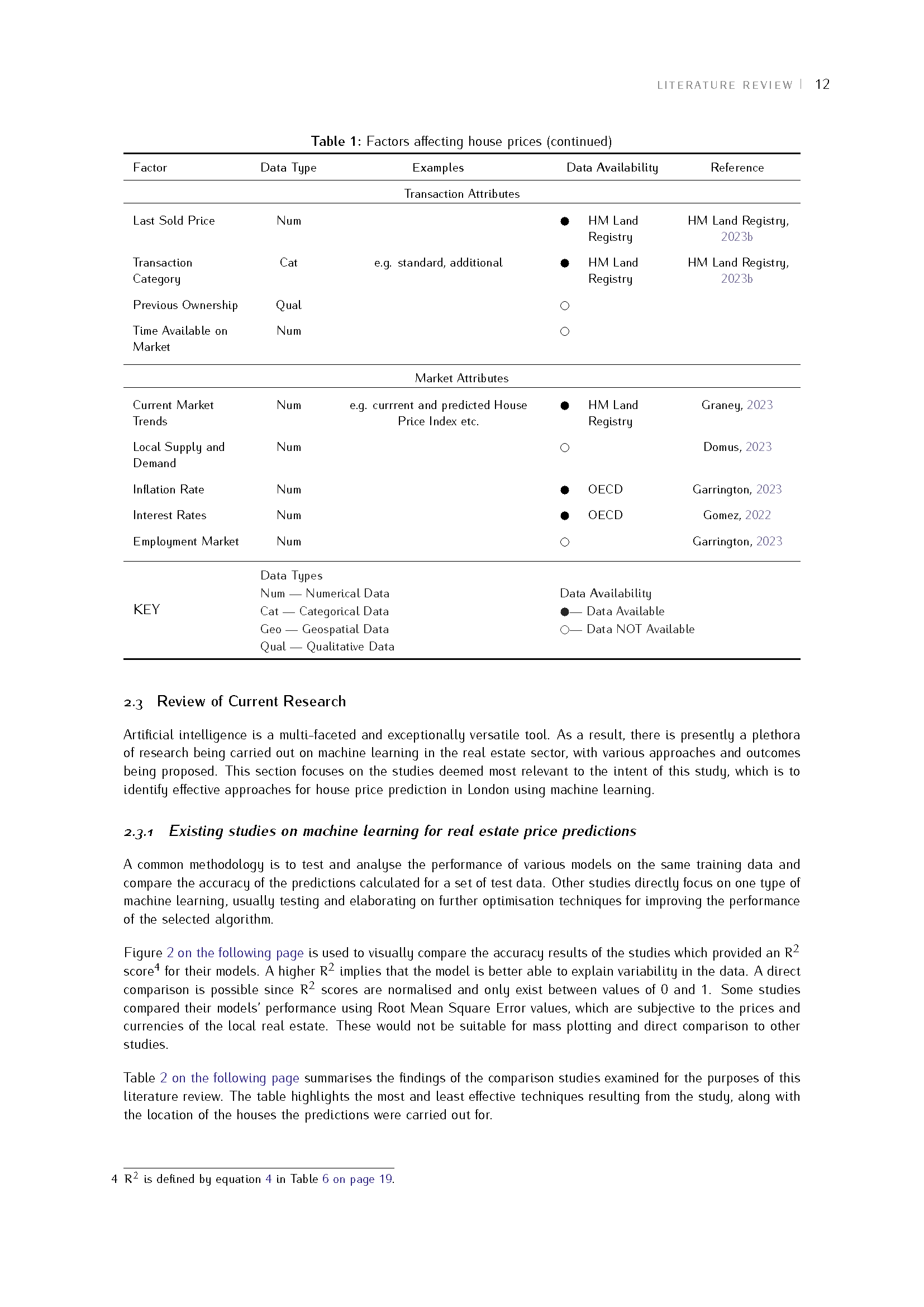
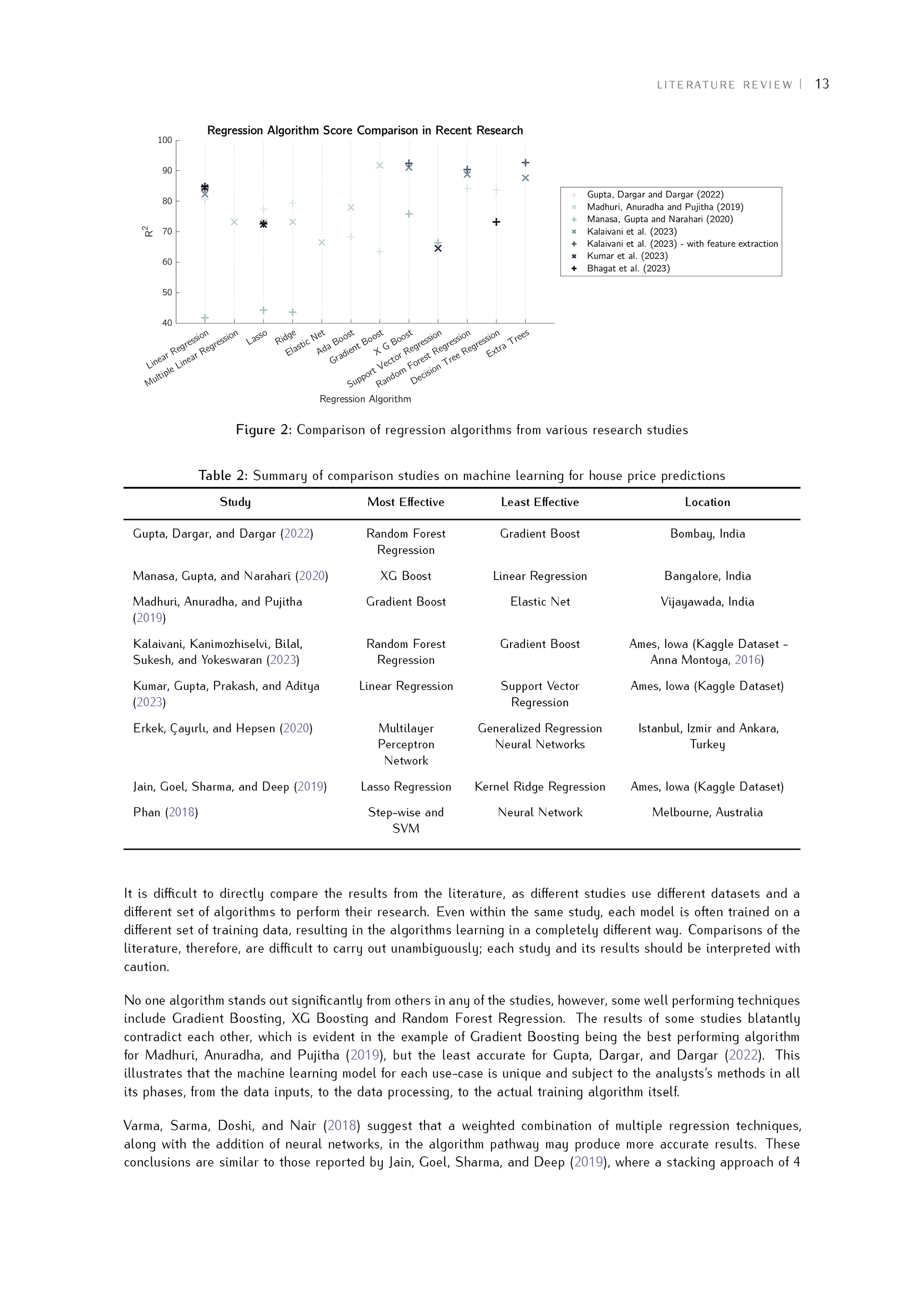
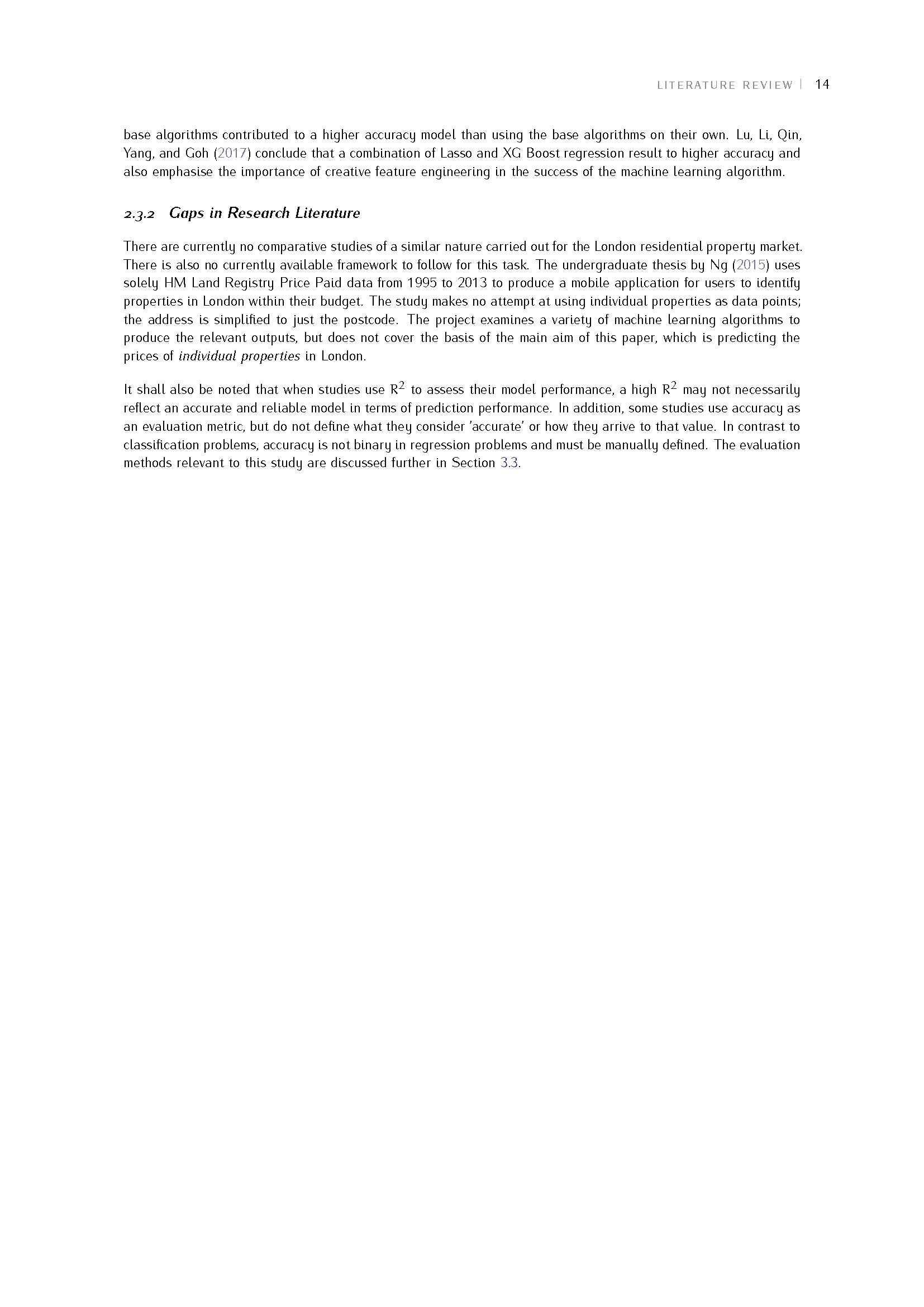
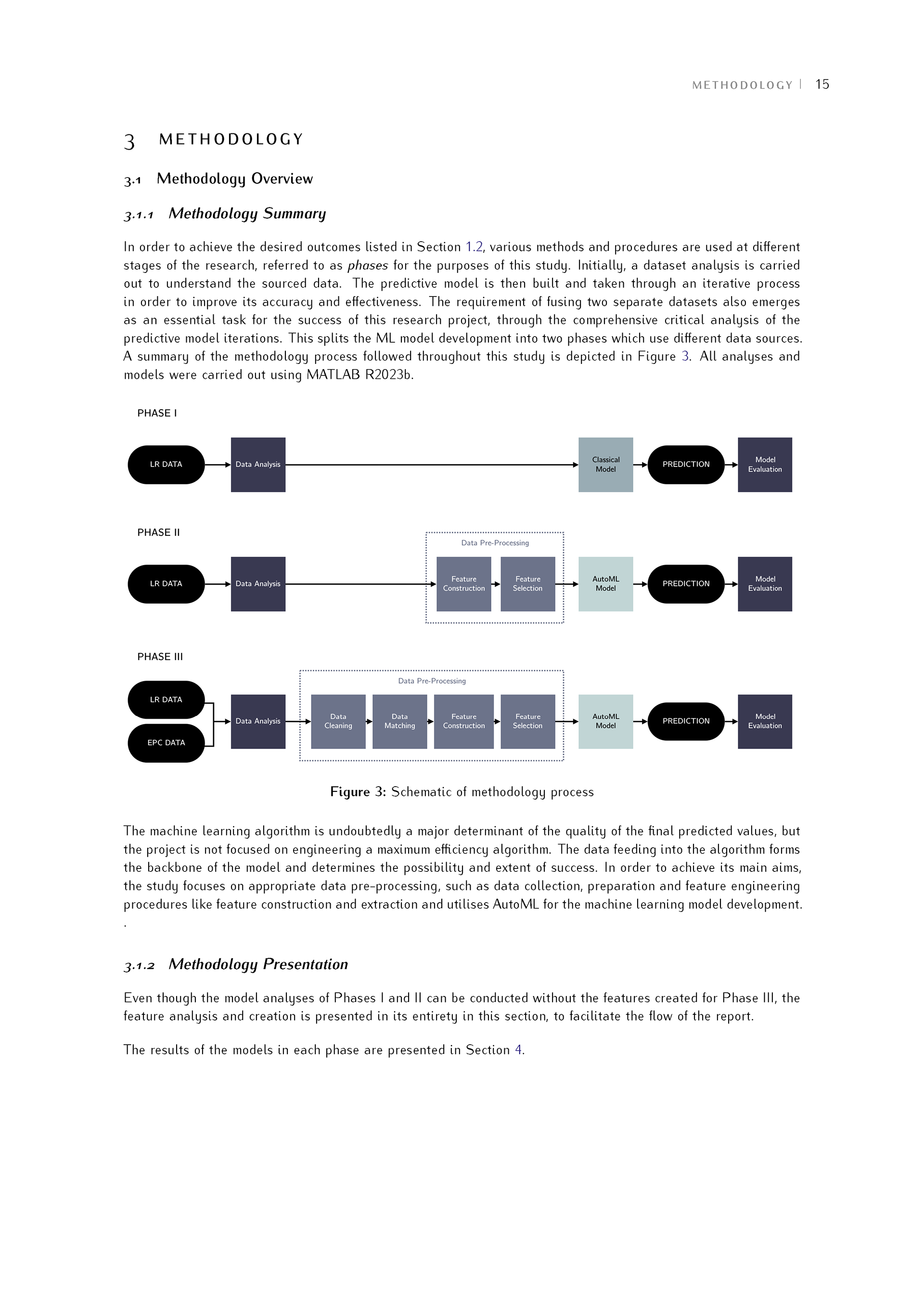
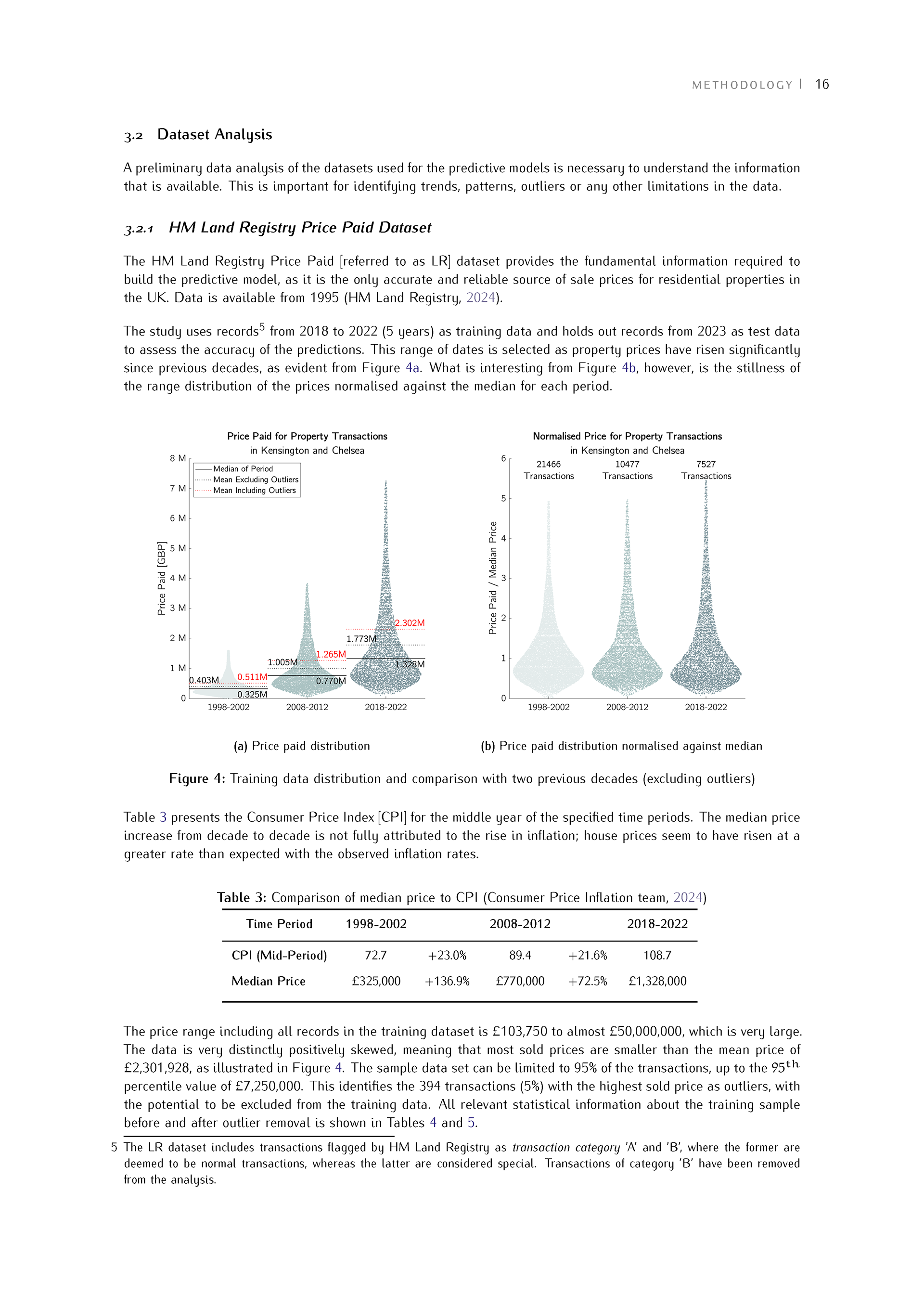
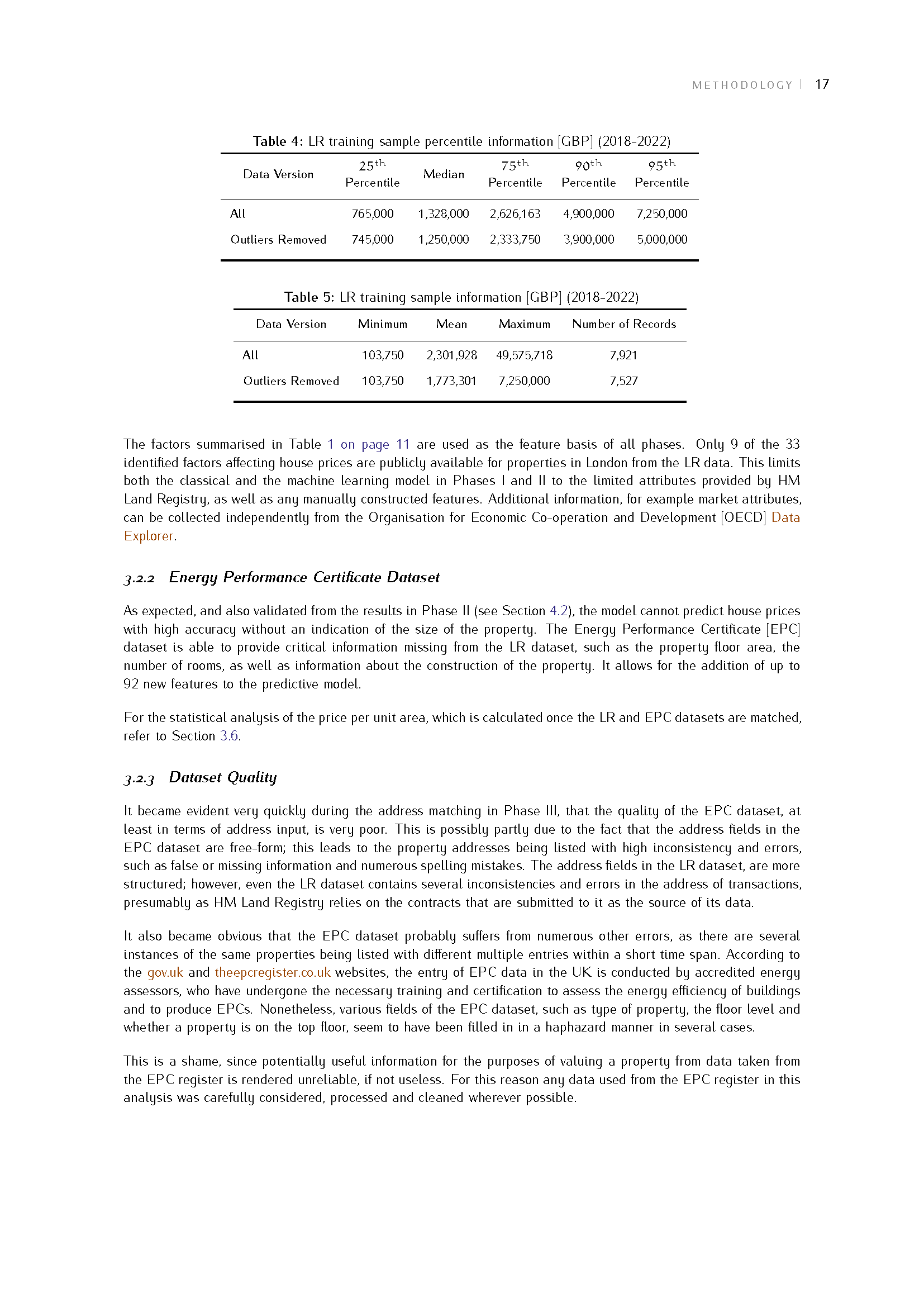
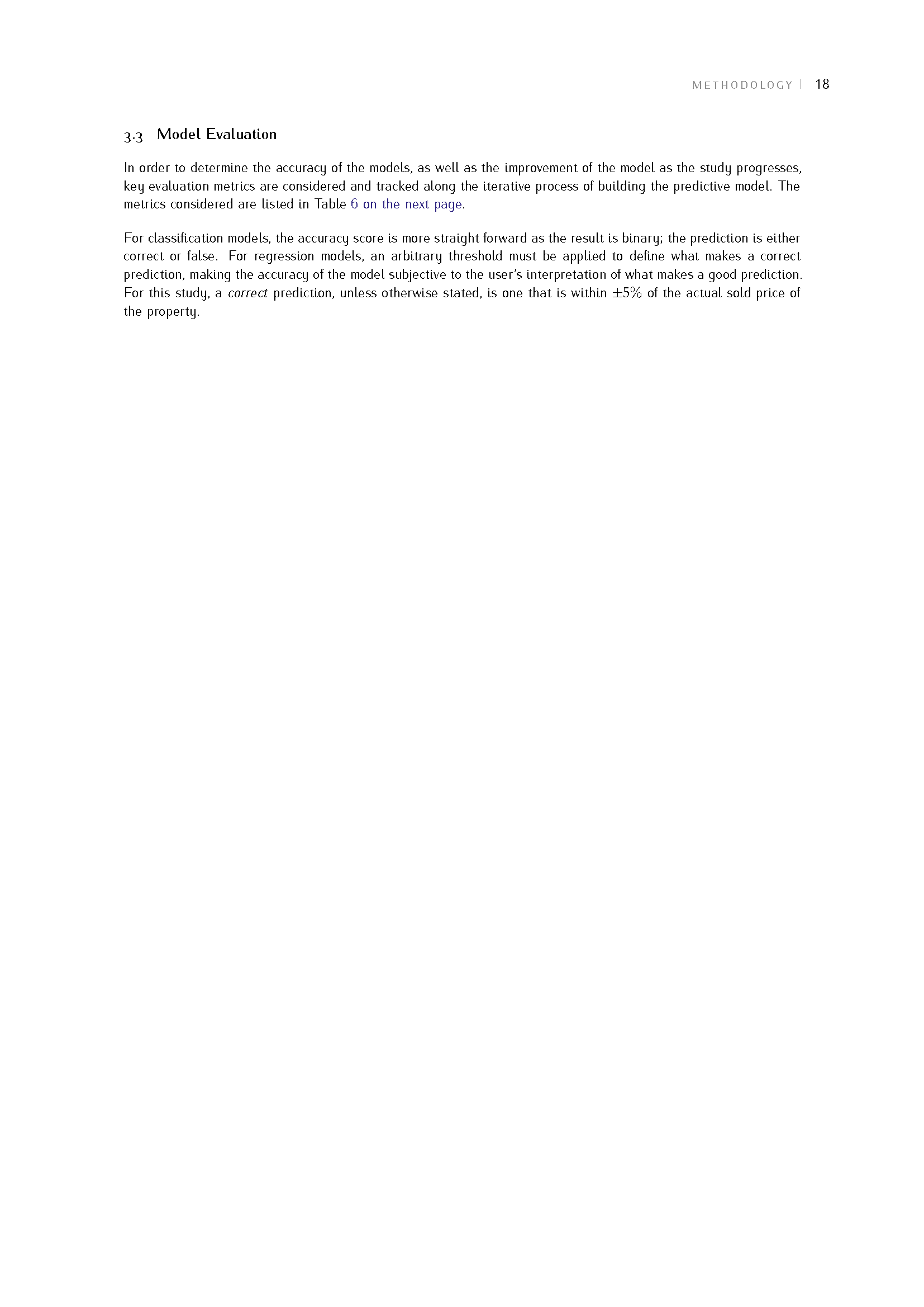
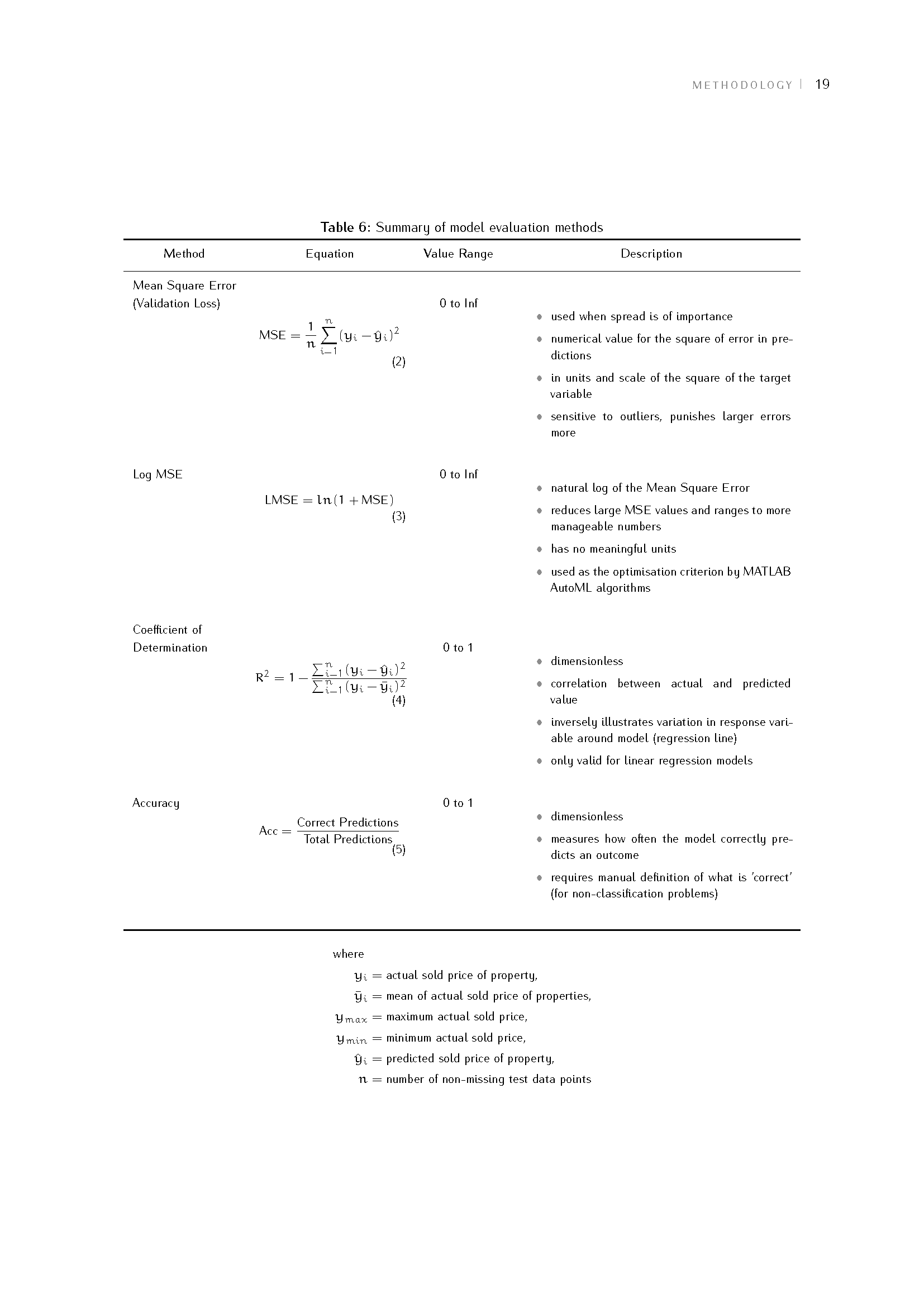
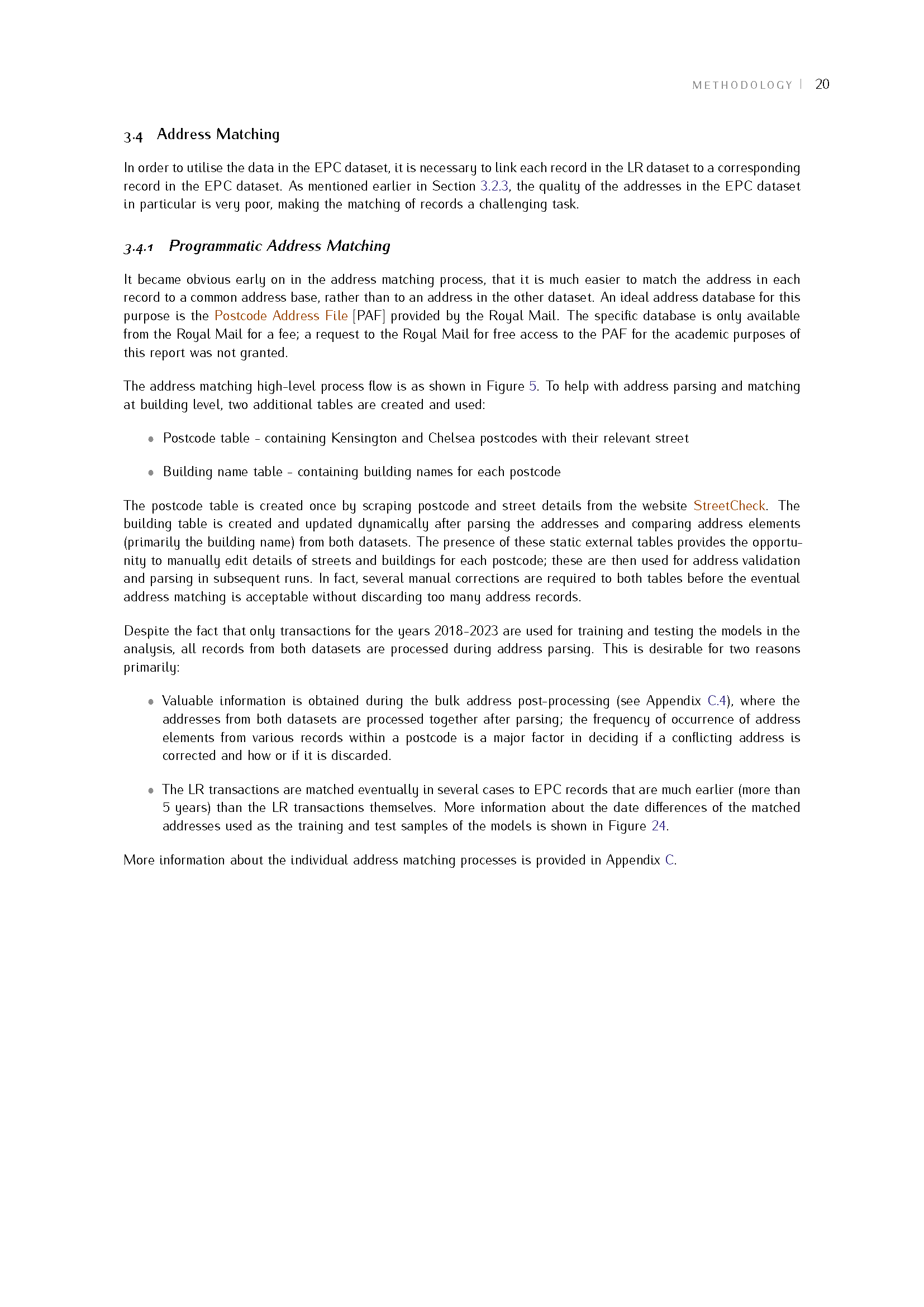
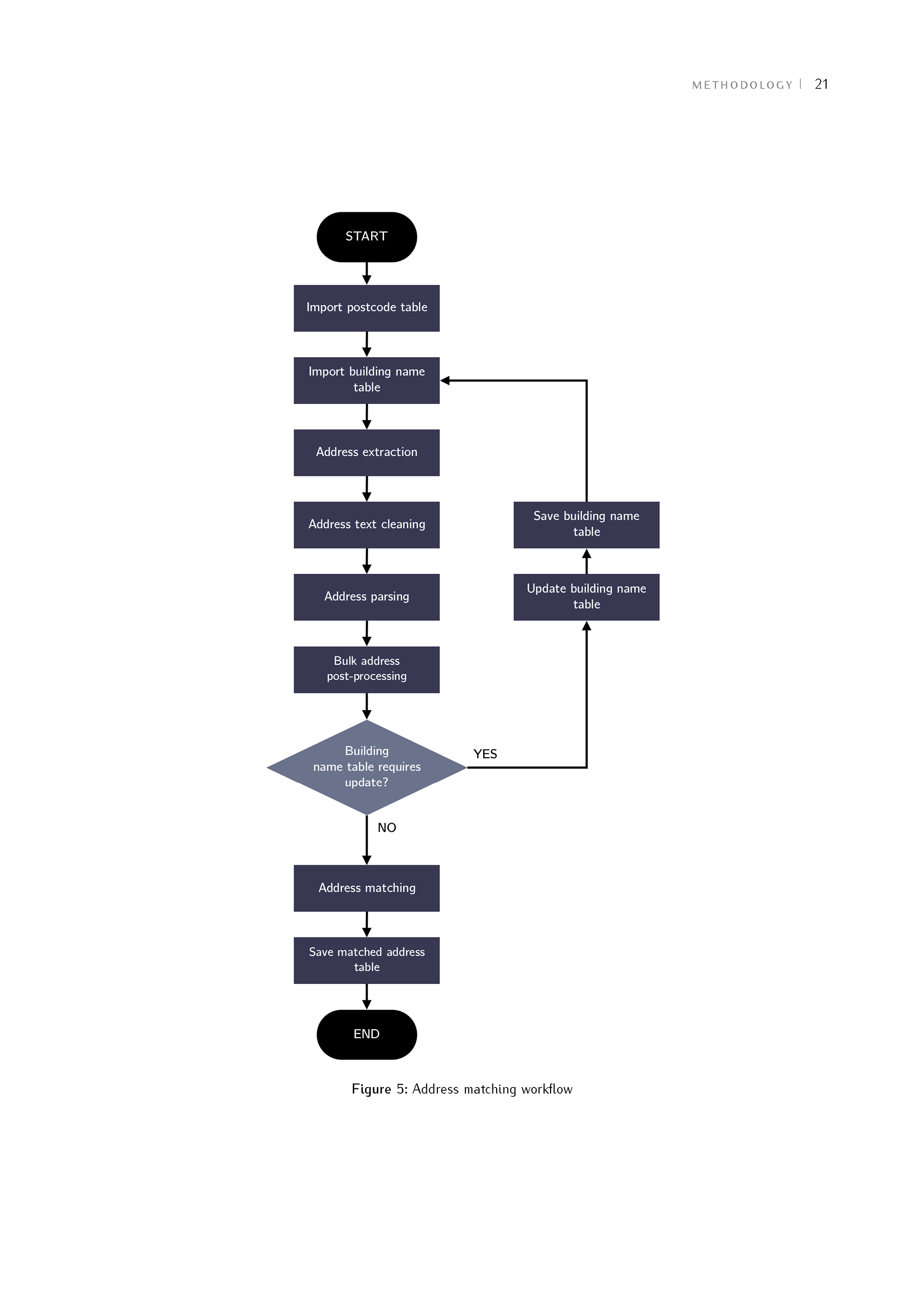
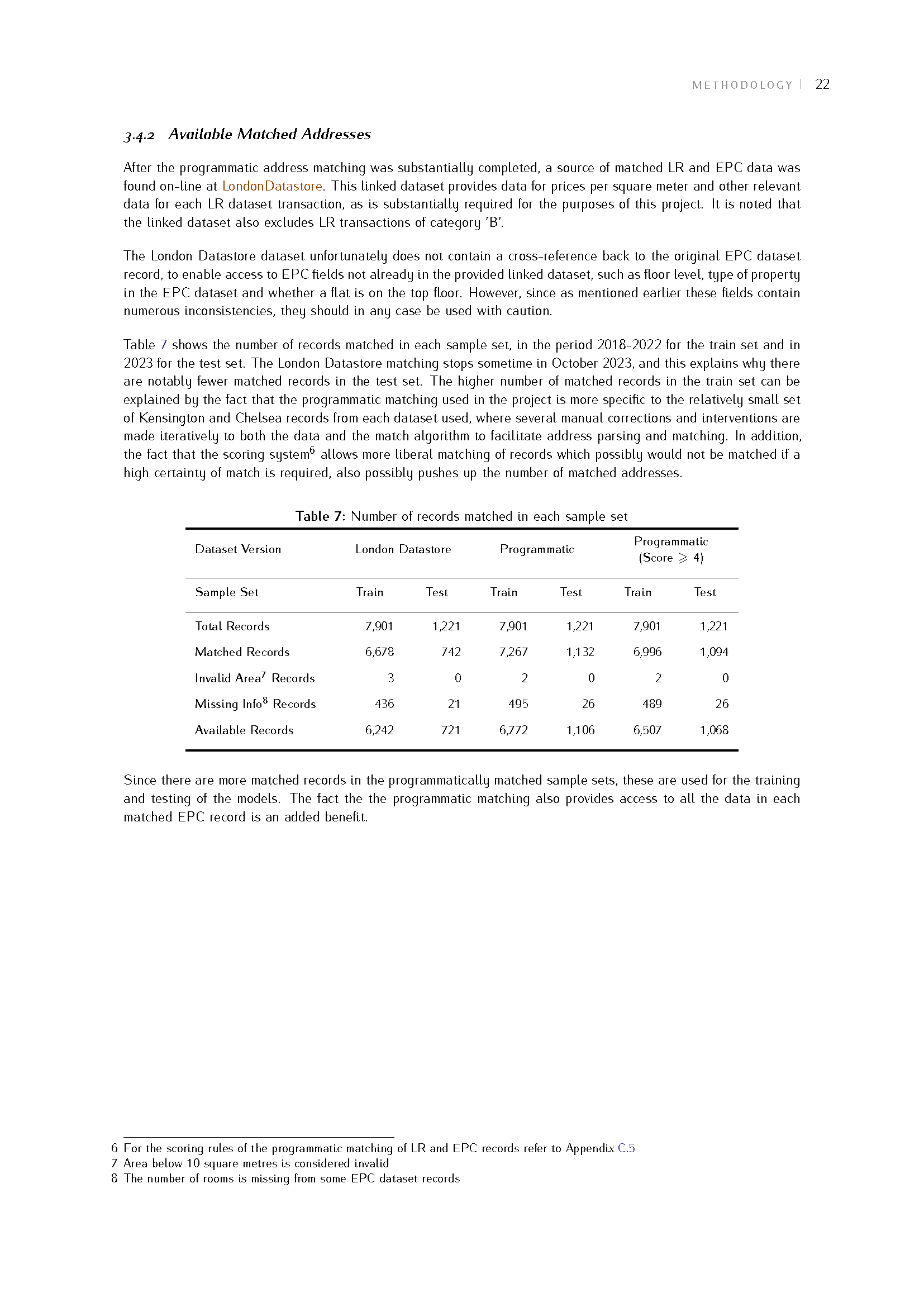
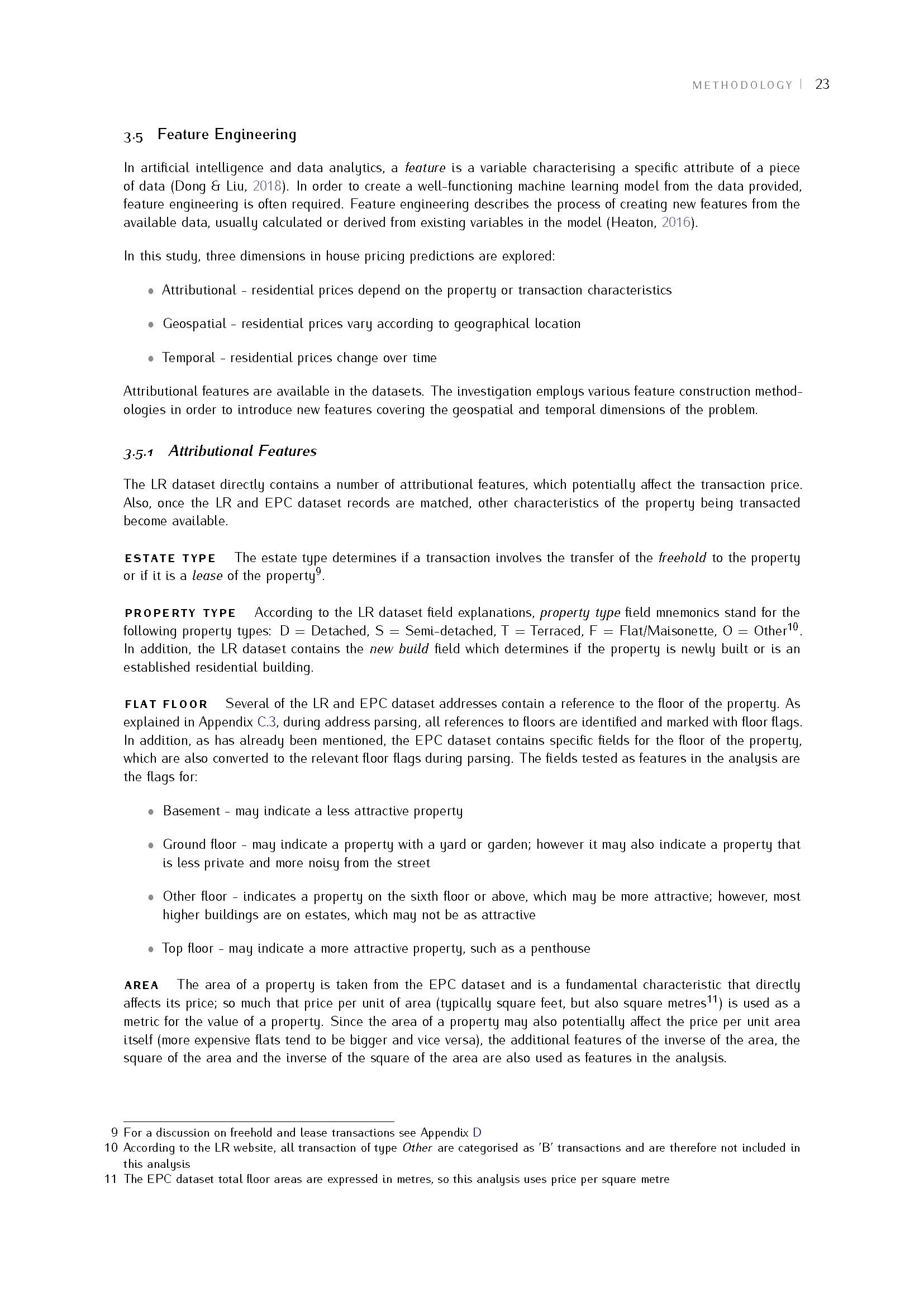
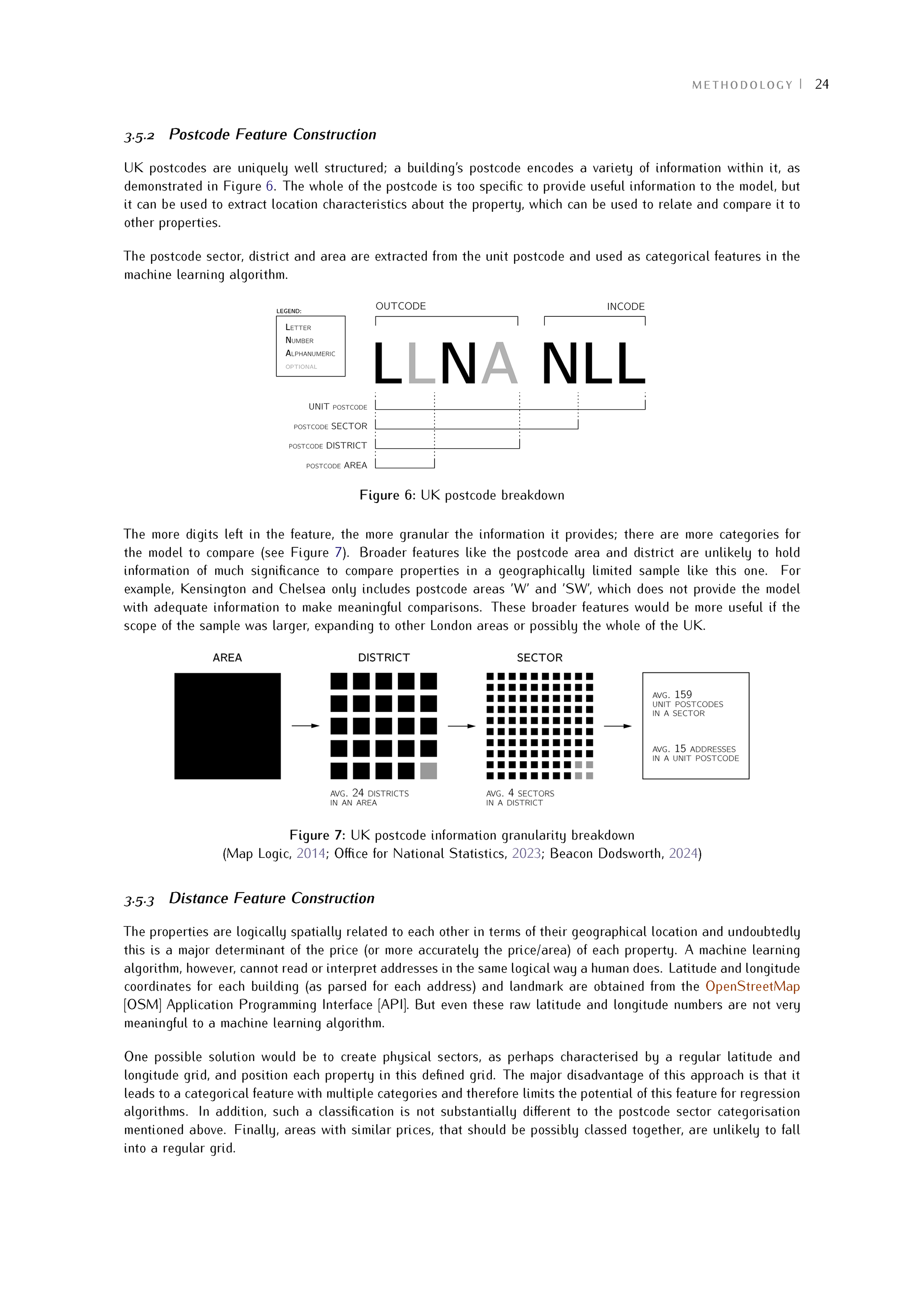
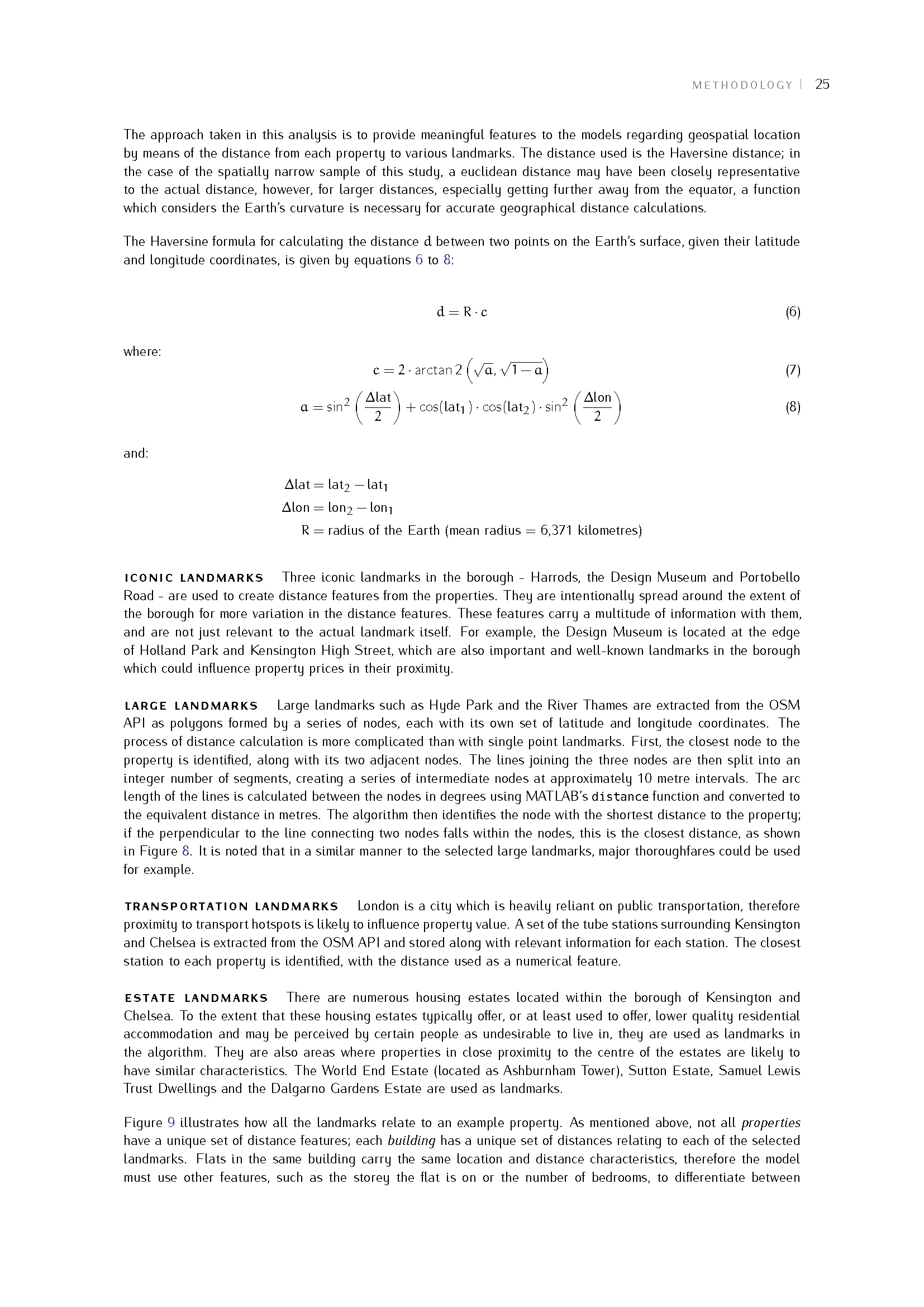
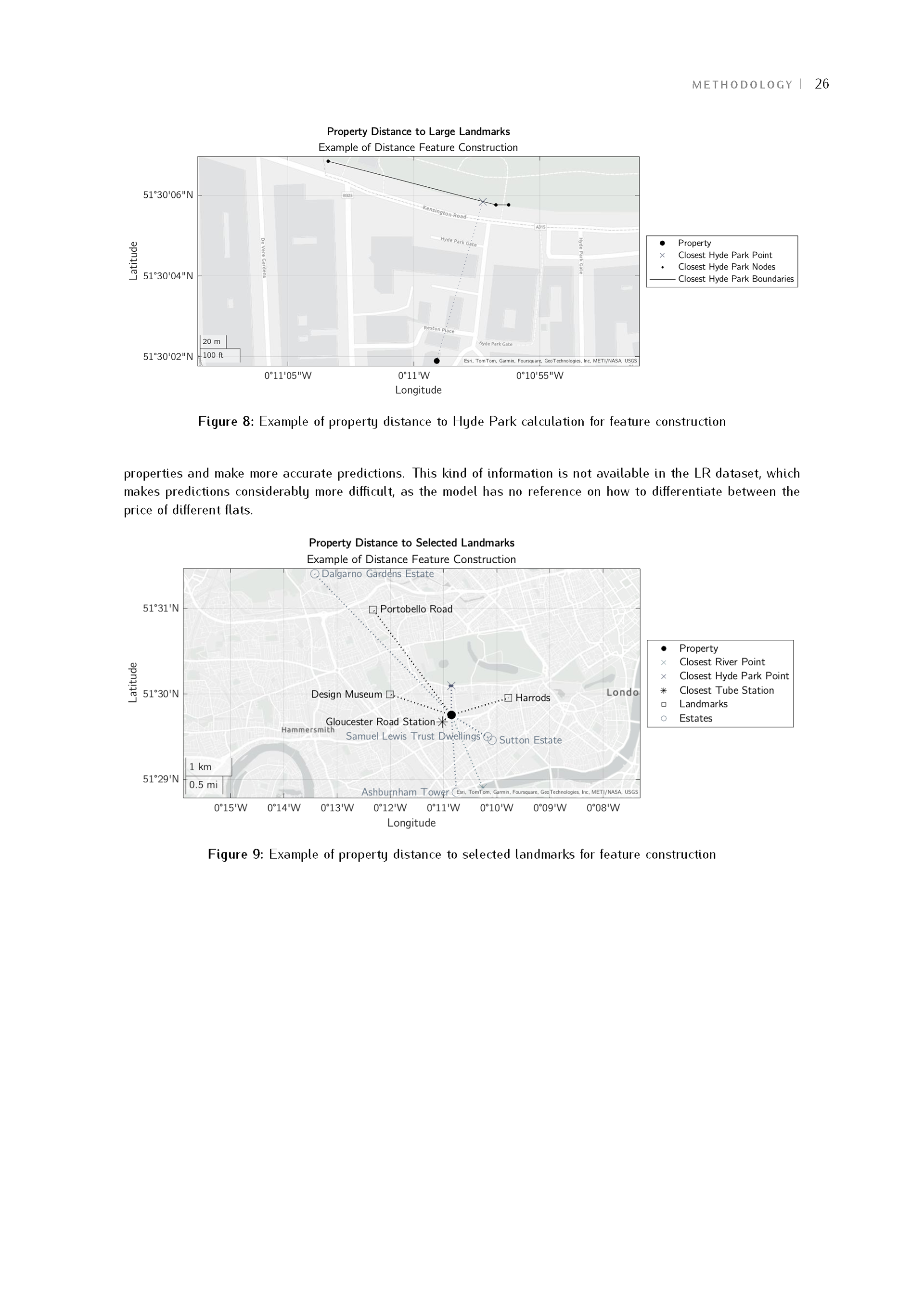
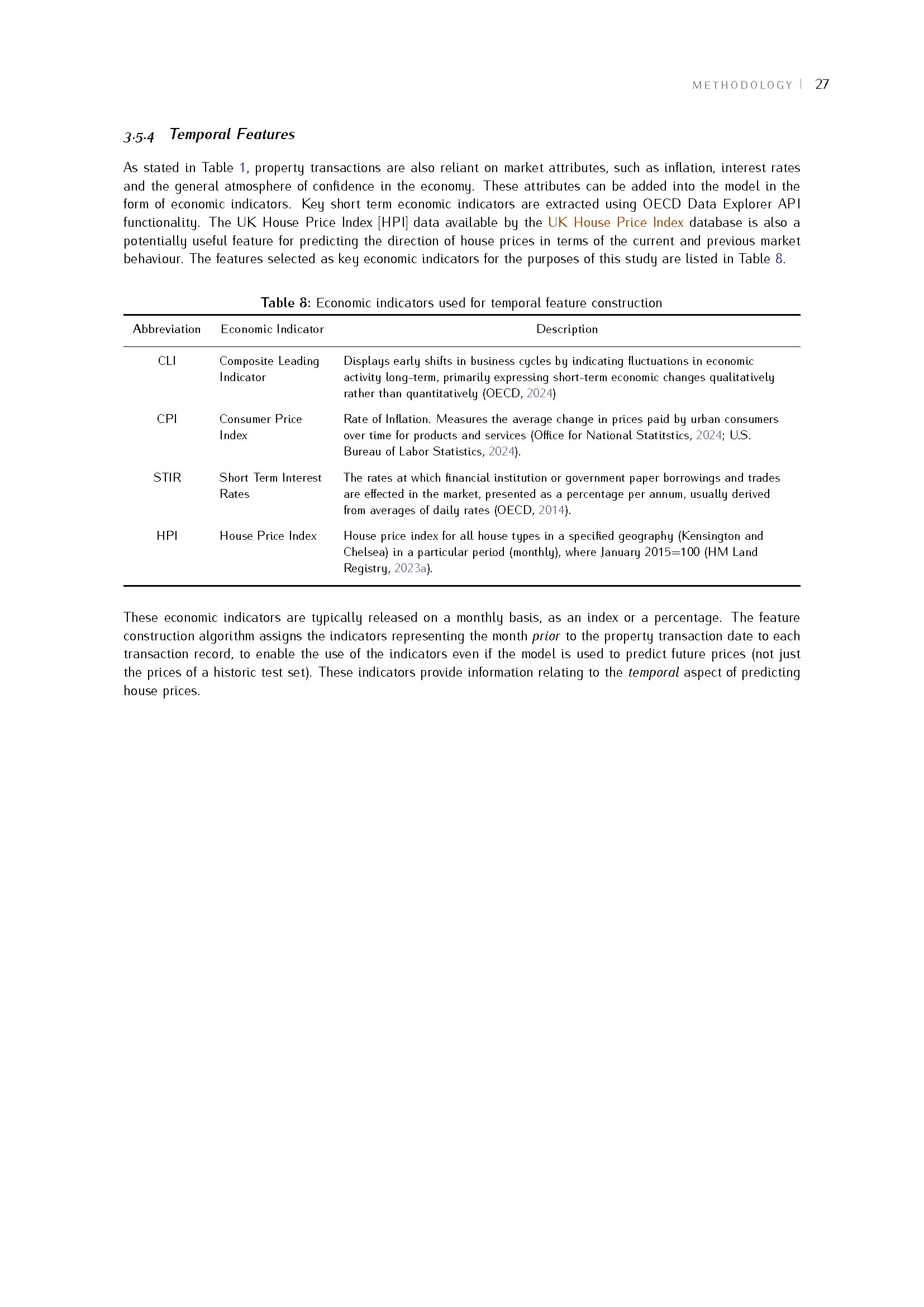
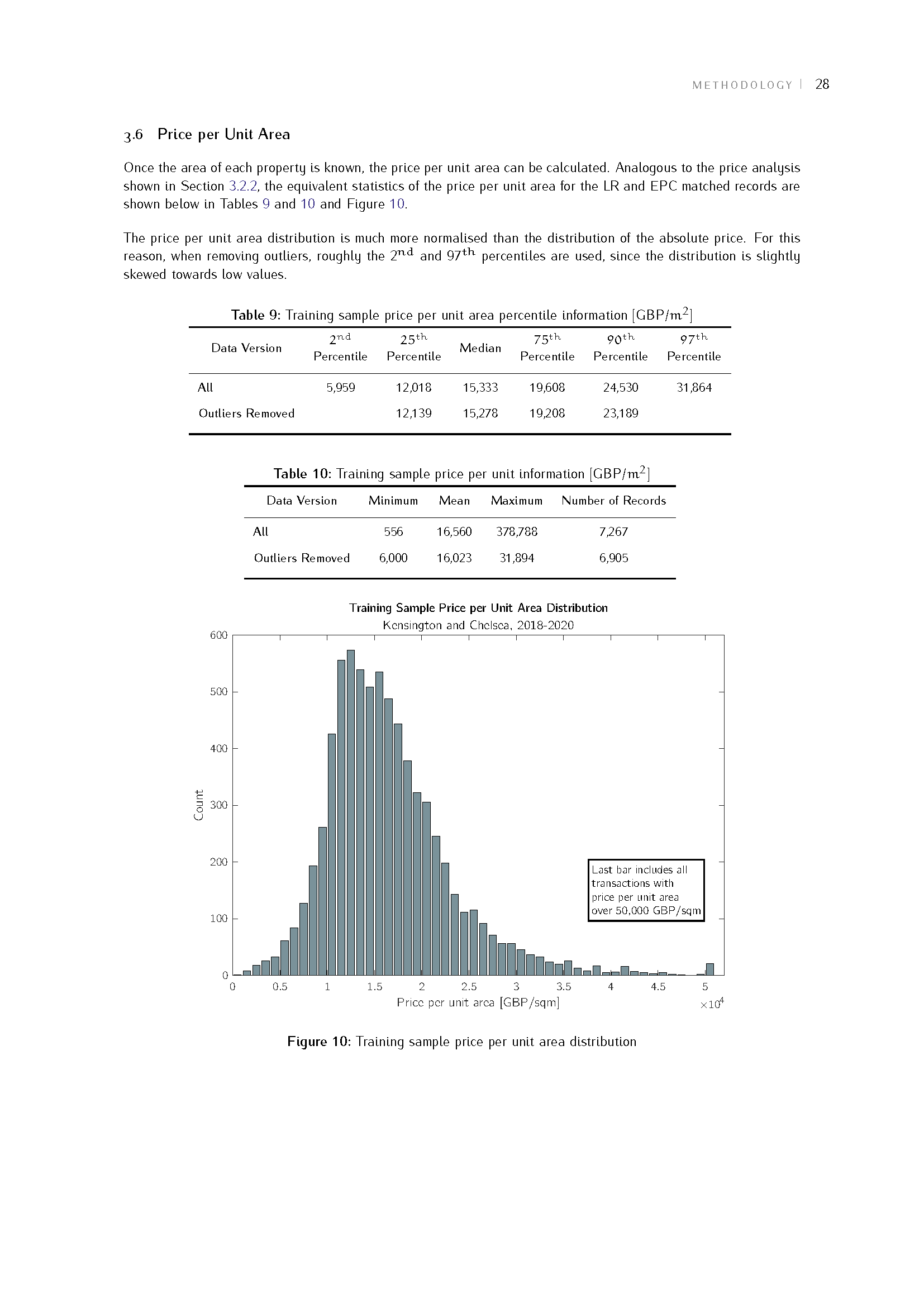
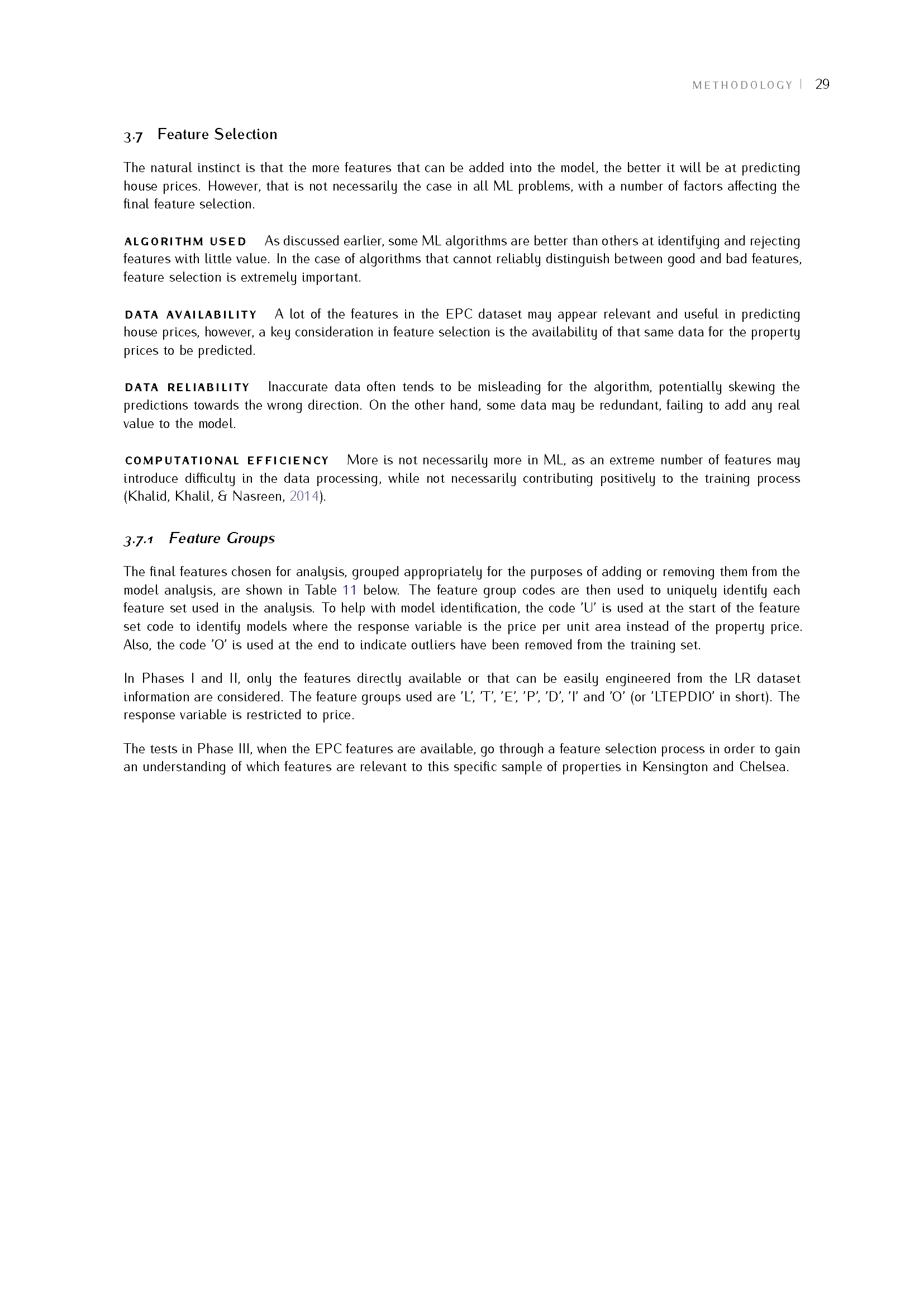
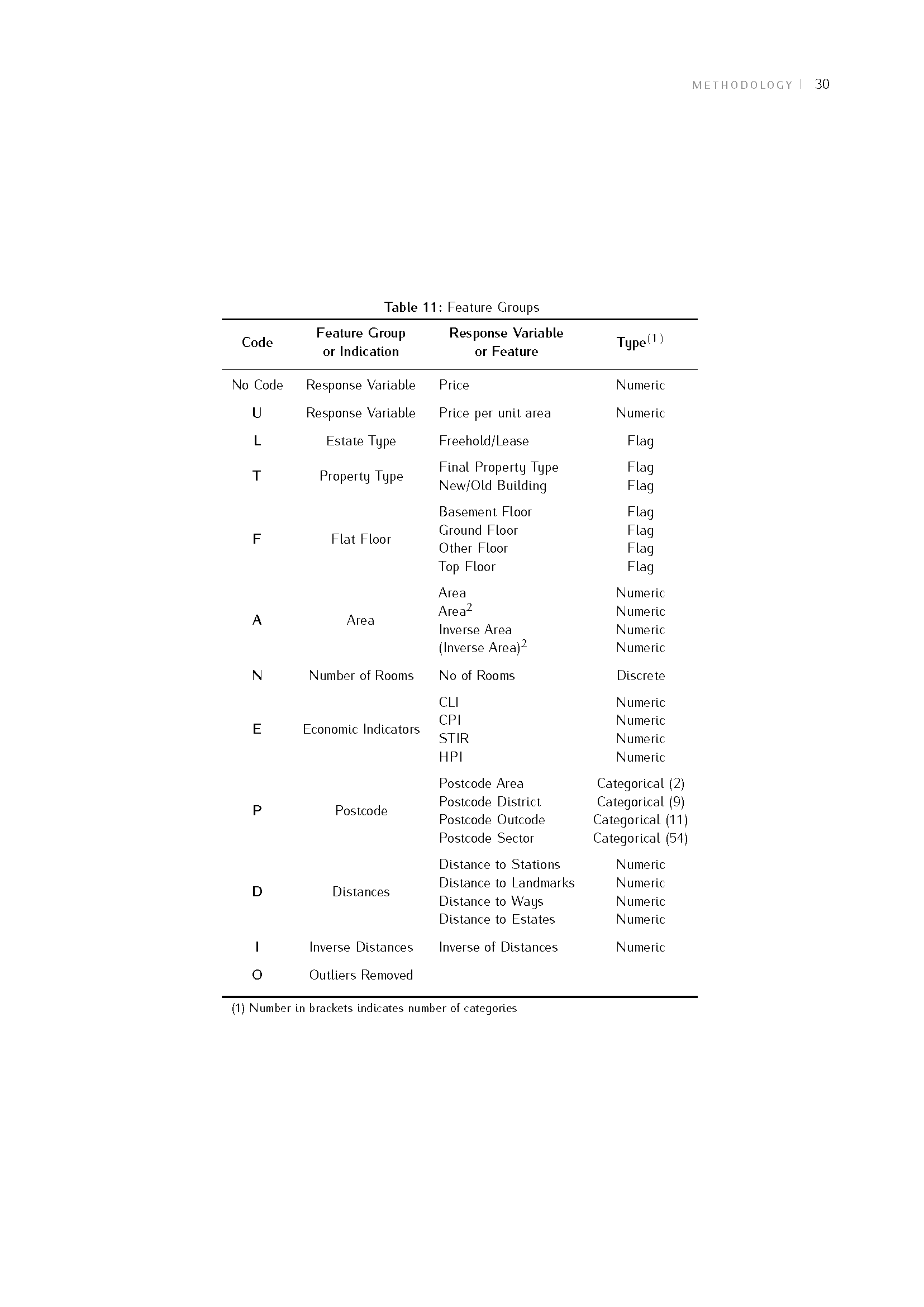
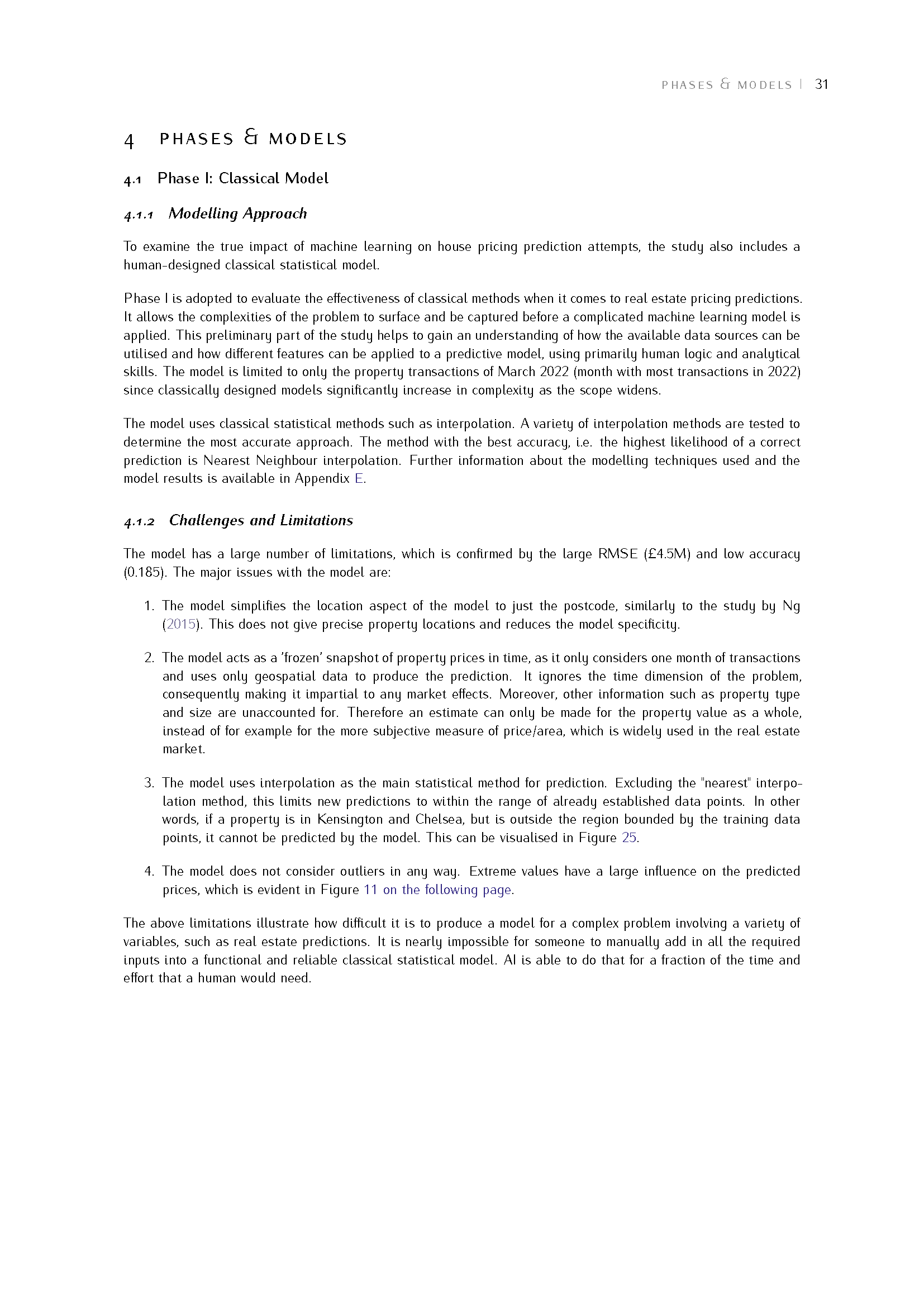
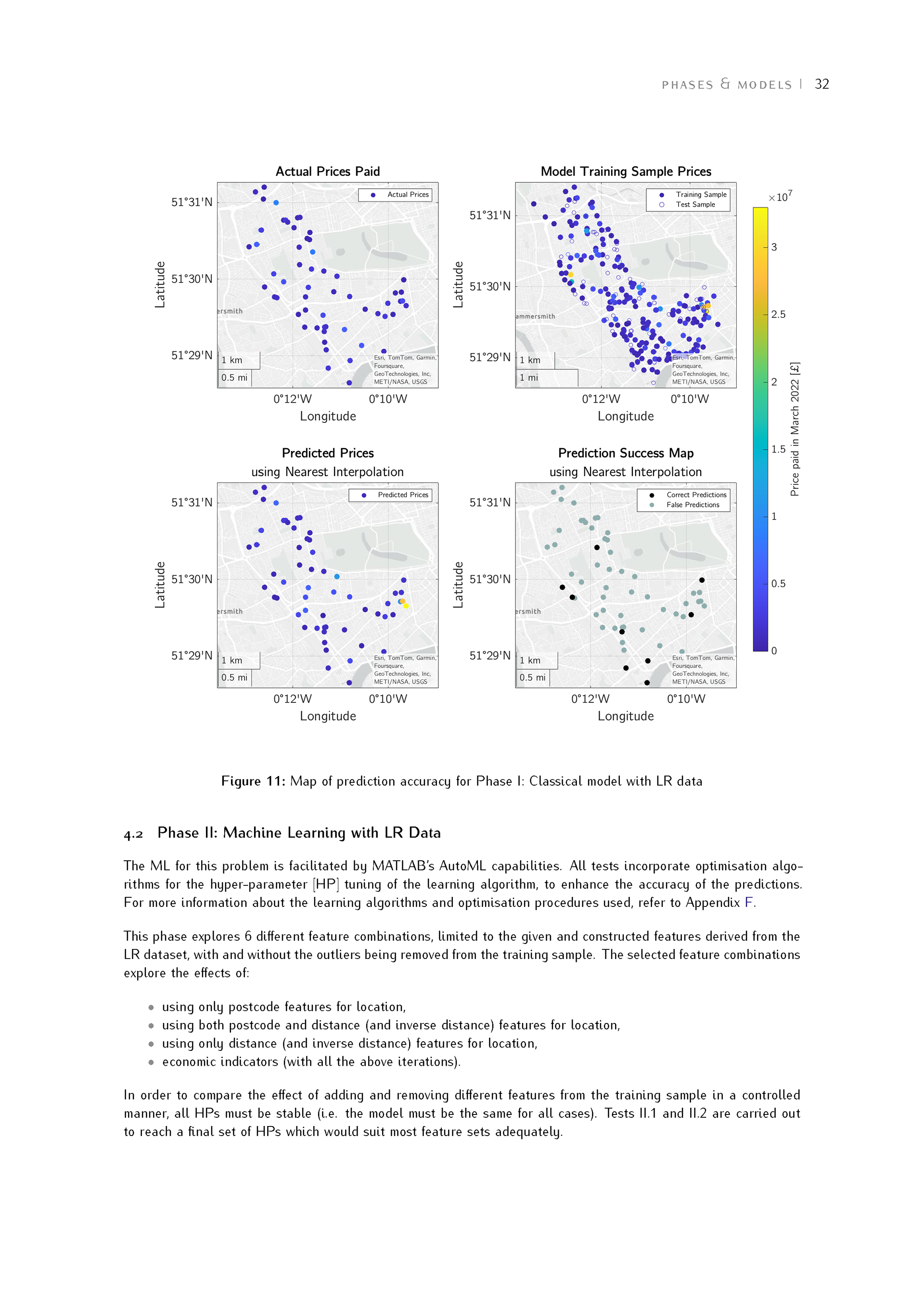
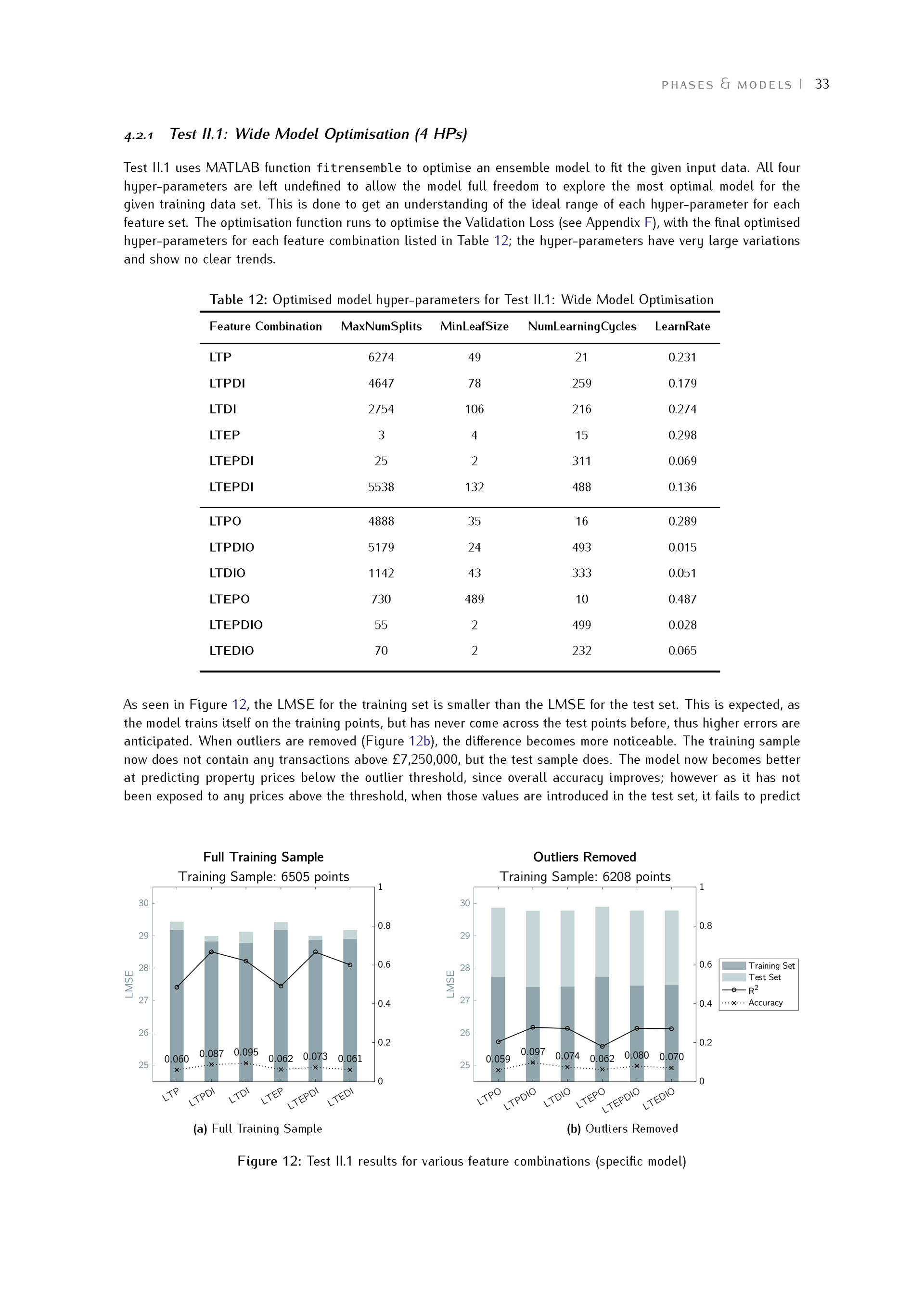
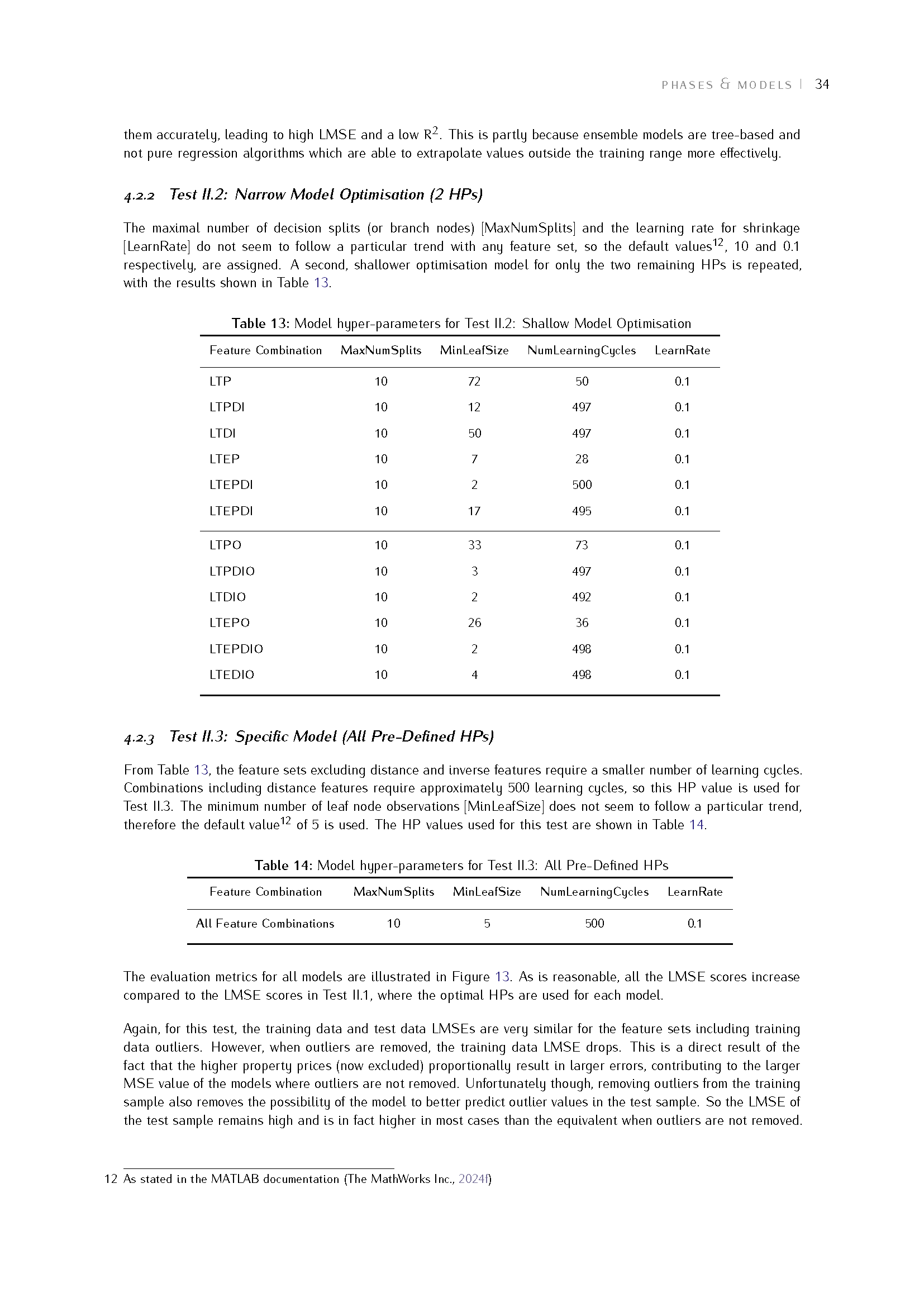
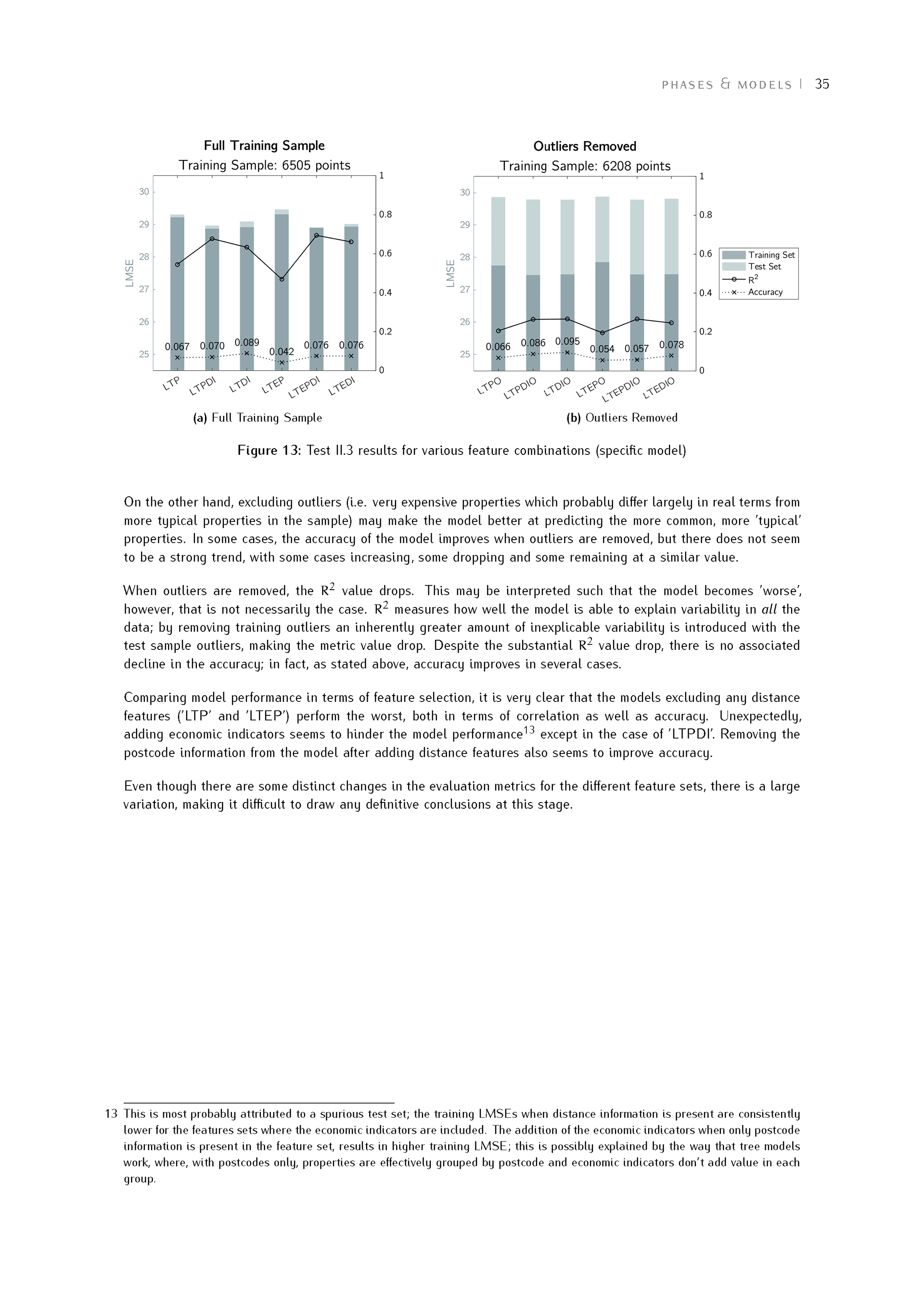
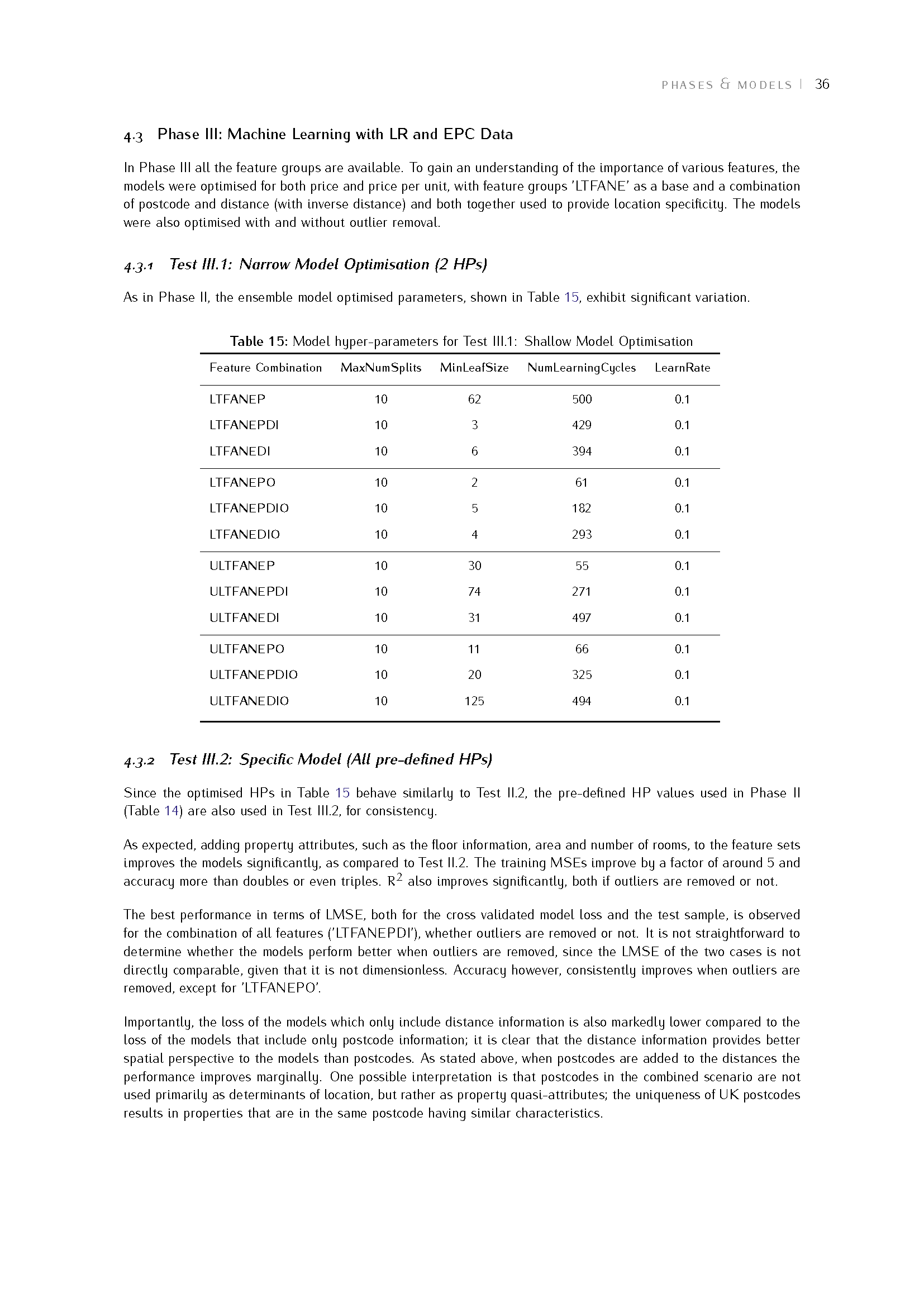

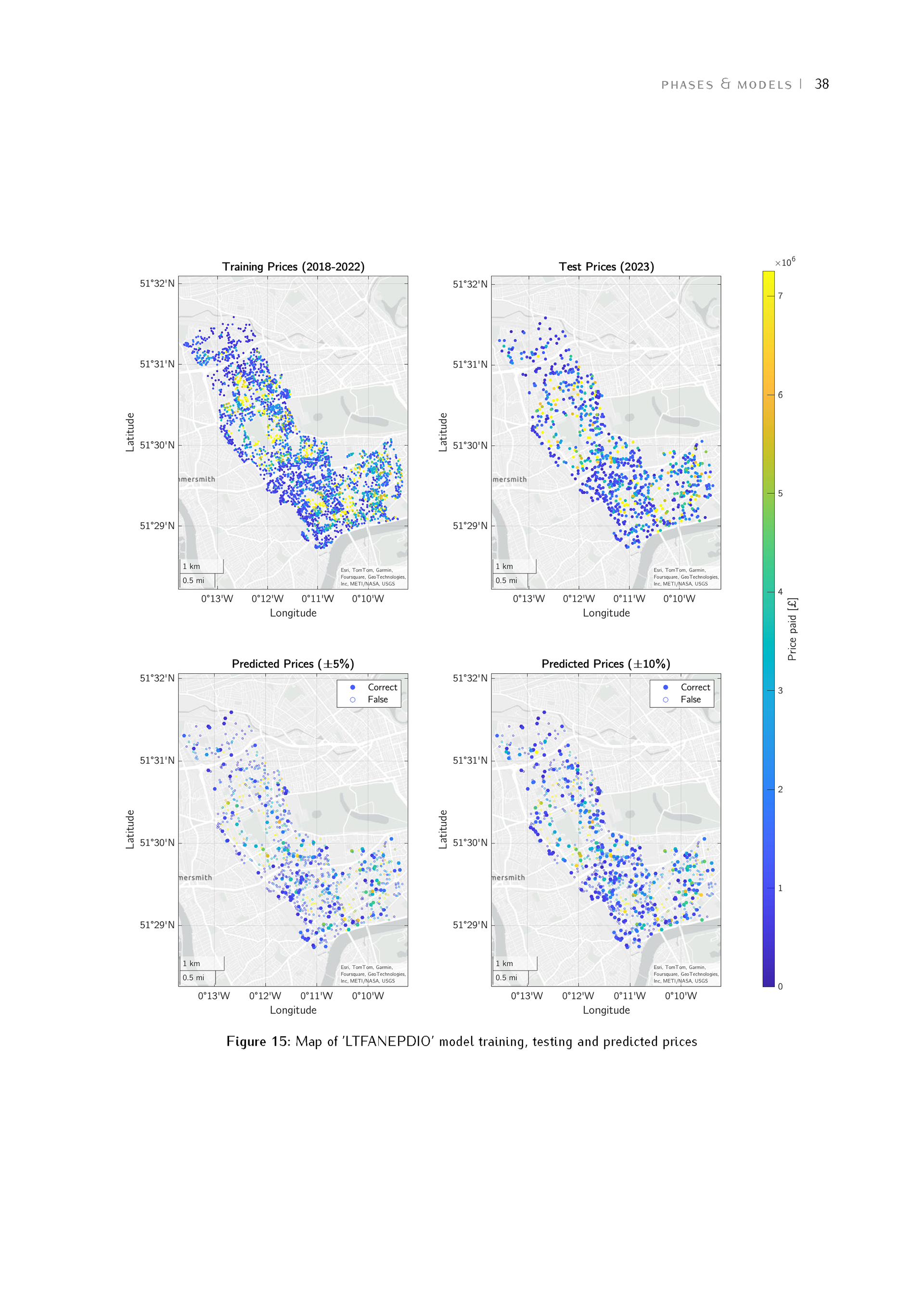
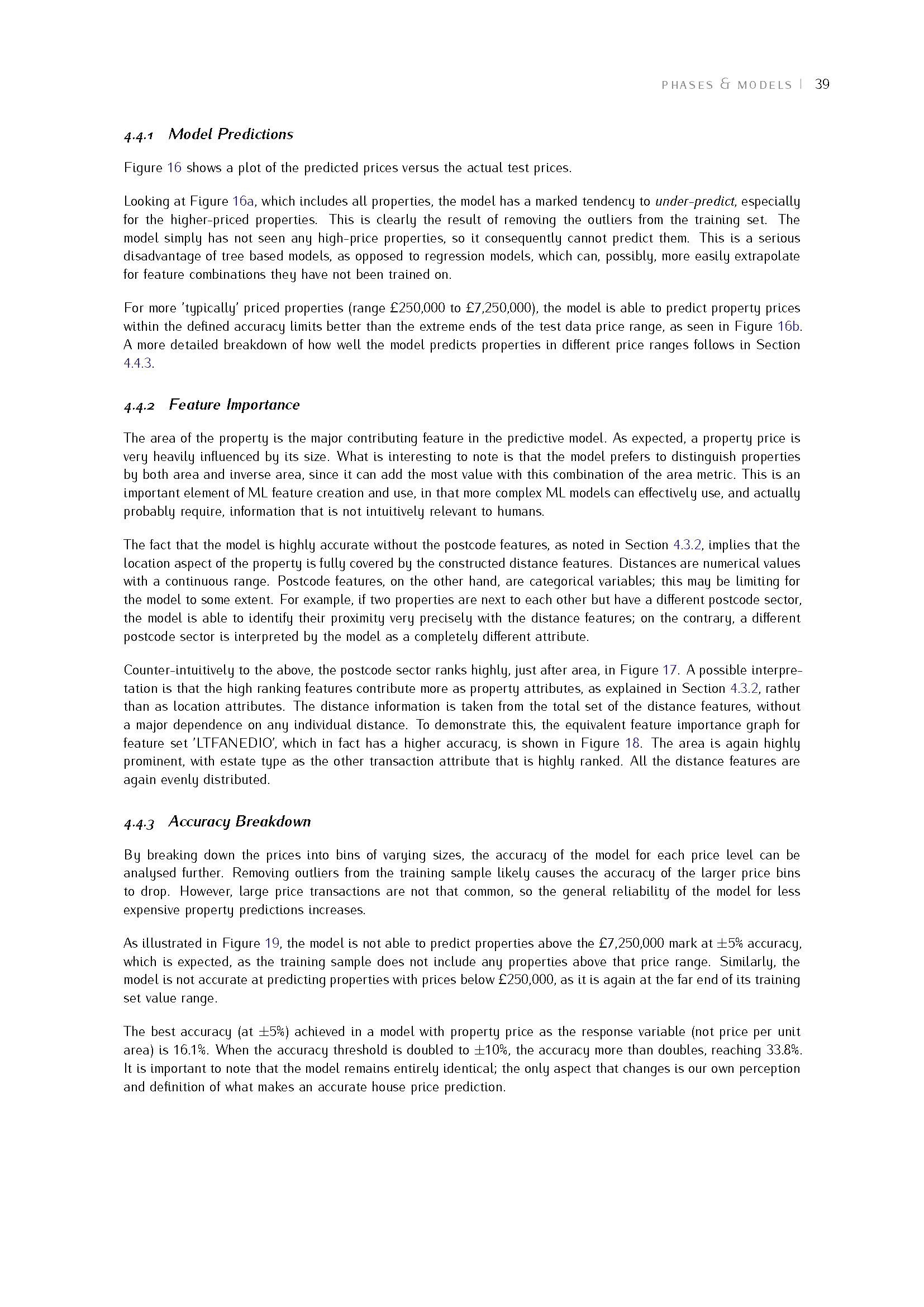
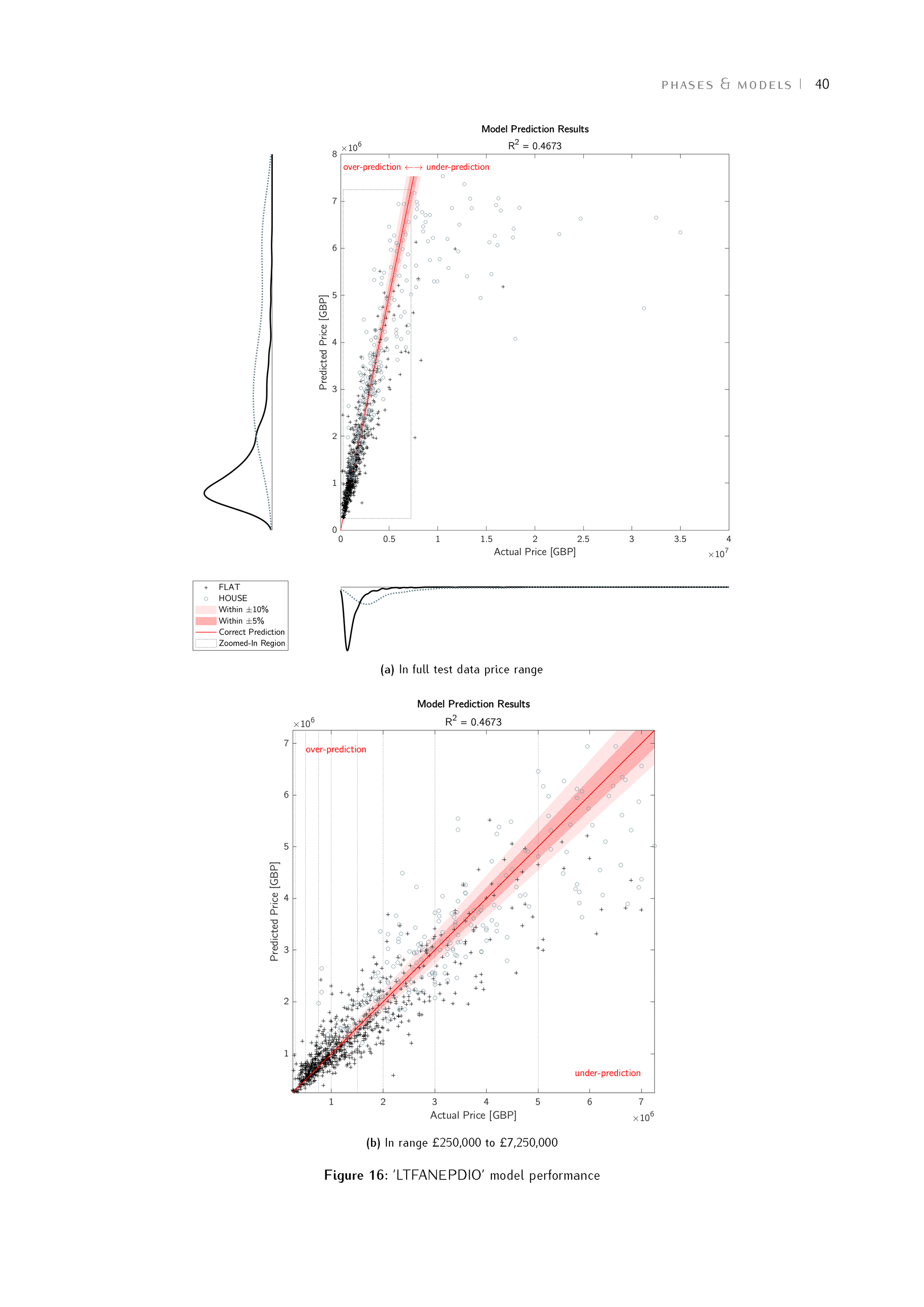
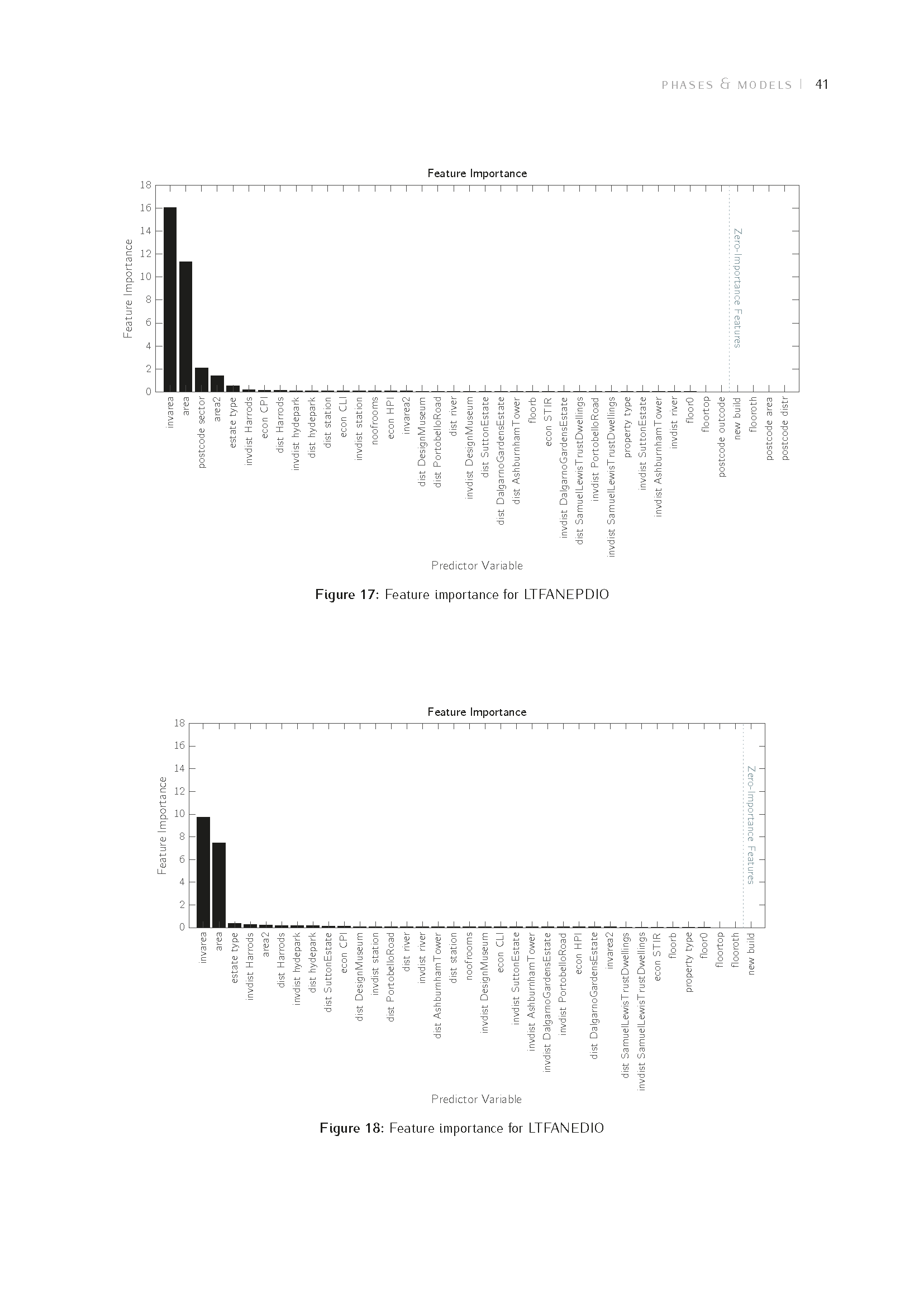
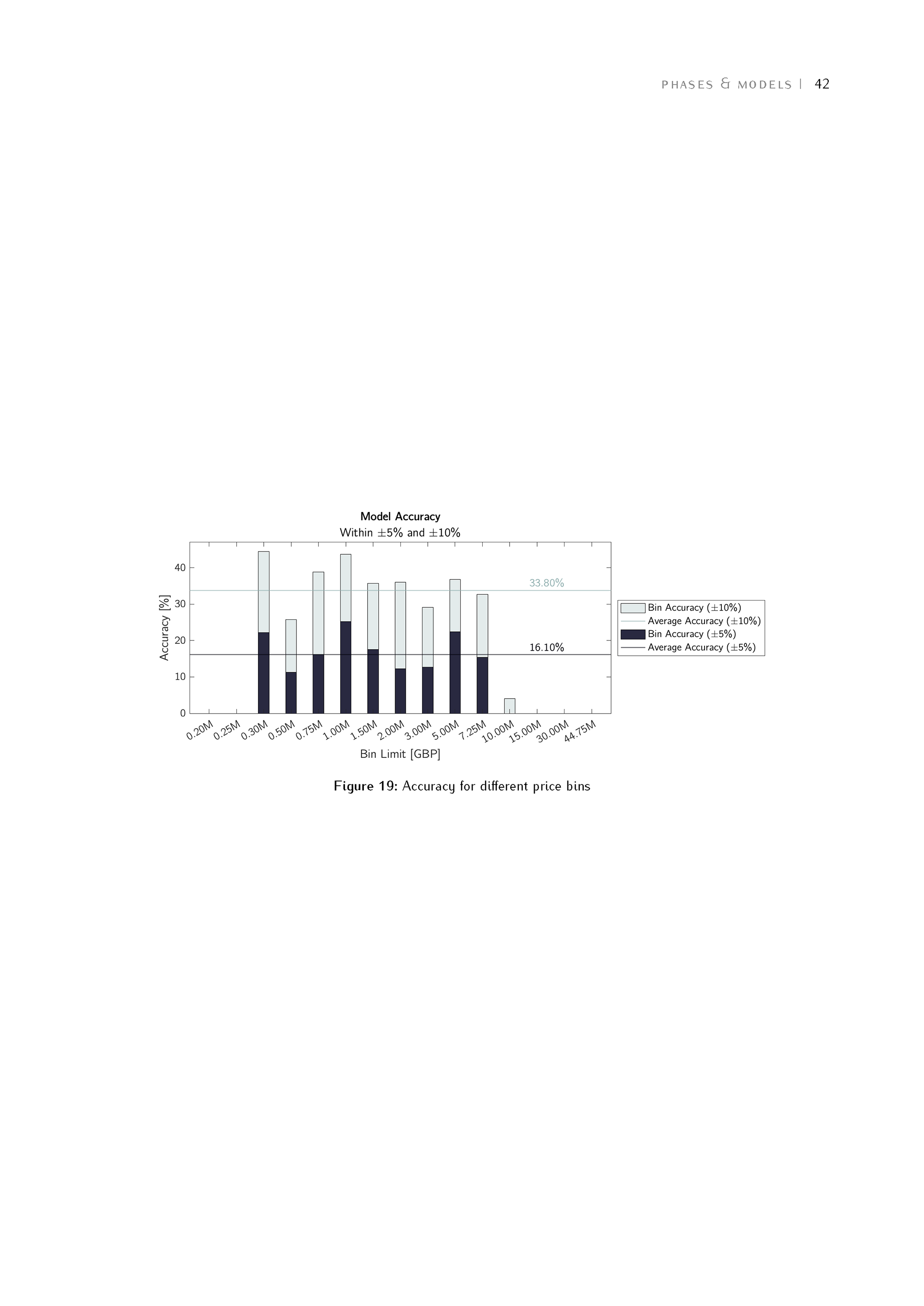
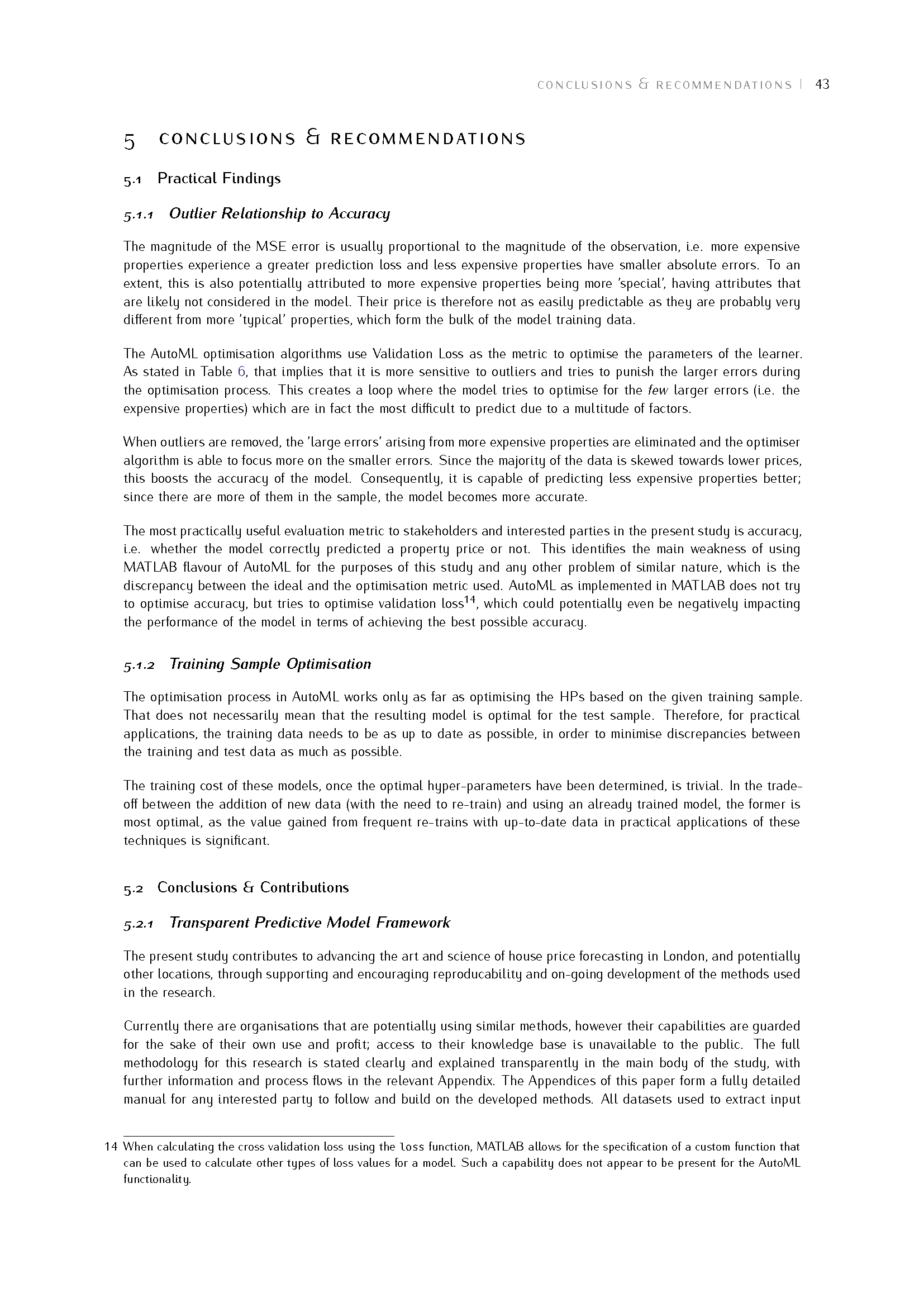
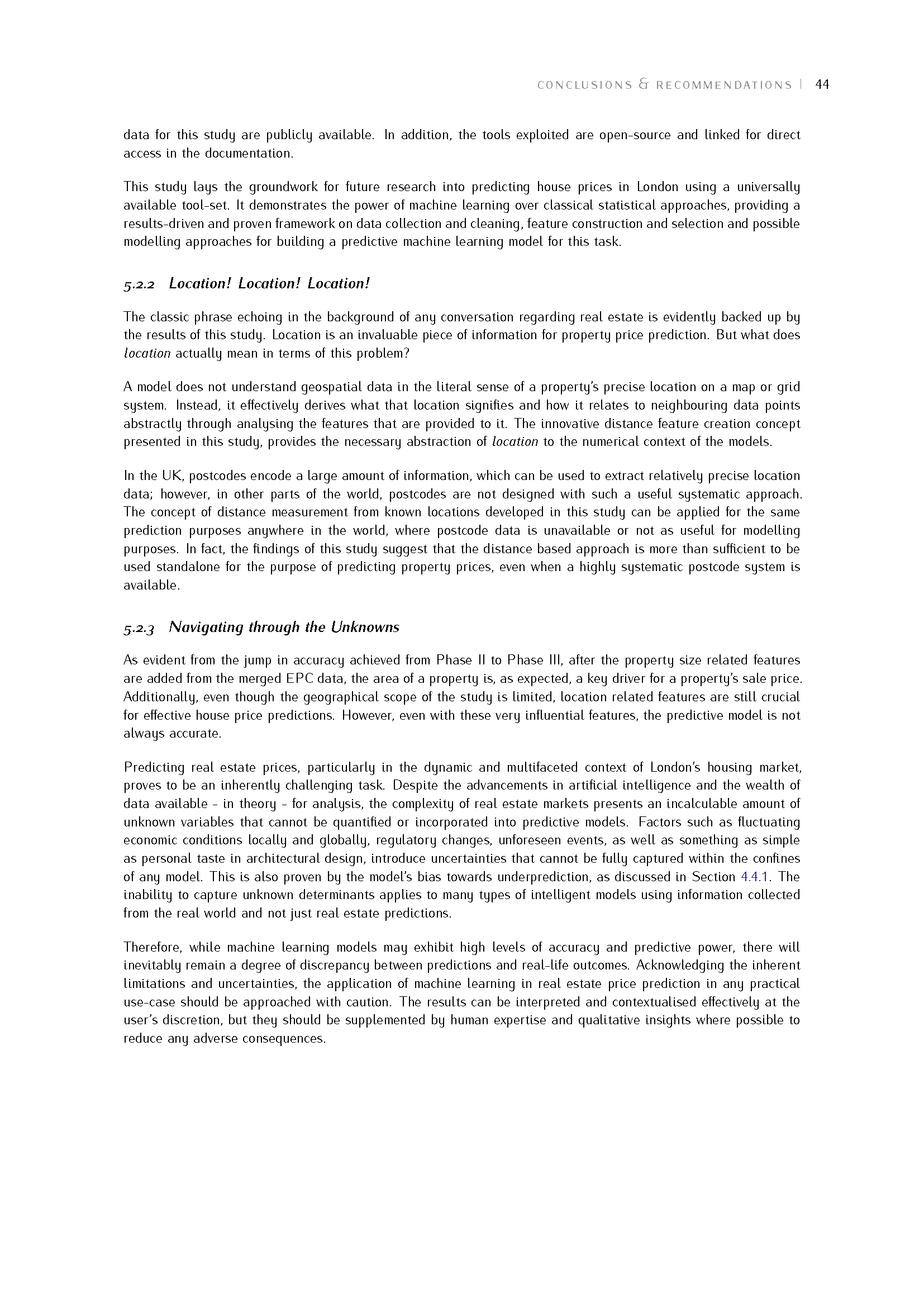
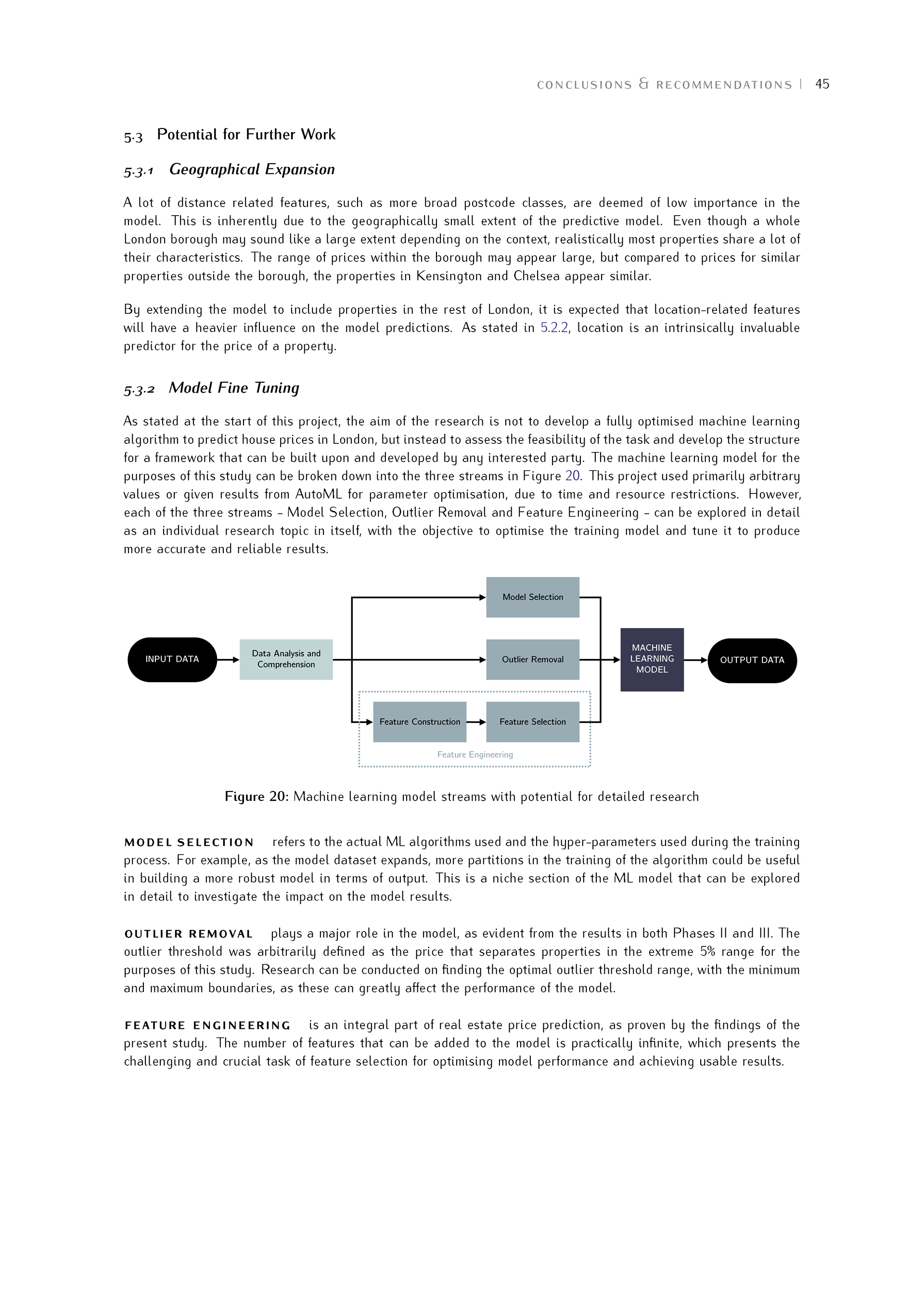
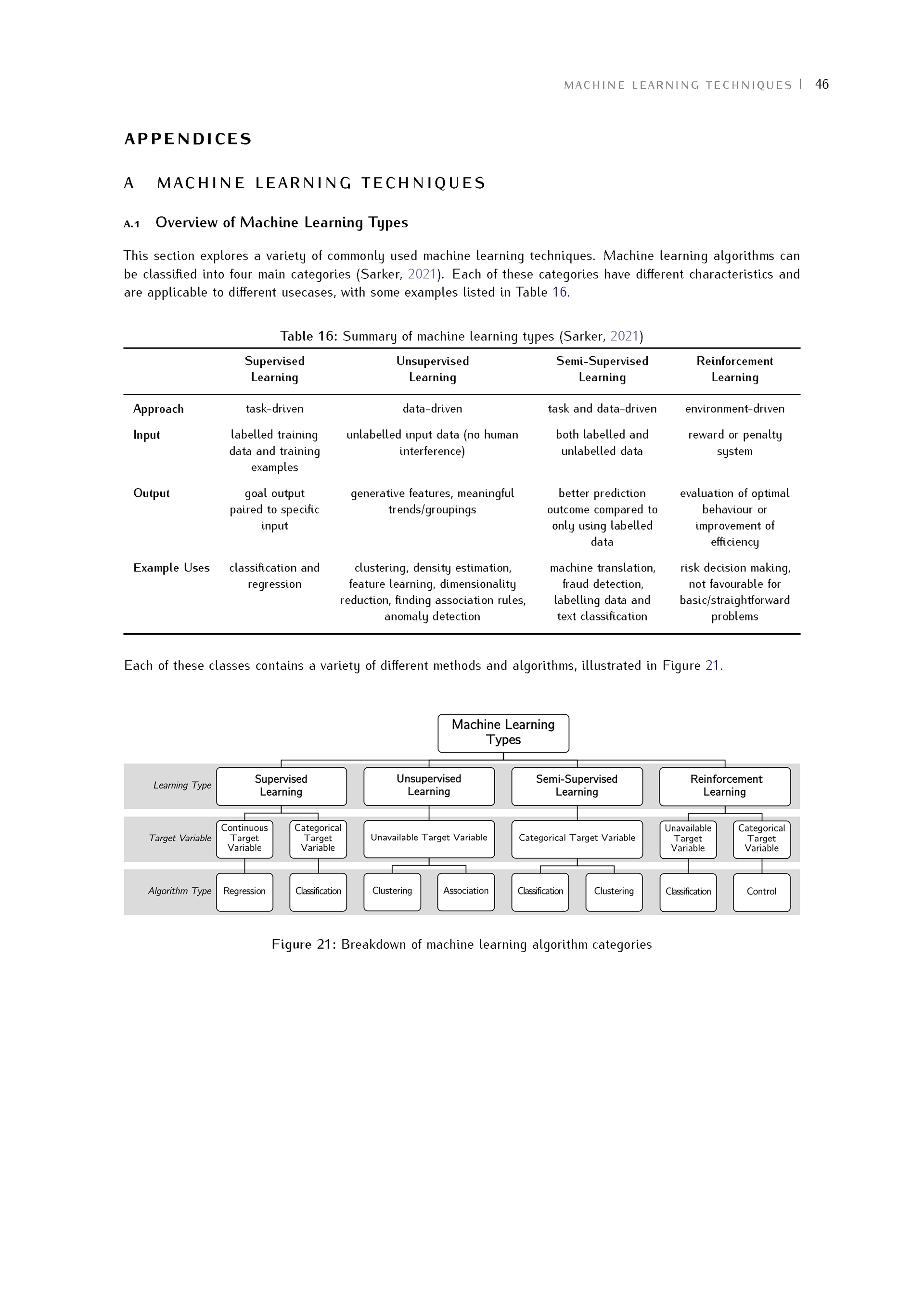
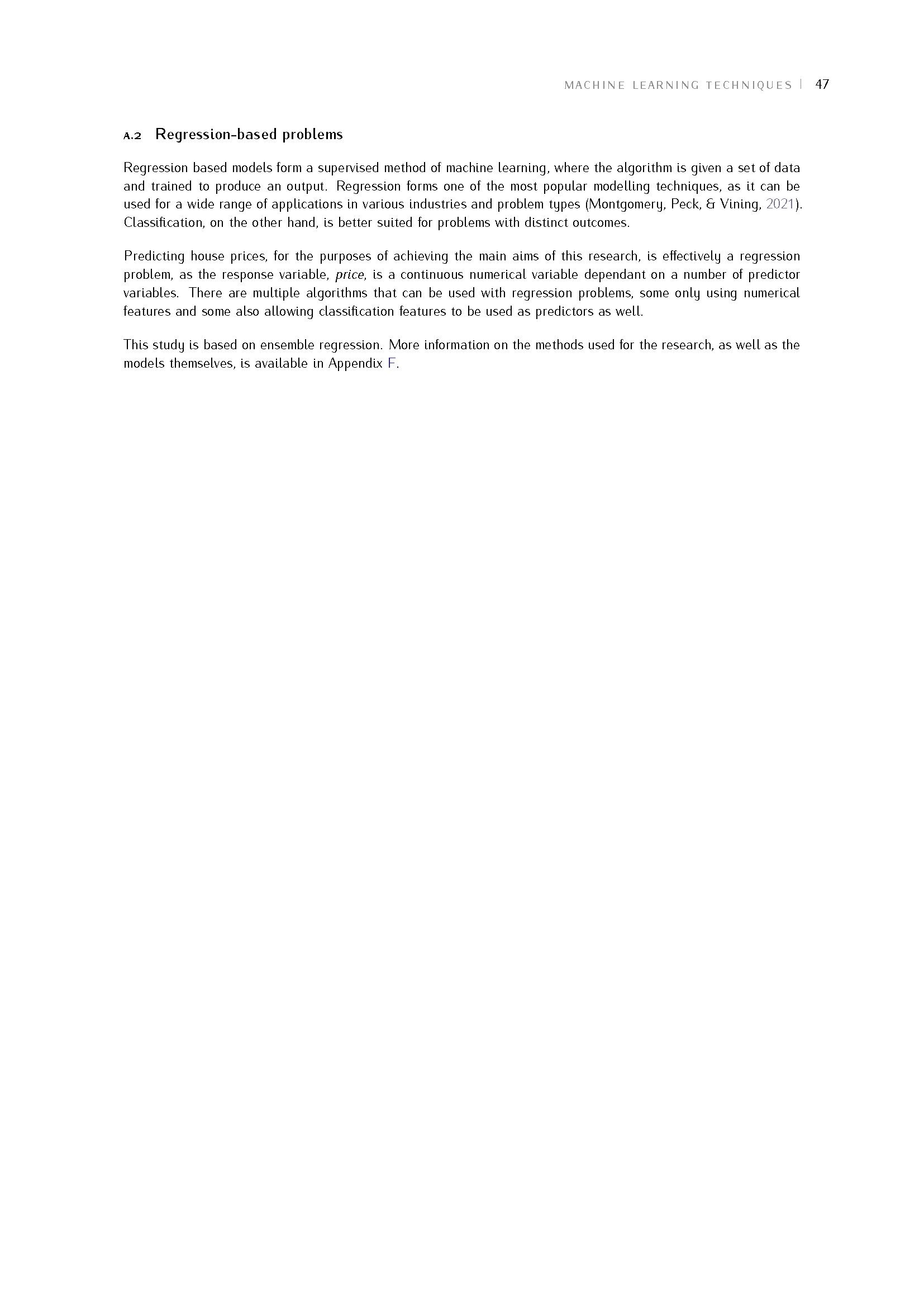
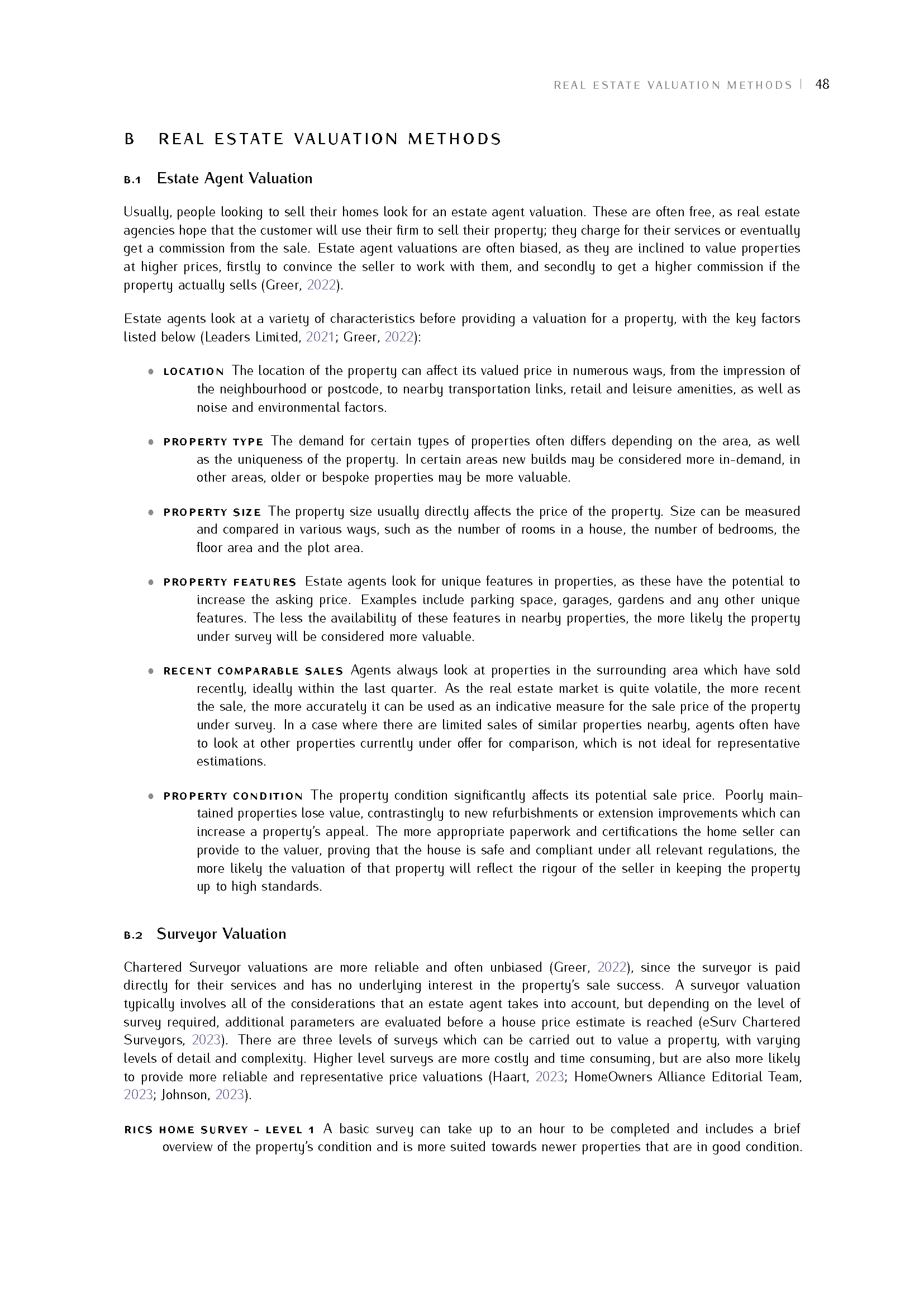
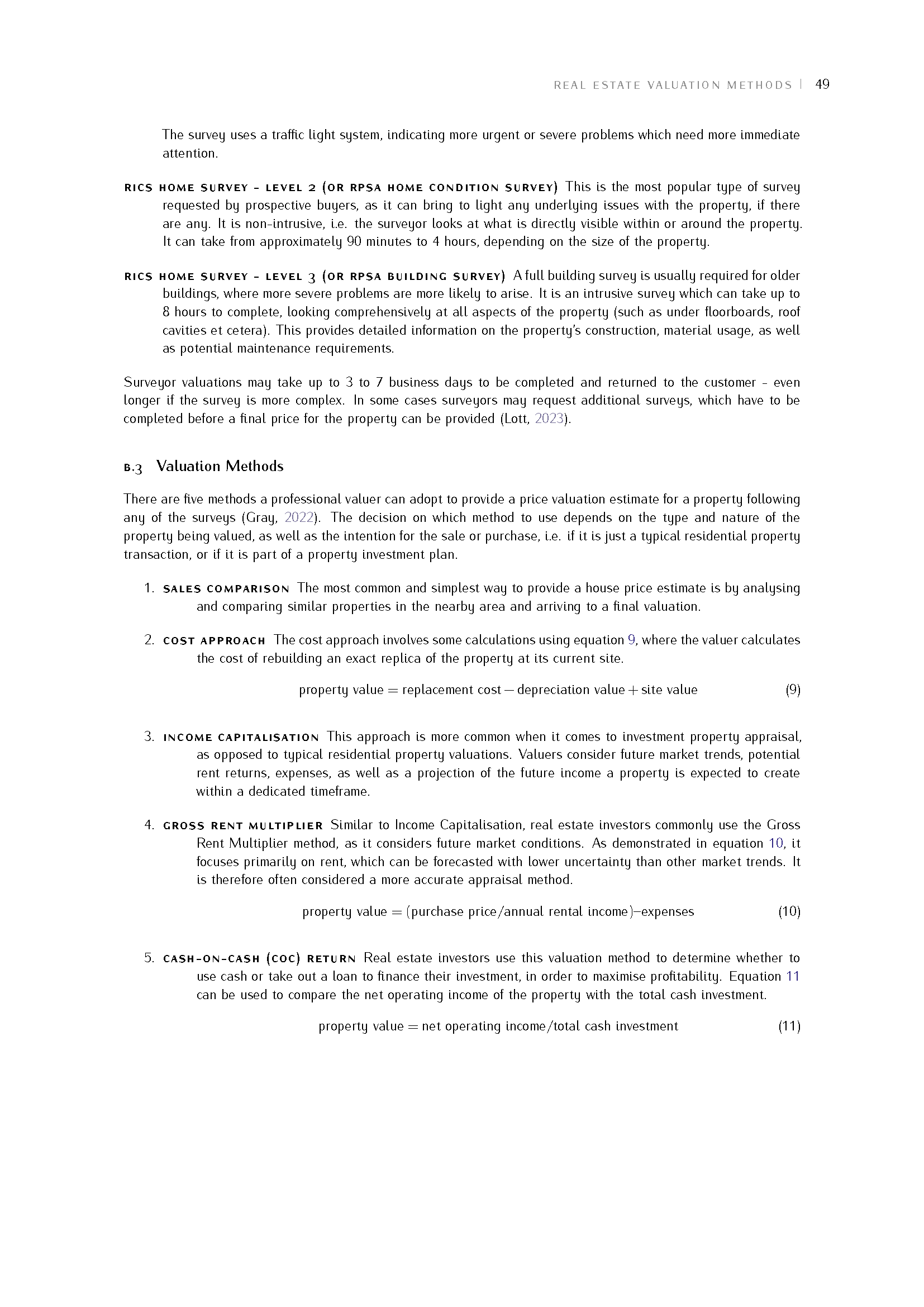
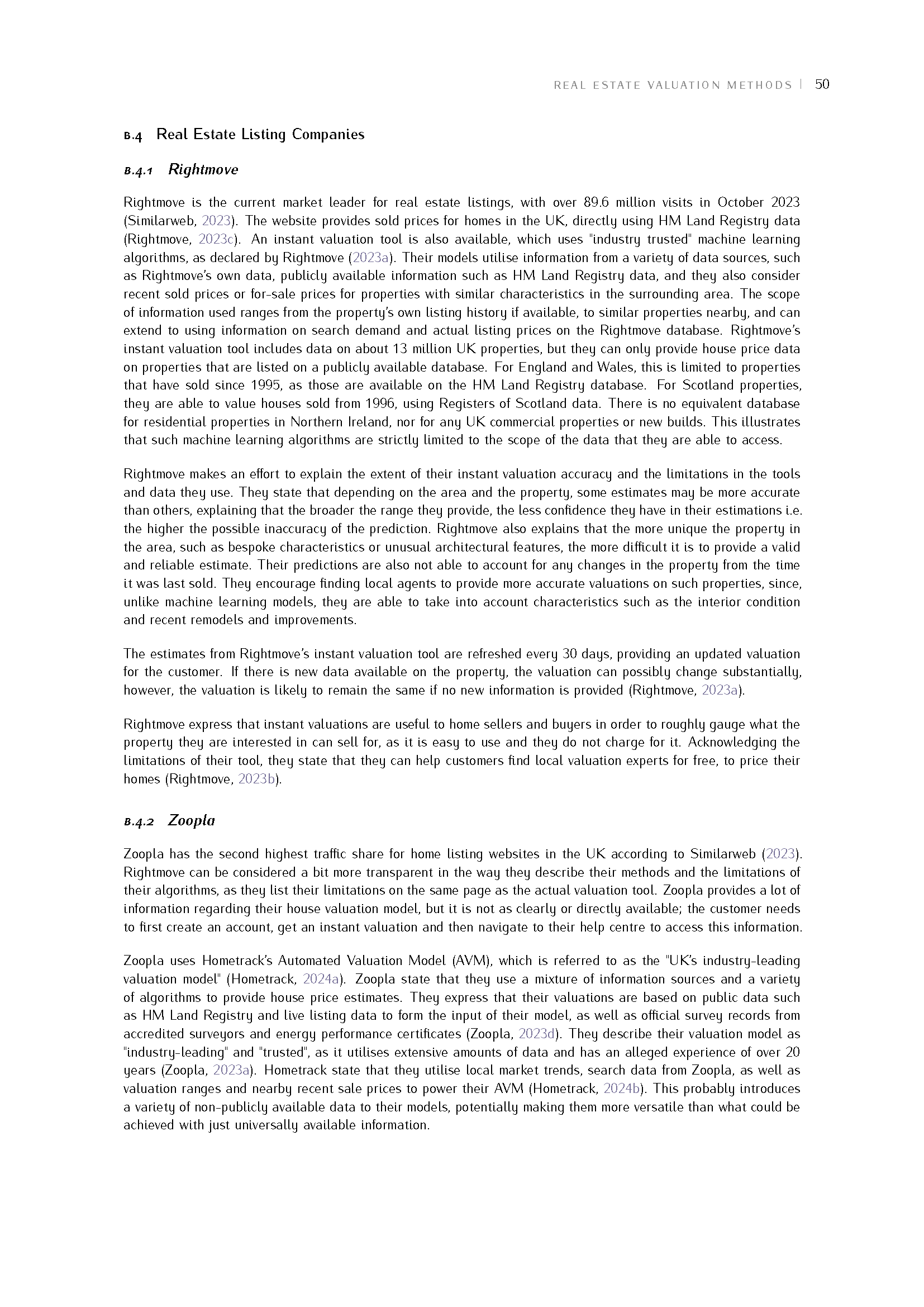
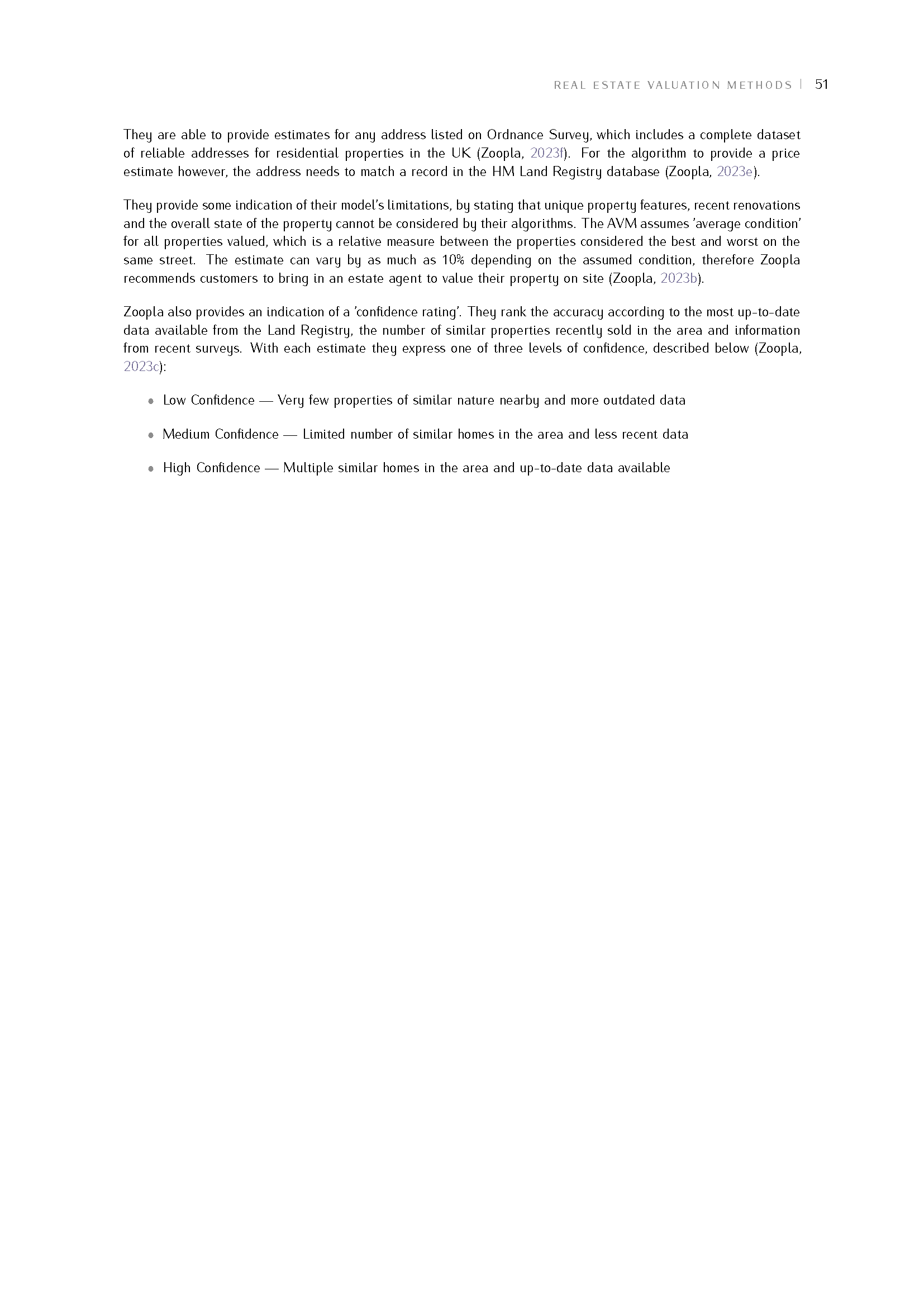
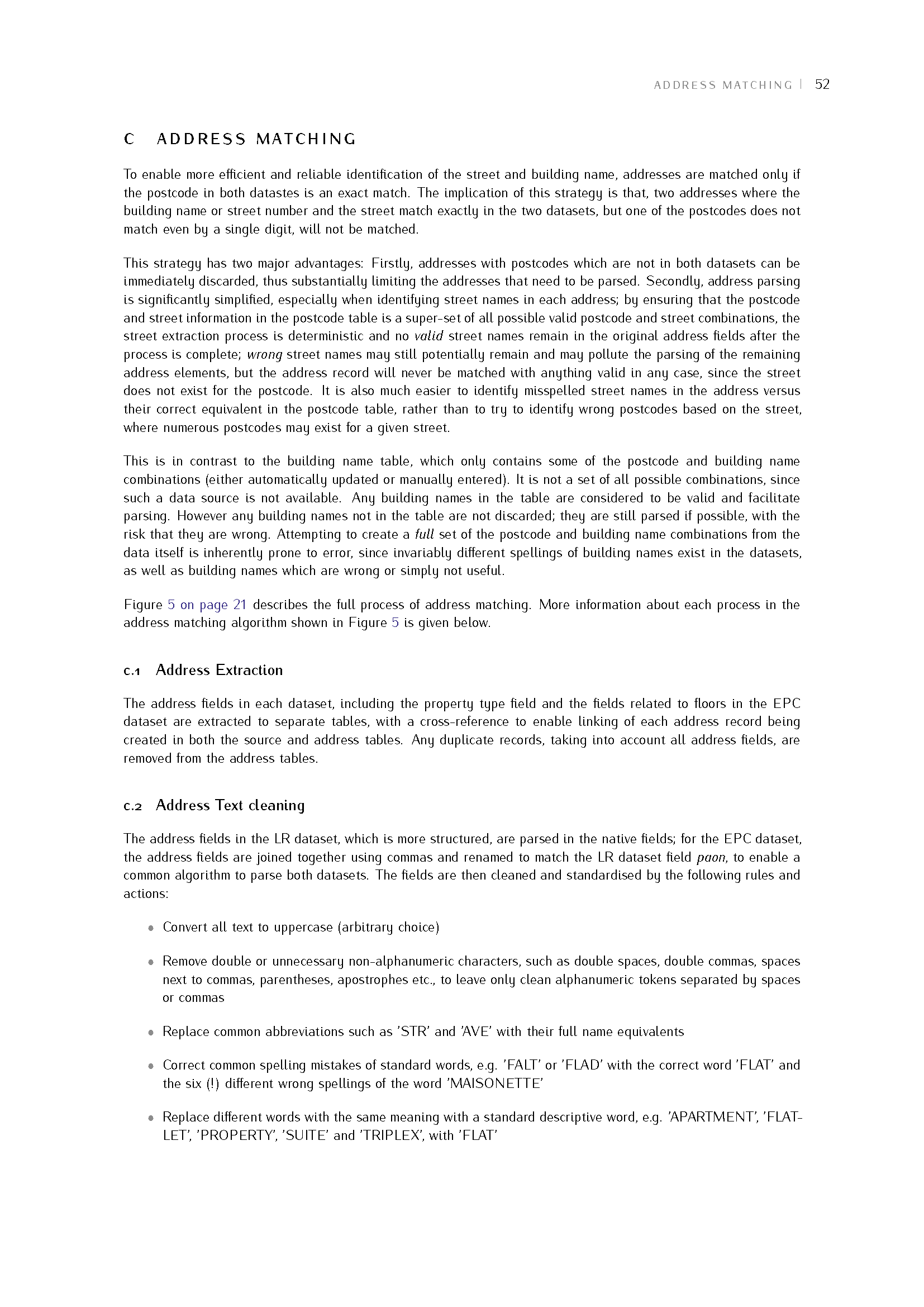
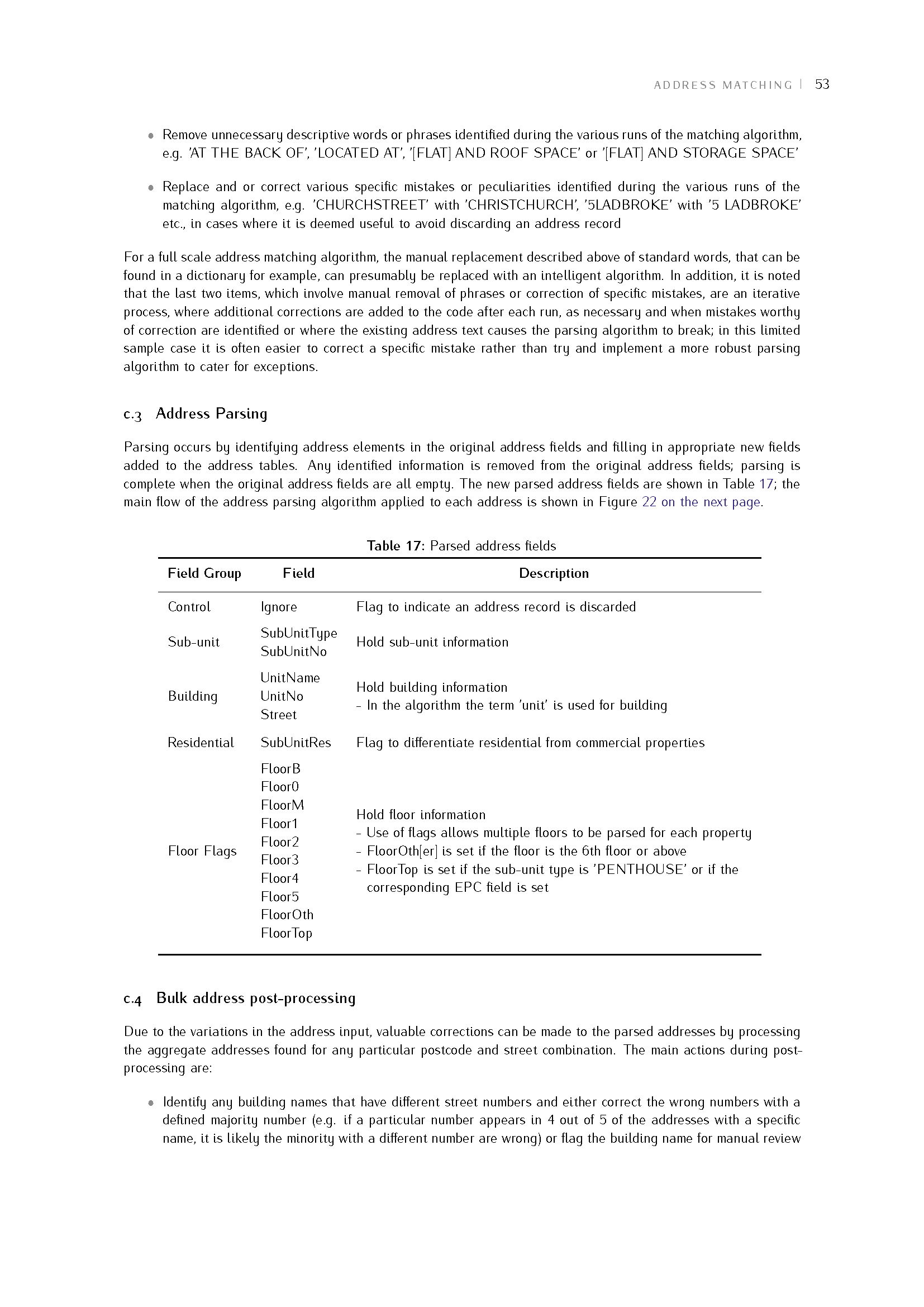
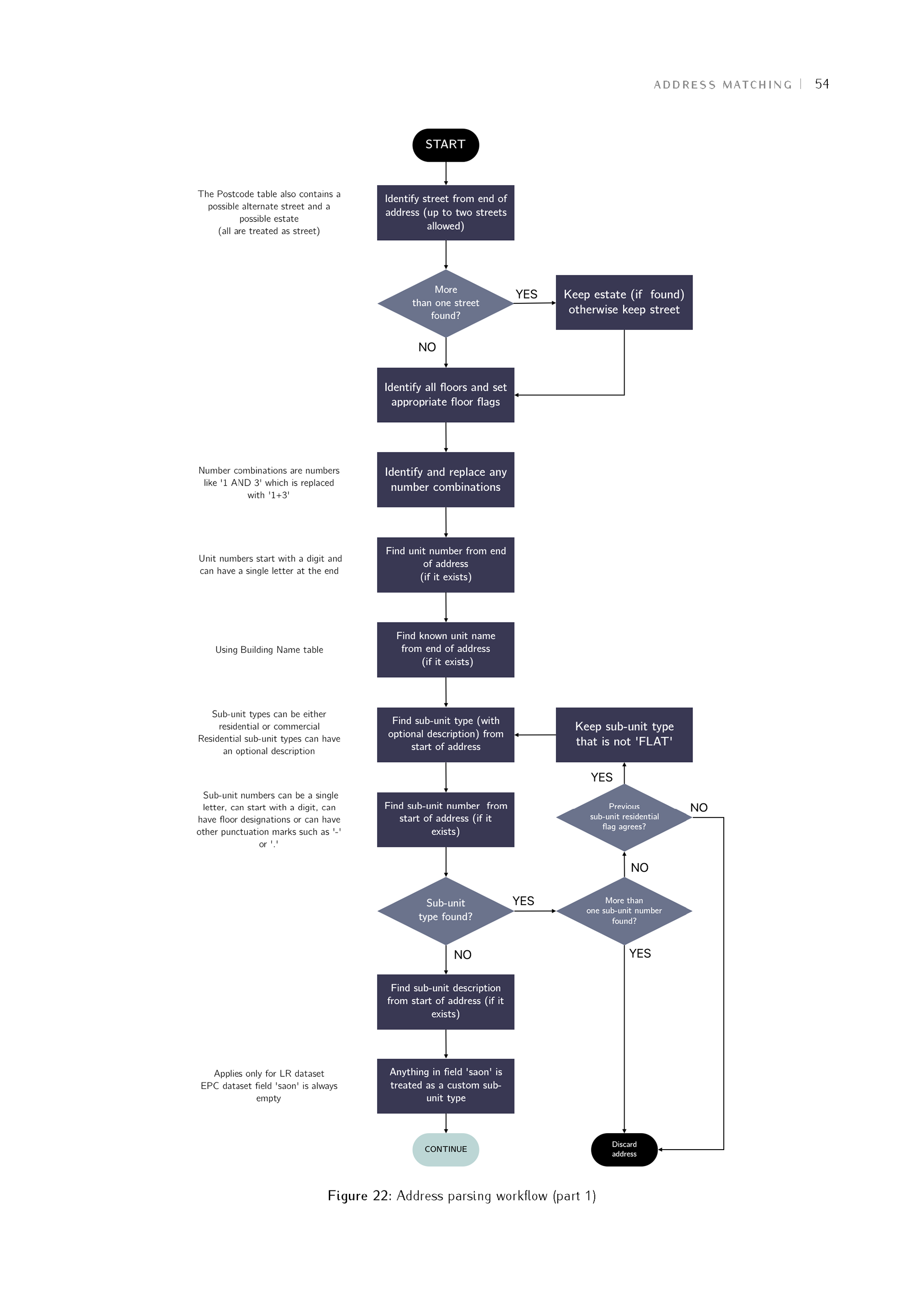
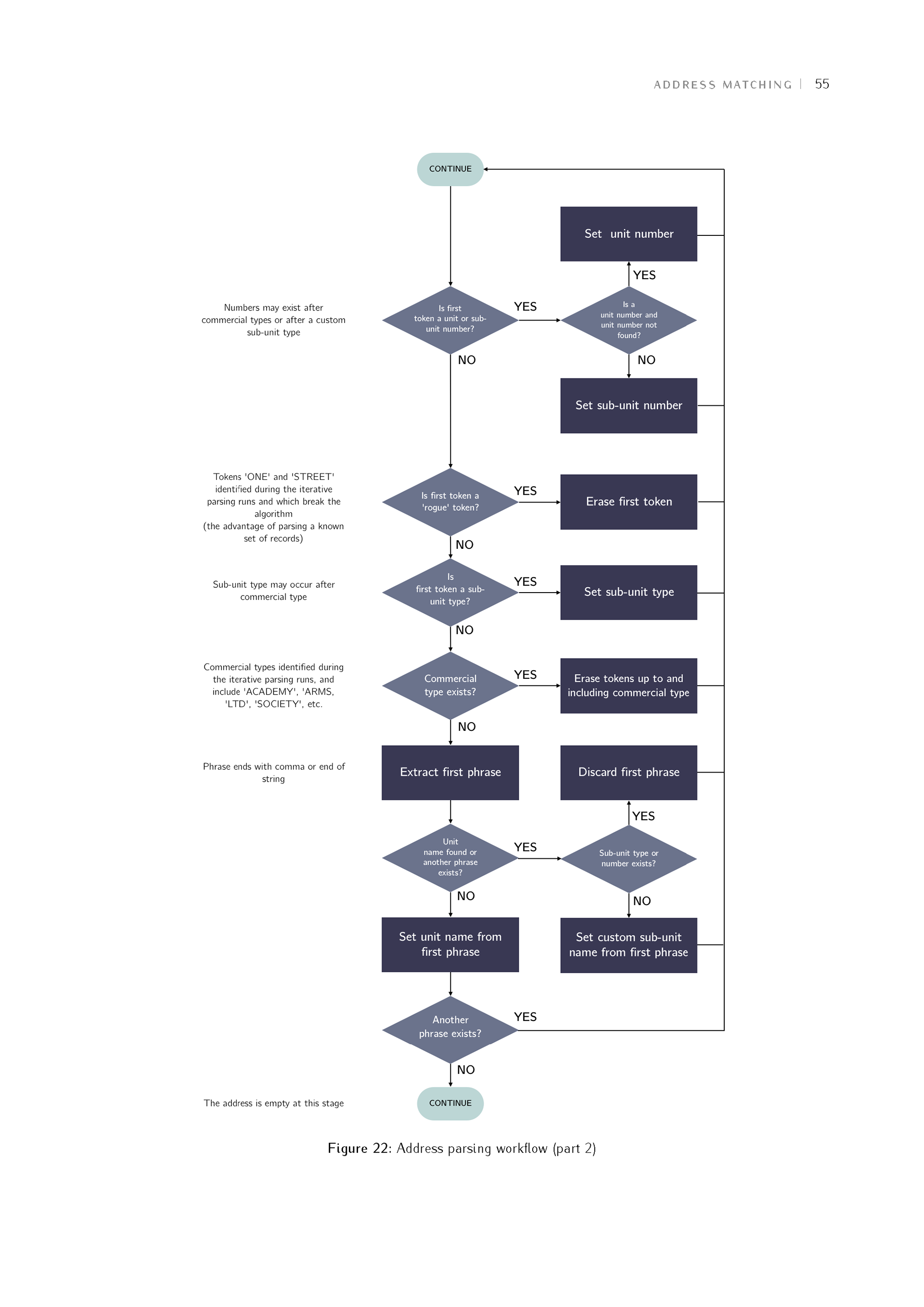
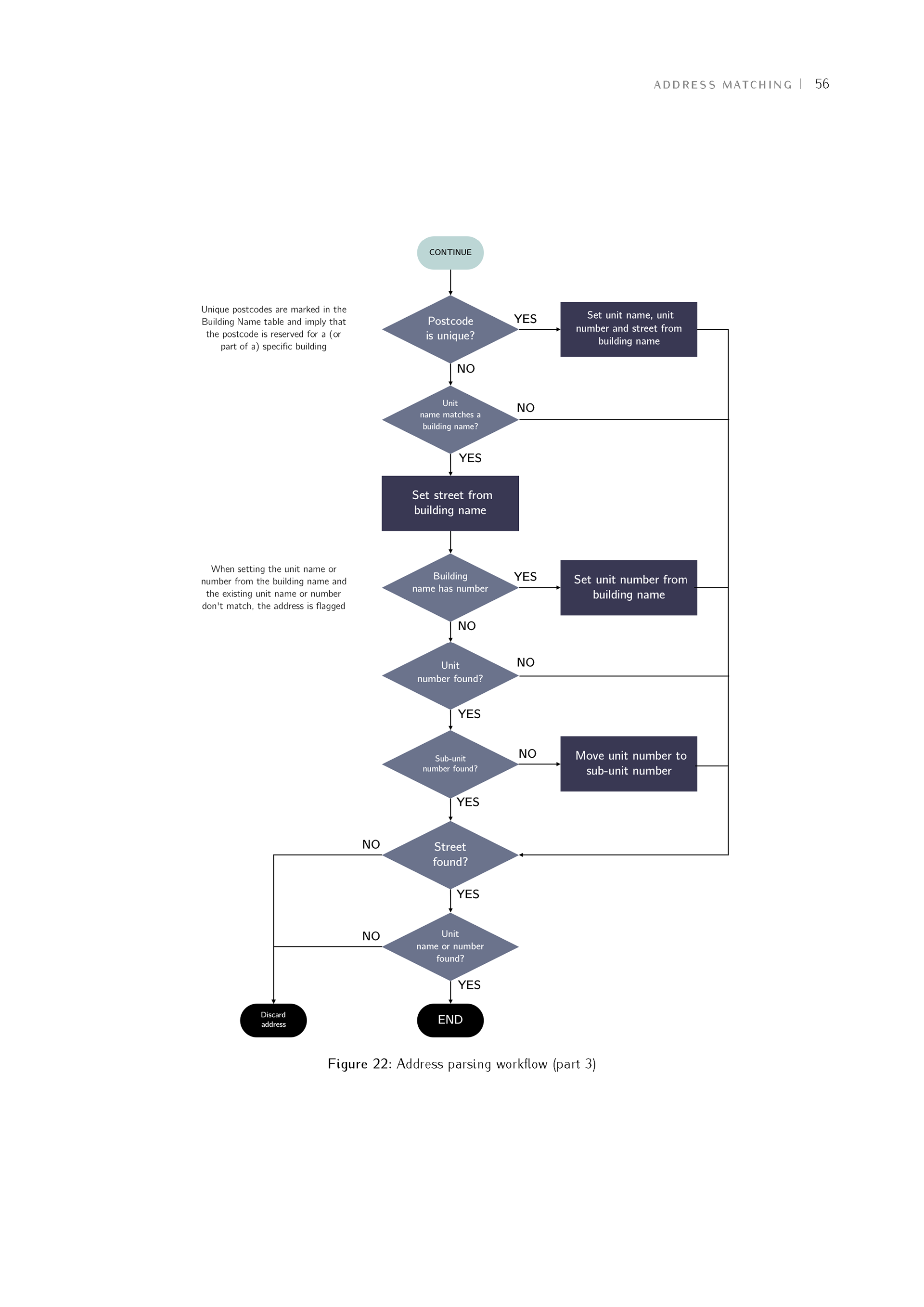
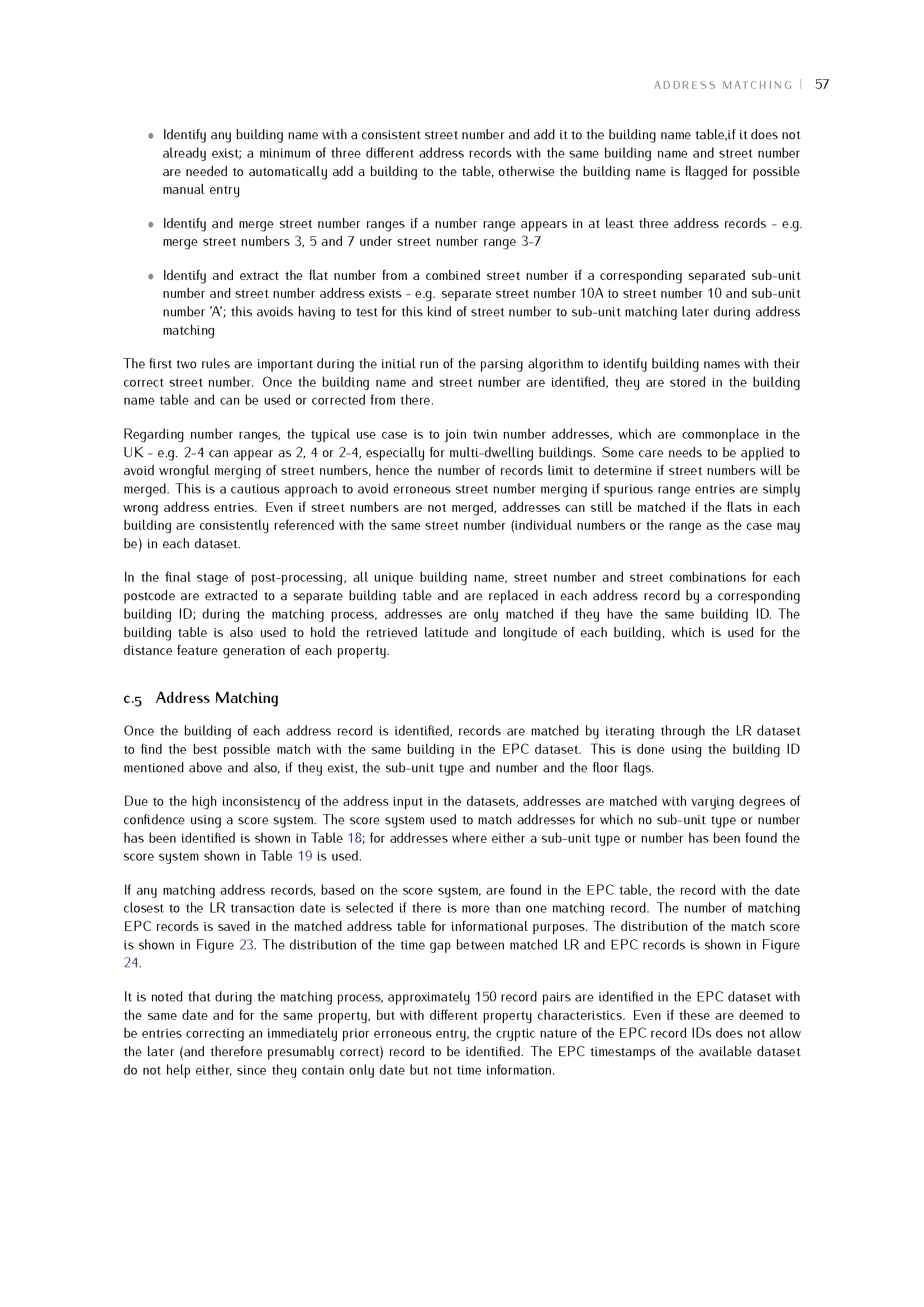
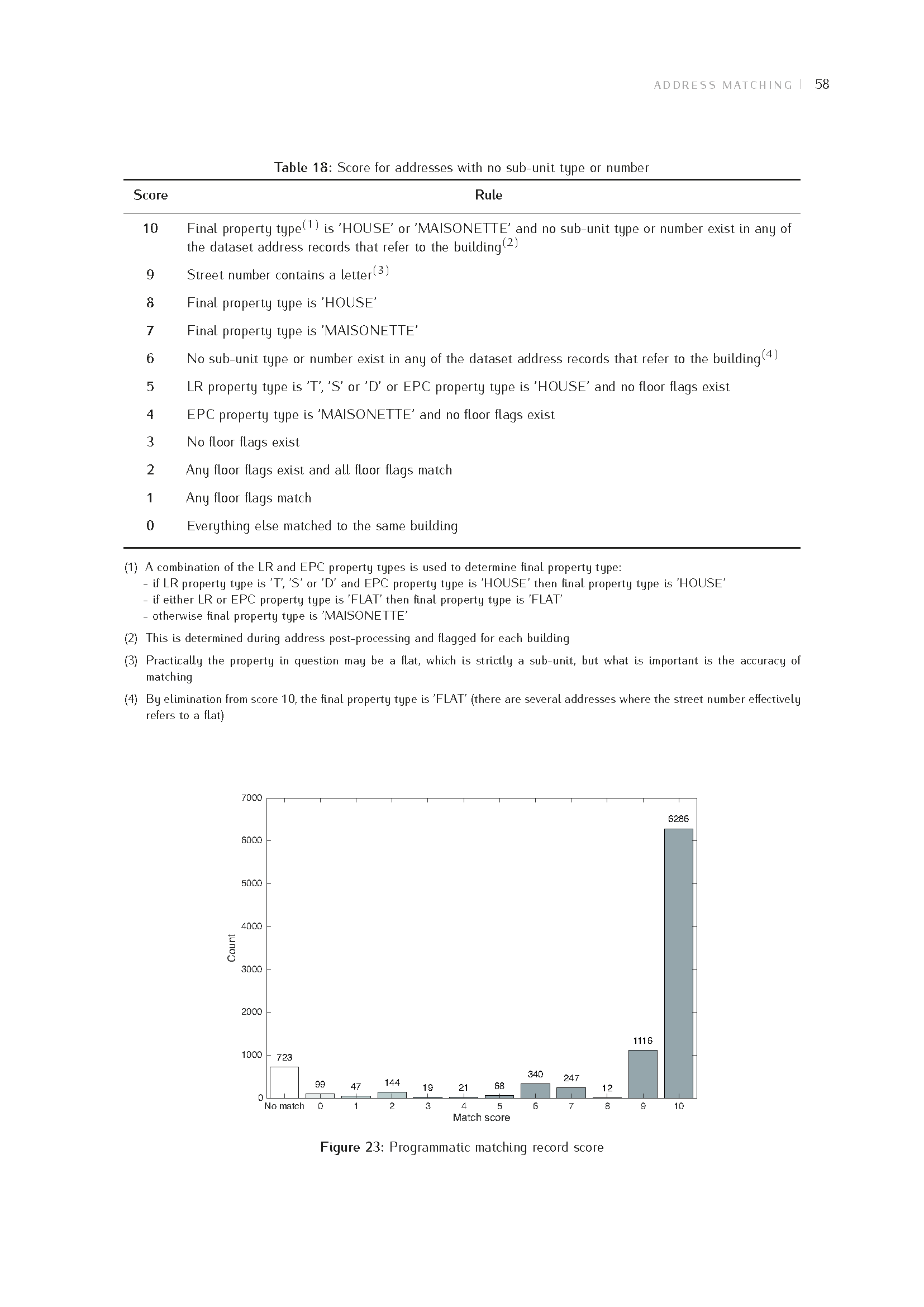
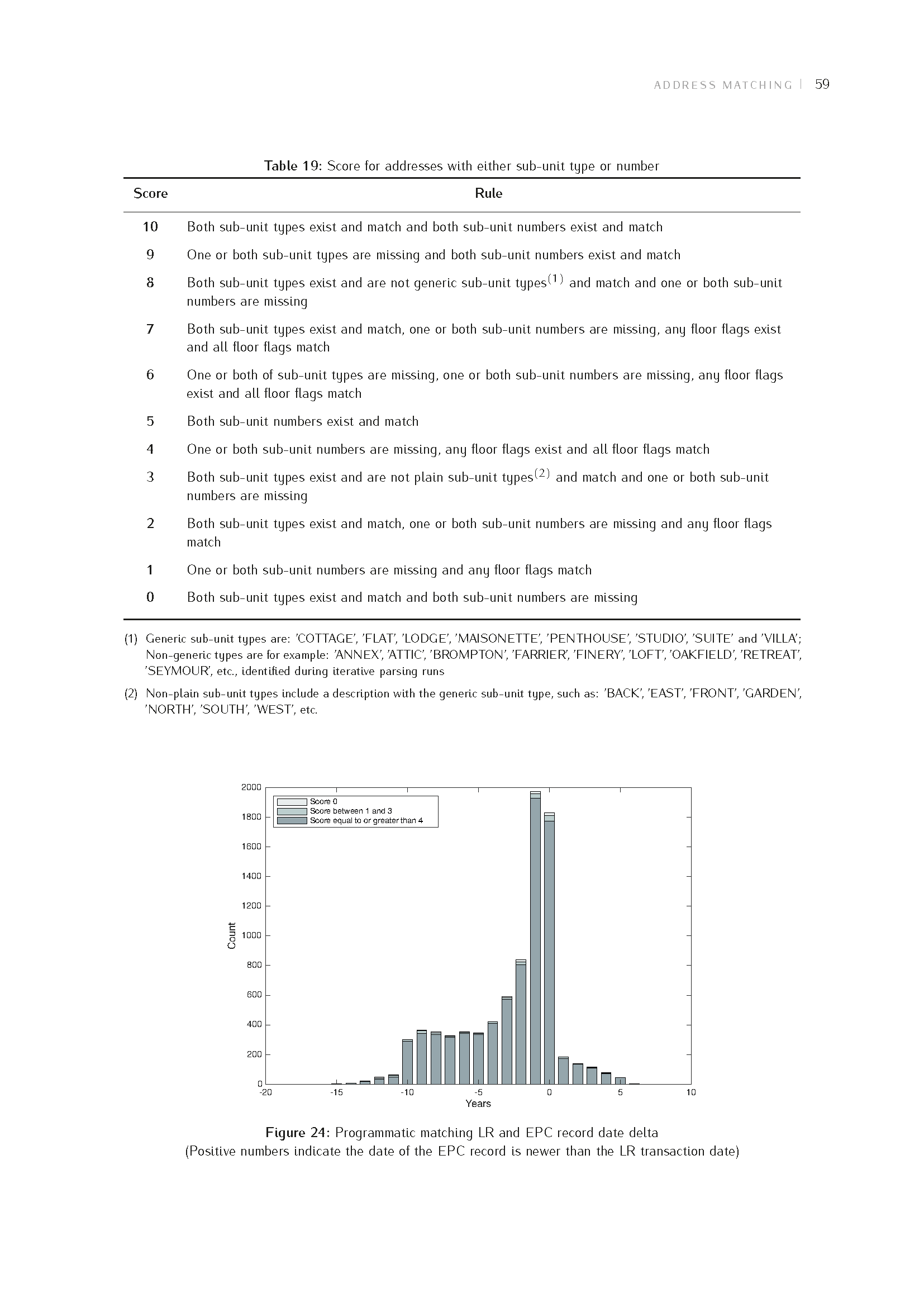
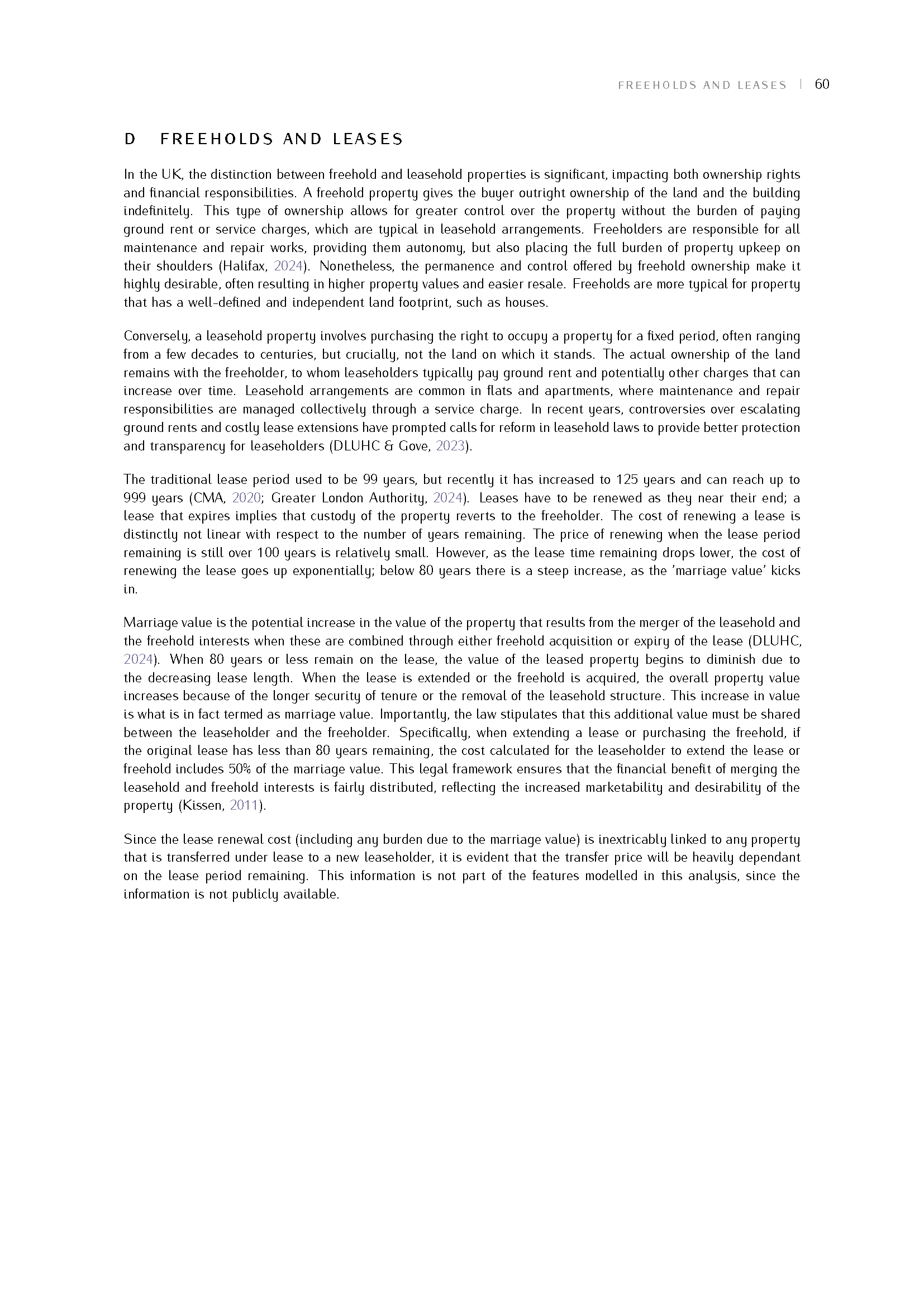
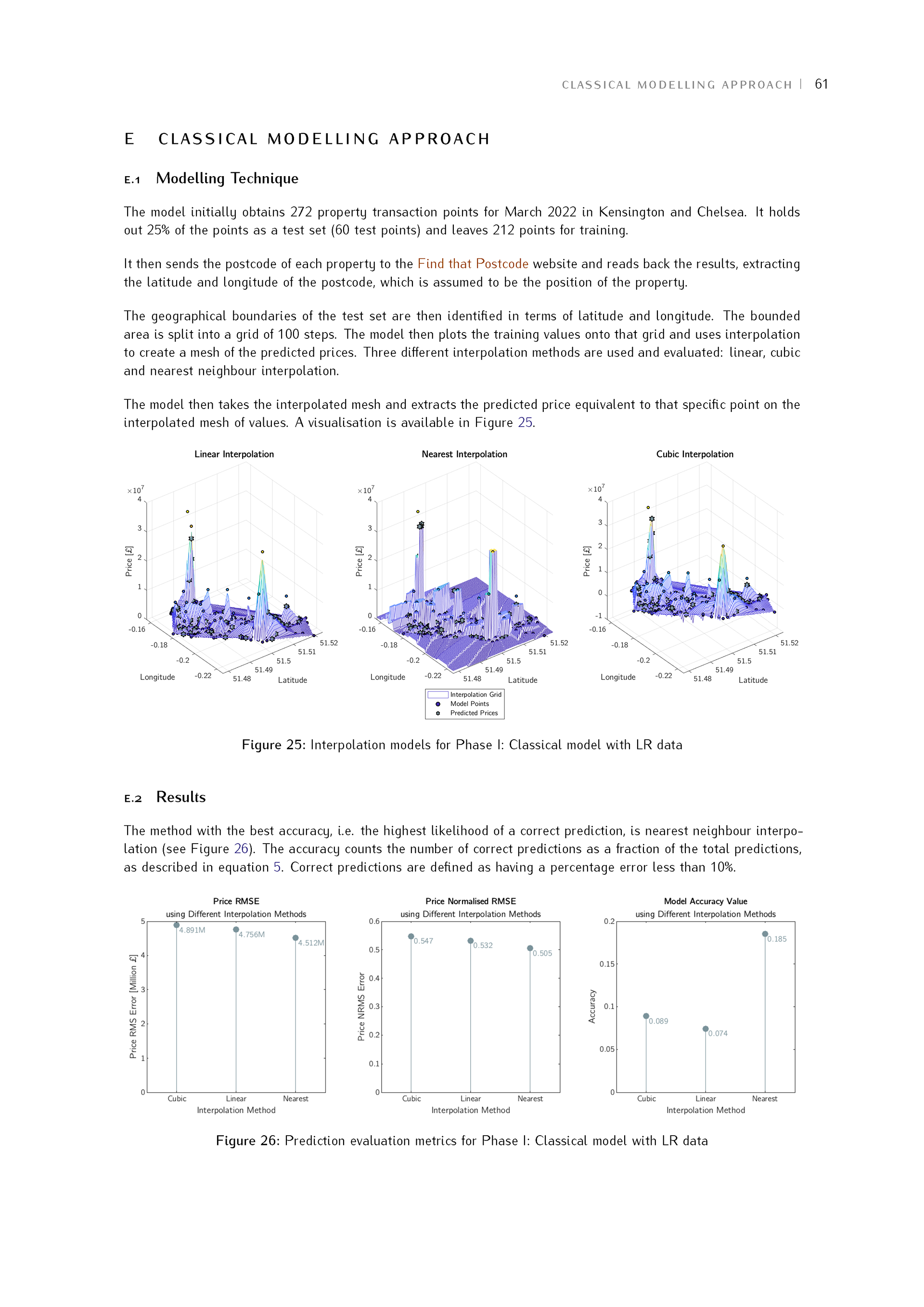
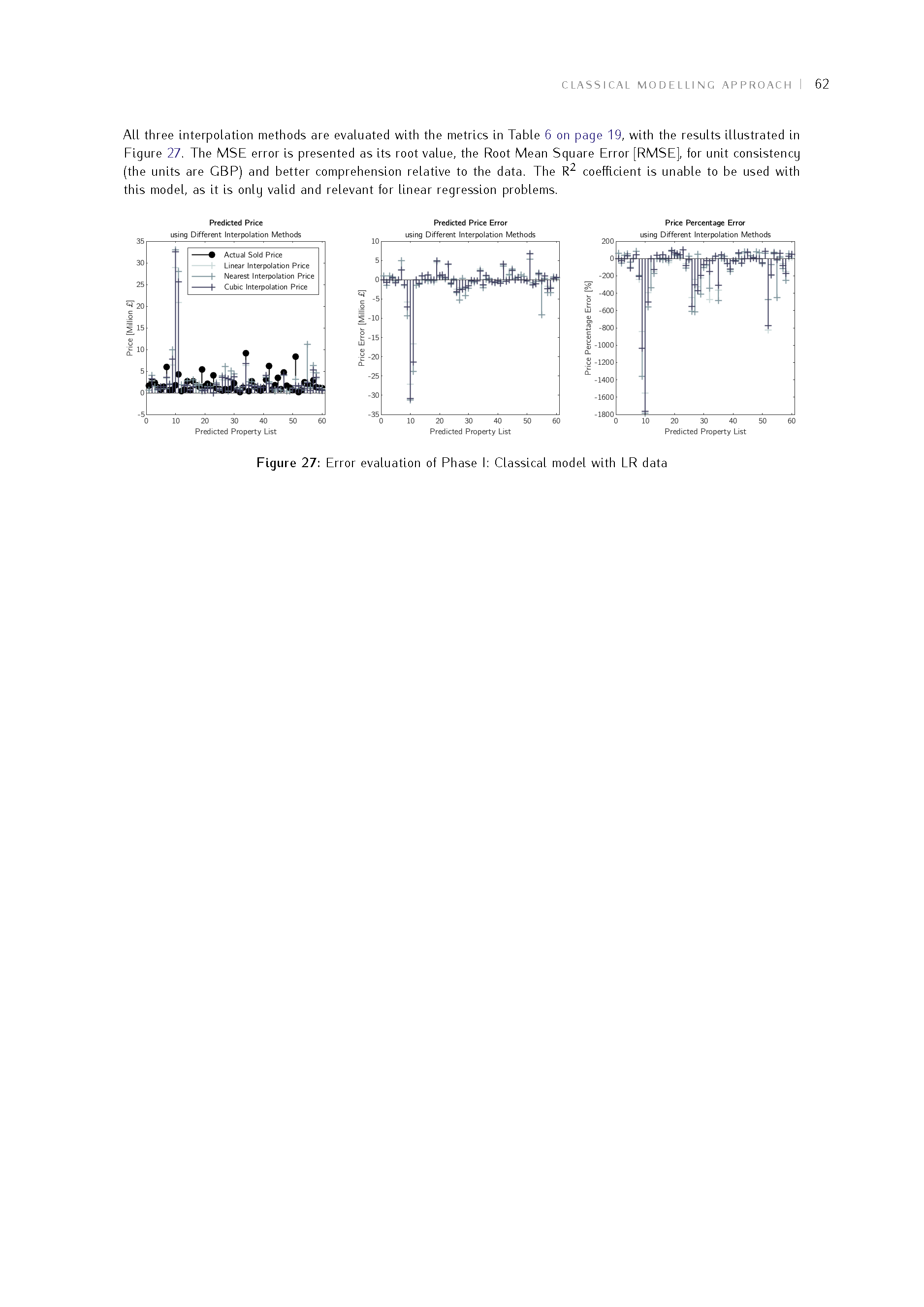
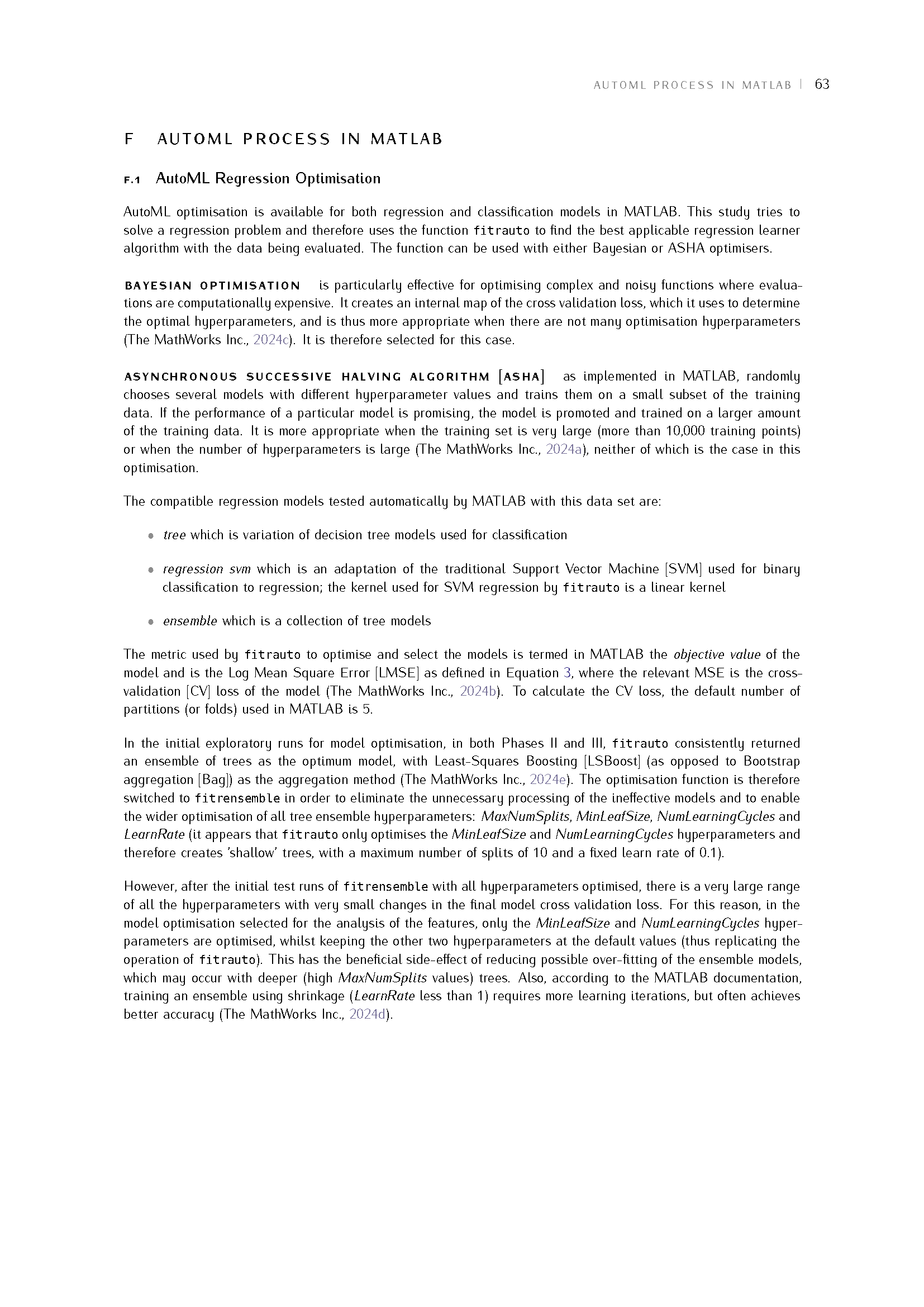
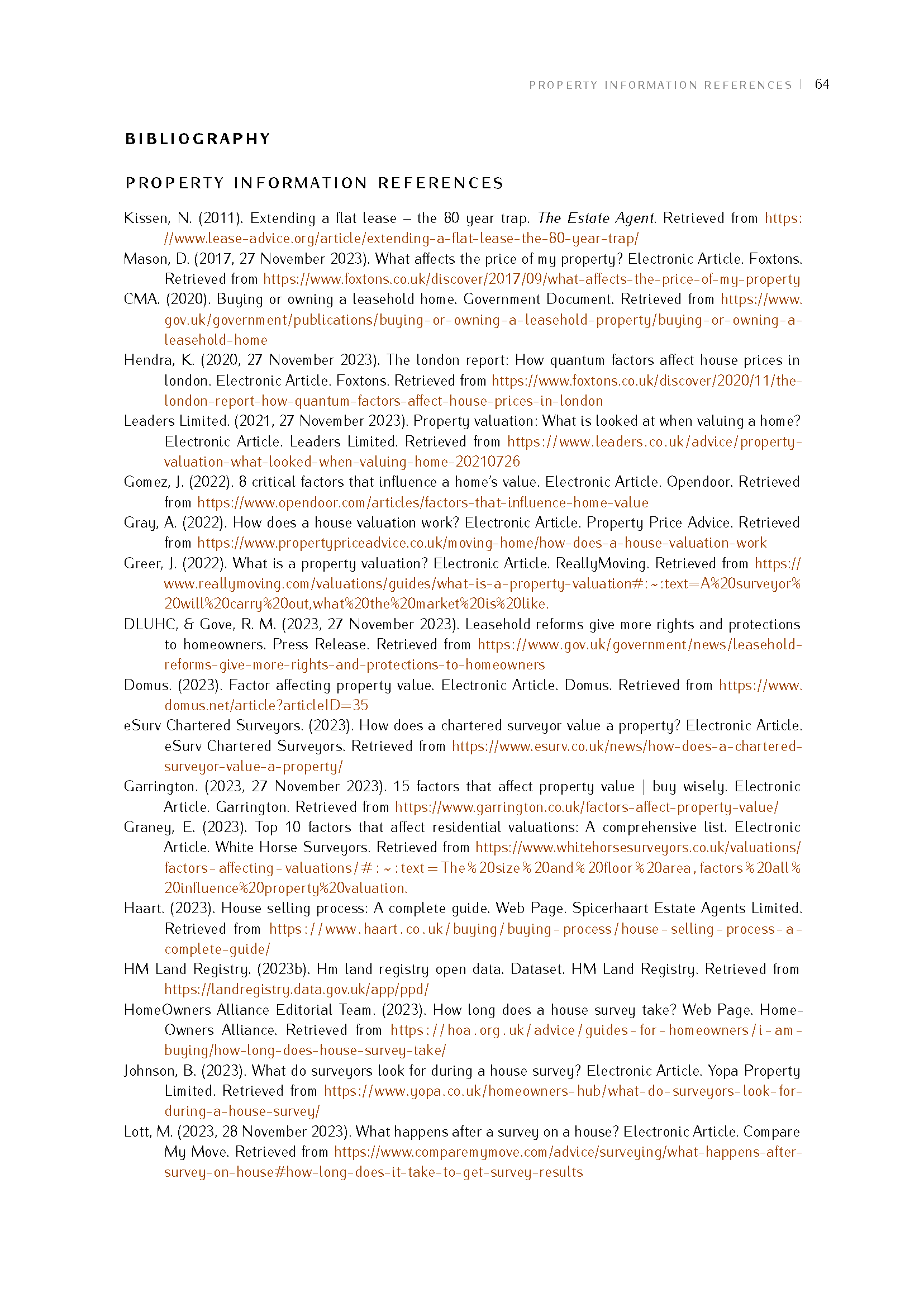
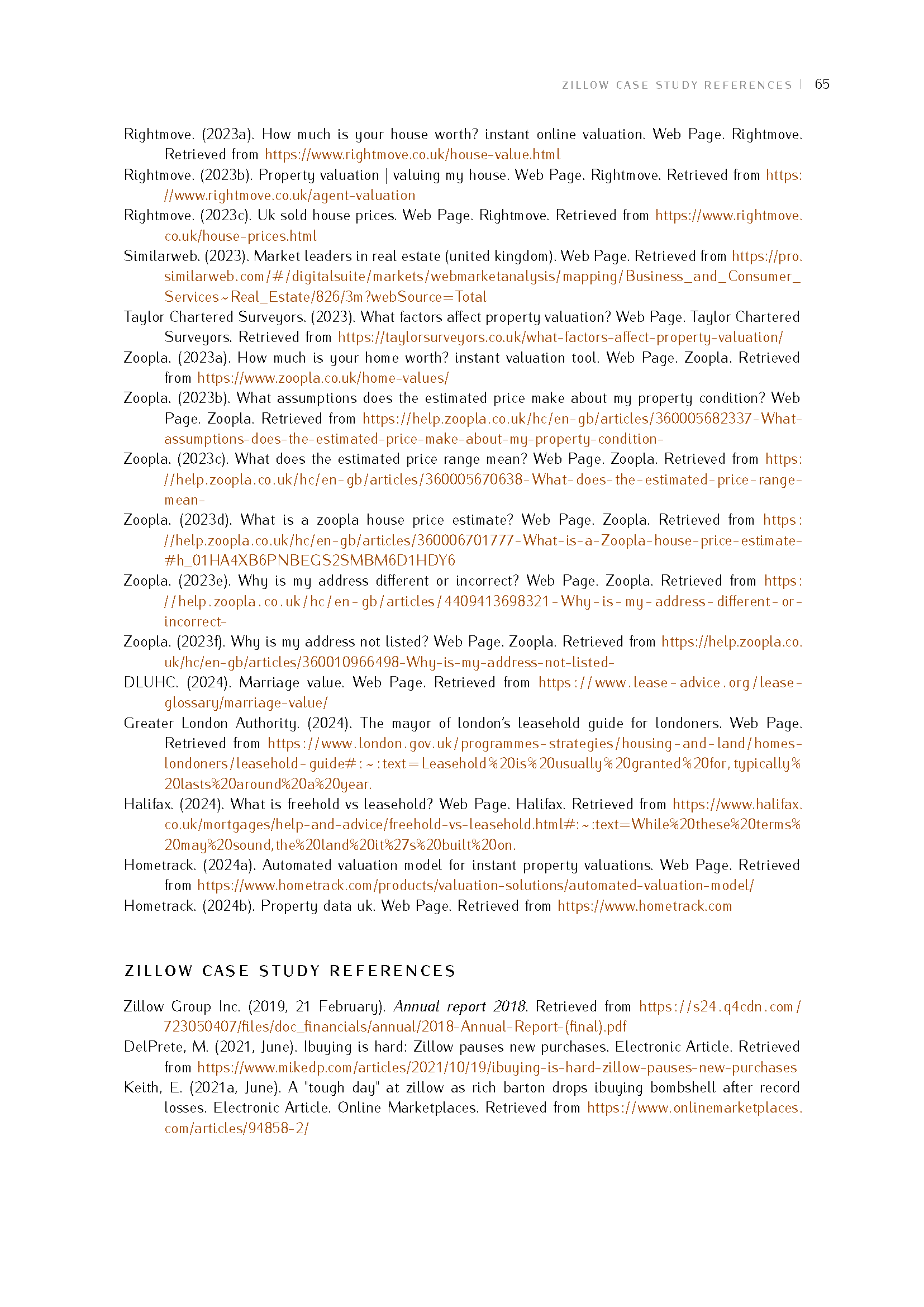
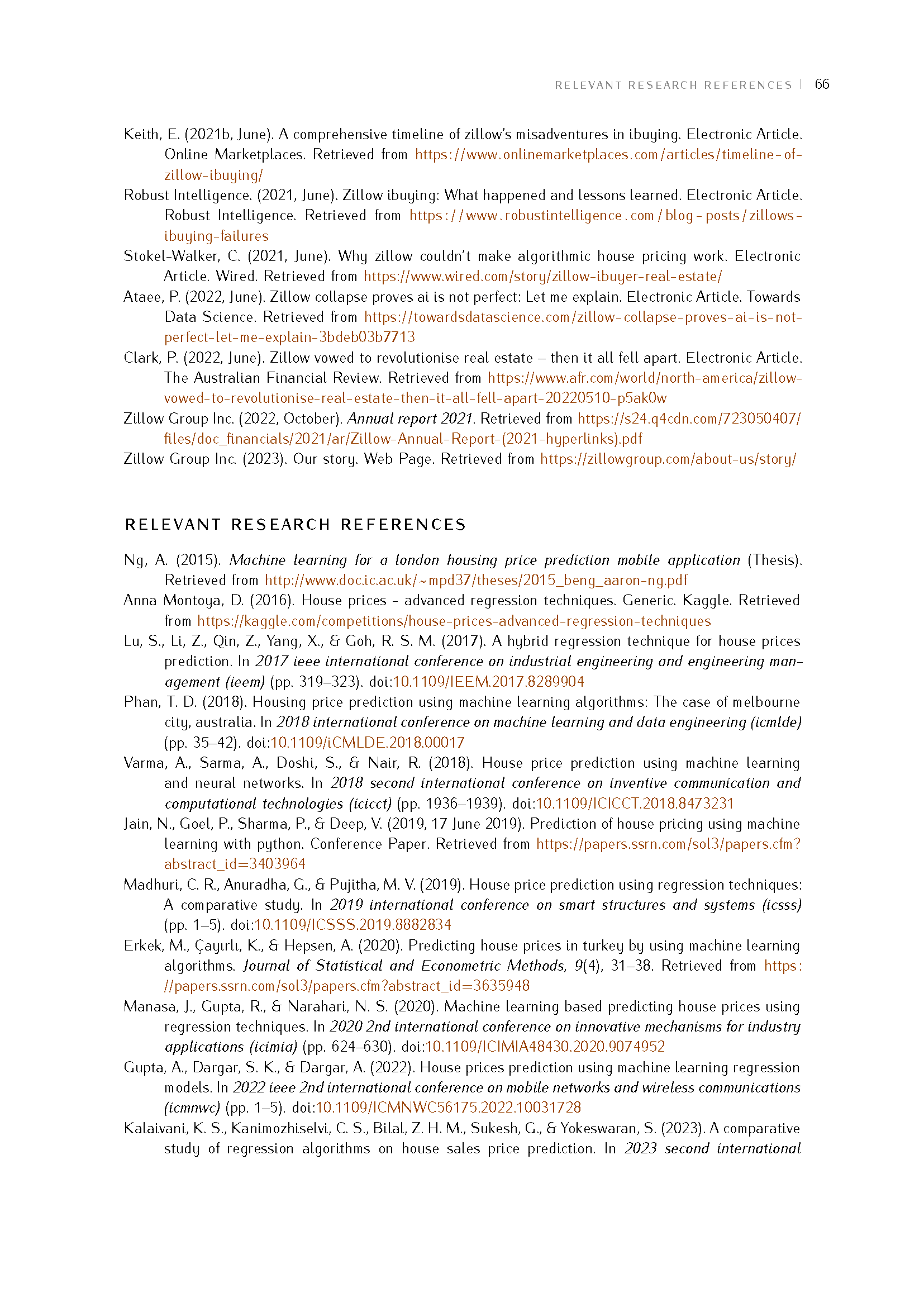
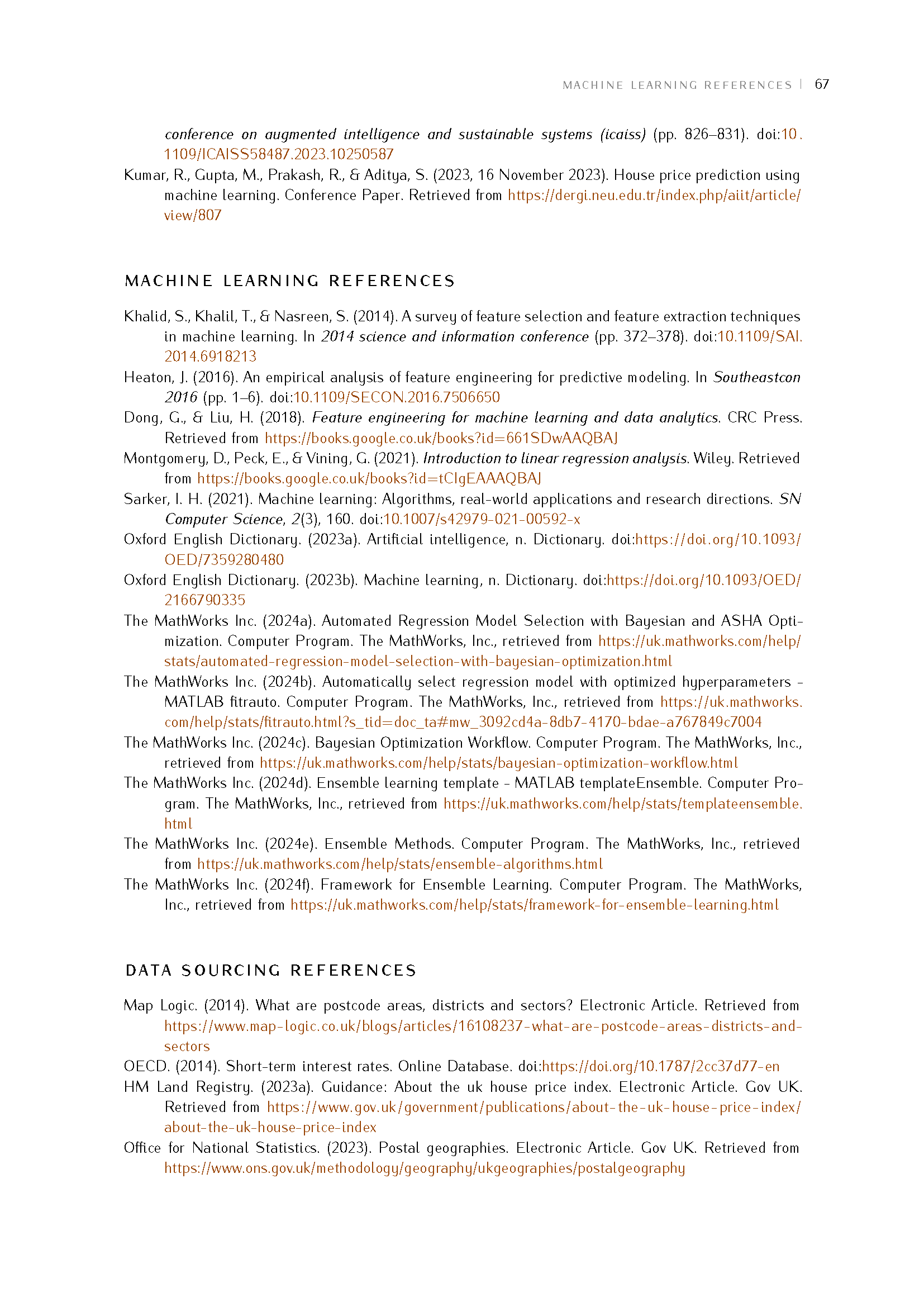
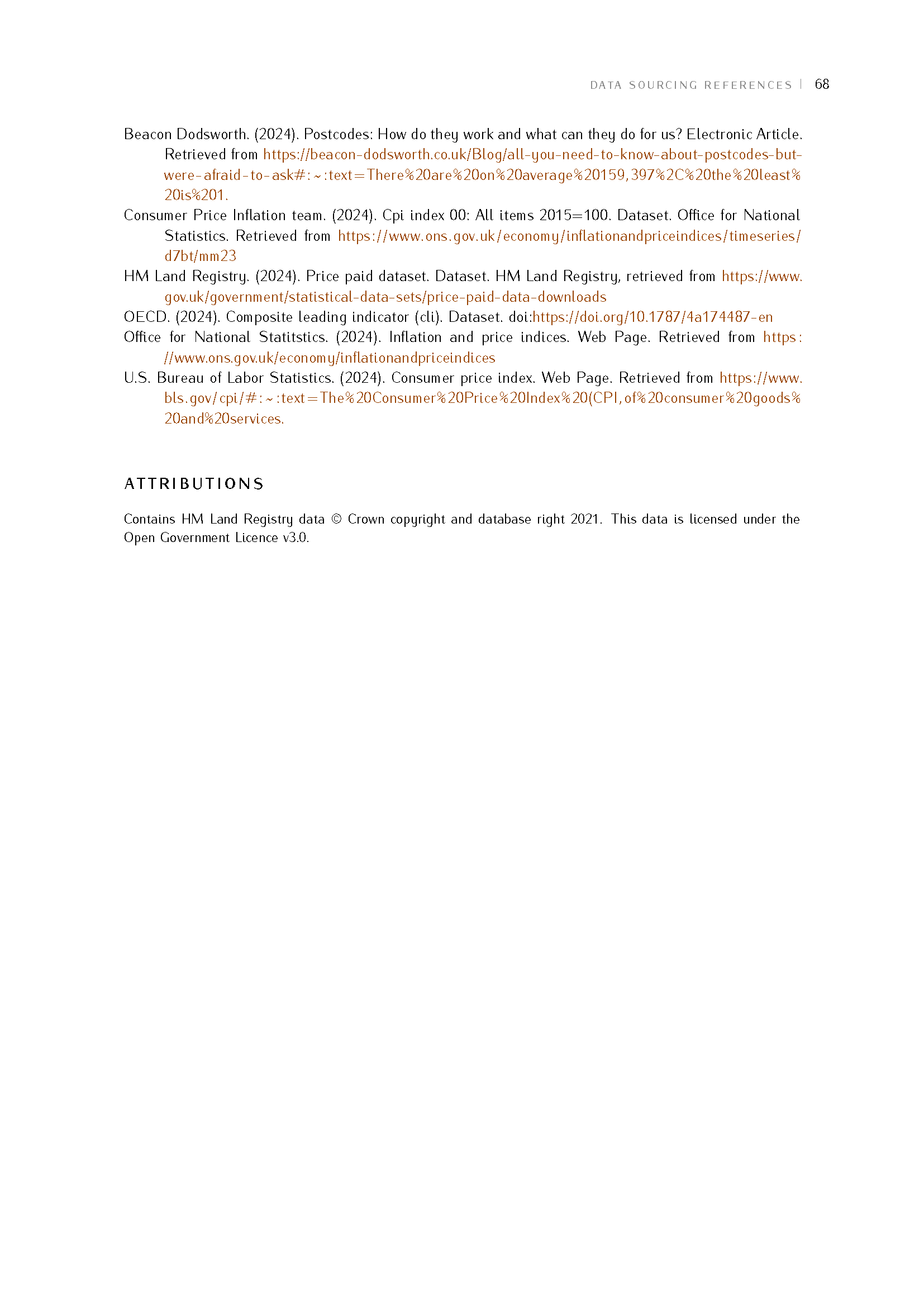